Advancements in AI-Based Natural Language Processing
Welcome to the fascinating world of Artificial Intelligence (AI) and its groundbreaking advancements in Natural Language Processing (NLP). As we journey through this article, you'll discover how AI-driven technologies are revolutionizing the way machines understand and generate human language. Imagine having a conversation with your computer that feels as natural as chatting with a friend! This is no longer a distant dream but a reality that's unfolding right before our eyes.
Recent innovations in NLP have paved the way for machines to not only comprehend human language but also to respond in a manner that feels intuitive and engaging. The evolution of NLP technologies has led to significant improvements in user experiences across various applications, from chatbots to advanced data analysis tools. Have you ever noticed how your virtual assistant seems to understand you better than ever? That's the magic of NLP at work!
These advancements can be attributed to several key factors:
- Deep Learning Techniques: The use of deep learning algorithms has enabled better understanding of context and semantics in language.
- Large Datasets: Access to vast amounts of data allows NLP models to learn and adapt, improving their accuracy over time.
- Transfer Learning: This technique allows models trained on one task to be fine-tuned for another, making them more versatile and efficient.
Furthermore, the integration of contextual embeddings has transformed NLP capabilities, allowing for a deeper understanding of language nuances. This means that machines can now grasp the subtleties of human conversation, such as sarcasm, idioms, and cultural references. The result? More engaging and meaningful interactions that enhance user satisfaction.
As we delve deeper into the applications of NLP, you'll see how it's making waves across various industries. From healthcare to finance, the impact of NLP is profound, making processes more efficient and tailored to individual needs. But with great power comes great responsibility, and the challenges that accompany these advancements cannot be overlooked. Let's explore the exciting yet complex landscape of AI-based NLP together!
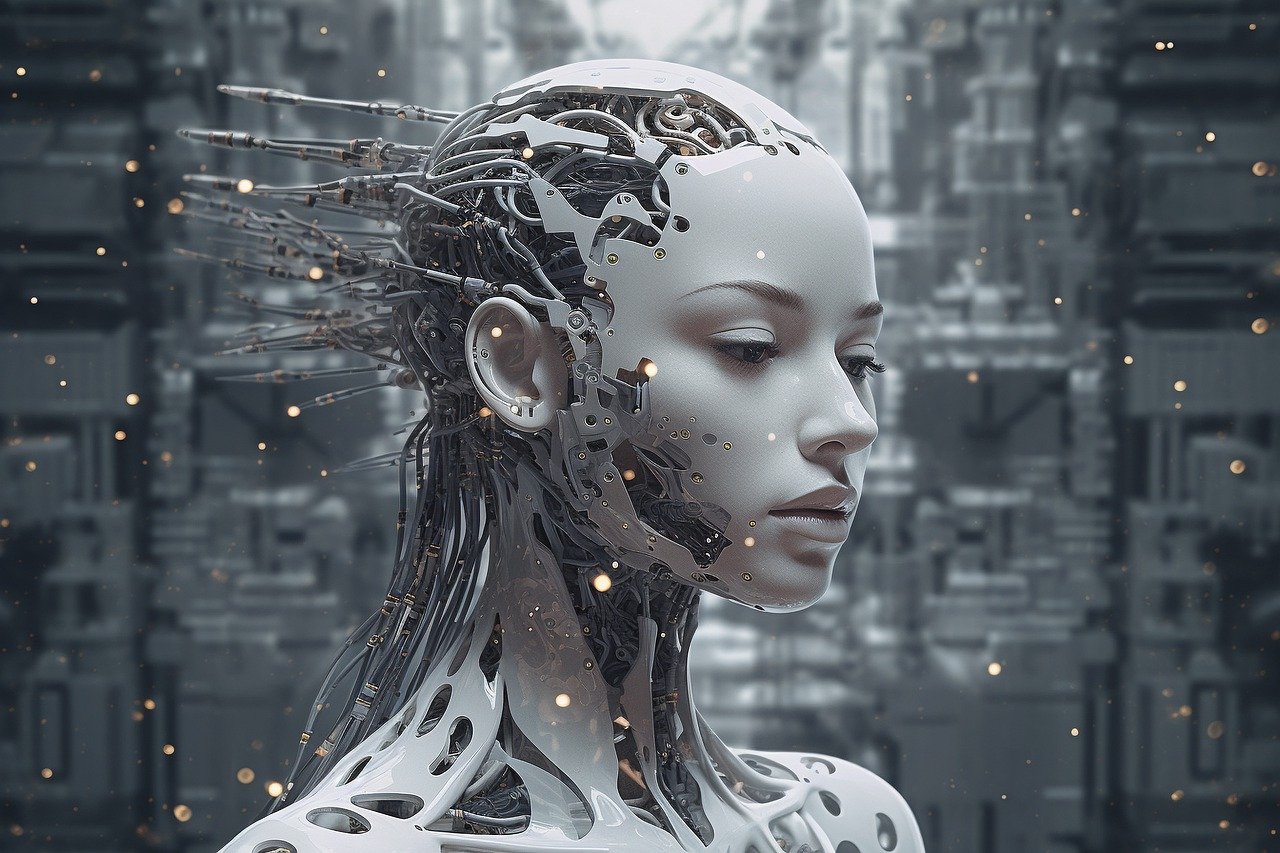
Recent Innovations in NLP
Recent breakthroughs in NLP have dramatically transformed the landscape of human-computer interaction. Imagine talking to your computer as if it were a friend, and it understands you perfectly—that's the magic of today's NLP technologies! With advancements in machine learning and deep learning, systems are now capable of not only understanding but also generating human-like text, making the interaction feel more natural and intuitive.
One of the most exciting innovations is the development of transformer models, like BERT and GPT-3. These models have revolutionized how machines process language by allowing them to consider context in a way that was previously unimaginable. For instance, they can discern the difference between the various meanings of the word "bank" depending on whether it's used in a financial or geographical context. This ability to grasp nuances in language has paved the way for more accurate sentiment analysis, enabling businesses to gauge public opinion on their products and services effectively.
Furthermore, advancements in transfer learning have made it easier to fine-tune pre-trained models for specific tasks without requiring massive datasets. This means that even smaller companies can leverage powerful NLP capabilities without incurring exorbitant costs. Imagine a small startup being able to implement sophisticated chatbots or content generation tools that were once the domain of tech giants. This democratization of technology is one of the most significant impacts of recent innovations in NLP.
Another area where NLP is making waves is in the realm of multimodal learning. This involves combining text with other forms of data, such as images and audio, to create more comprehensive models. For example, a system could analyze a video, understand the spoken words, and even interpret the visual content simultaneously. This capability opens up a treasure trove of possibilities, from enhancing virtual assistants to creating more engaging educational tools.
Moreover, the integration of ethical AI practices into NLP development is gaining traction. As organizations become increasingly aware of the potential biases in AI systems, there is a push towards developing models that are not only effective but also fair and inclusive. This involves using diverse datasets and implementing algorithms that actively mitigate bias, ensuring that NLP technologies serve a broad spectrum of users.
In summary, recent innovations in NLP are not just enhancing the way we interact with technology but are also reshaping industries by making processes more efficient and user-friendly. As we continue to explore the possibilities of NLP, we can expect even more groundbreaking advancements that will further blur the lines between human and machine communication.

Applications of NLP in Various Industries
Natural Language Processing (NLP) is not just a buzzword; it's a game-changer that is reshaping how industries operate. From healthcare to finance, the applications of NLP are vast and varied, making processes more efficient and user-friendly. Imagine a world where machines can understand your words as clearly as your best friend does—this is the reality that NLP is bringing to life.
In the healthcare sector, for example, NLP is revolutionizing how patient data is analyzed and utilized. Hospitals are inundated with unstructured data—think clinical notes, research papers, and patient feedback. NLP algorithms sift through this mountain of information, extracting valuable insights that improve patient care and operational efficiency. For instance, by analyzing patient interactions and historical data, NLP tools can identify patterns that lead to better diagnoses and treatment plans.
Moreover, the integration of AI-driven chatbots and virtual assistants in healthcare settings is enhancing patient interactions. These tools provide immediate responses to patient inquiries, offering personalized health information that increases engagement and satisfaction. Imagine waiting for a doctor's appointment and having a chatbot provide you with relevant health tips or reminders about your medications—this is the kind of proactive care that NLP facilitates.
Moving over to the financial sector, NLP is making waves by enabling organizations to conduct sentiment analysis, fraud detection, and risk management. By analyzing customer feedback and market trends, financial institutions can gauge public sentiment and make informed decisions. For instance, during a financial crisis, NLP can analyze social media and news reports to understand public sentiment towards a particular stock, allowing traders to make timely decisions.
Furthermore, NLP plays a crucial role in ensuring compliance and enhancing security. By automating the analysis of large volumes of transaction data, NLP helps in identifying suspicious activities that may indicate fraud. This not only saves time but also adds an additional layer of security for financial organizations.
In the education sector, NLP is transforming personalized learning experiences. By analyzing students' interactions and performance data, educational platforms can tailor content that meets individual learning needs. This can be likened to having a personal tutor who understands your strengths and weaknesses, providing resources that are just right for you.
In summary, the applications of NLP across various industries are not just enhancing efficiency; they are also creating more personalized and engaging experiences for users. As we continue to explore the capabilities of NLP, the future looks bright. With ongoing advancements, we can expect even more innovative uses that will further transform how we interact with technology and each other.
- What is Natural Language Processing (NLP)? NLP is a branch of artificial intelligence that focuses on the interaction between computers and humans through natural language.
- How is NLP used in healthcare? NLP is used in healthcare for analyzing unstructured data, improving patient care, and enhancing administrative efficiency.
- Can NLP help in fraud detection? Yes, NLP can analyze transaction data and identify patterns that may indicate fraudulent activities.
- What industries benefit from NLP? Industries such as healthcare, finance, education, and customer service are significantly benefiting from NLP technologies.
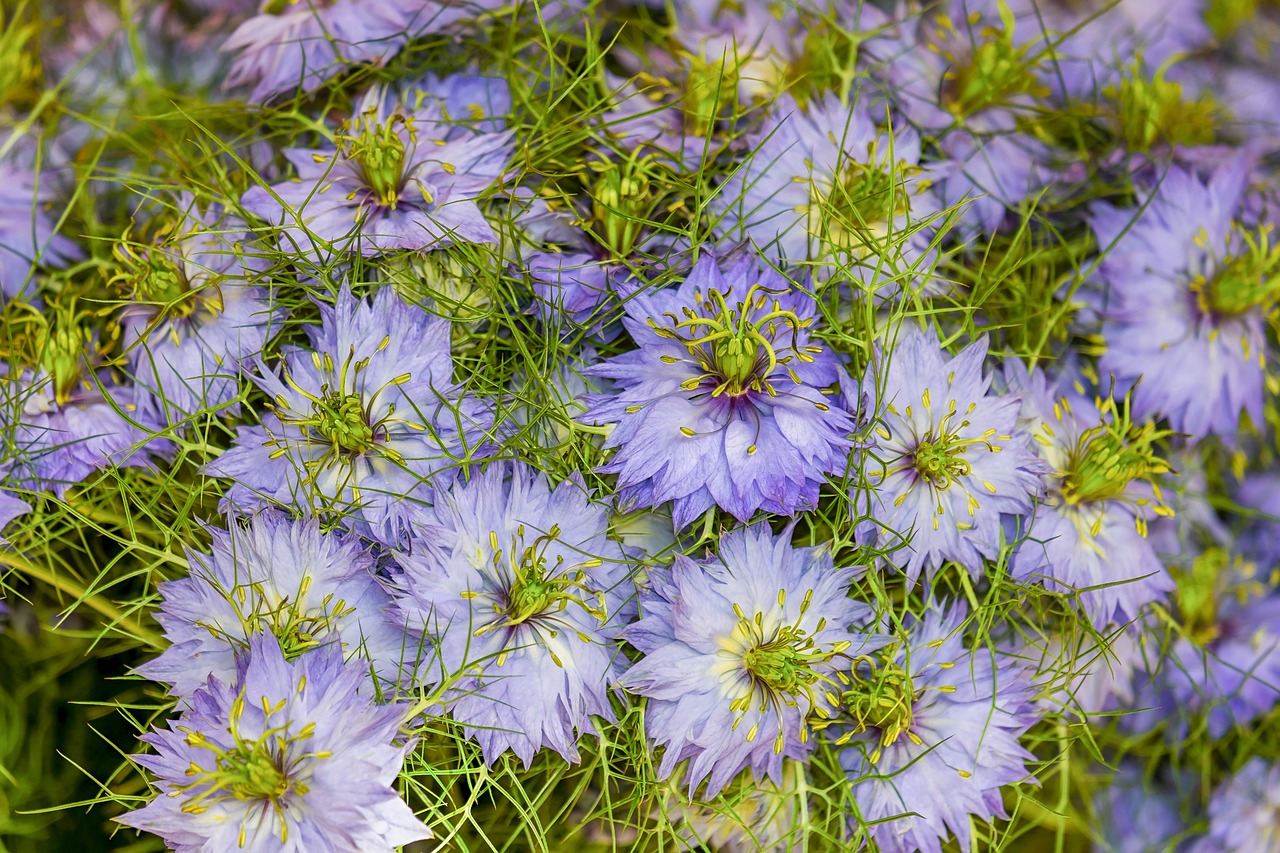
Healthcare Applications
In today's fast-paced world, the healthcare industry is undergoing a significant transformation, largely driven by advancements in Artificial Intelligence (AI) and Natural Language Processing (NLP). These technologies are not just buzzwords; they are reshaping how healthcare providers interact with patients and manage critical data. Imagine a world where doctors can quickly access vital patient information, and patients can receive answers to their health queries instantly—this is the reality that NLP is helping to create.
One of the most exciting applications of NLP in healthcare is its ability to extract insights from unstructured data, such as clinical notes, patient records, and research papers. This capability is crucial because a significant portion of healthcare data is unstructured, making it challenging to analyze using traditional methods. By employing sophisticated NLP algorithms, healthcare professionals can sift through mountains of data to find relevant information, leading to better diagnosis and treatment options.
Moreover, NLP is streamlining administrative tasks, allowing healthcare providers to focus more on patient care rather than paperwork. For instance, NLP can automate the transcription of doctor-patient interactions, ensuring accurate records without the need for manual entry. This not only saves time but also reduces the likelihood of human error, ultimately enhancing operational efficiency.
AI-driven chatbots and virtual assistants are revolutionizing patient interactions. These tools provide timely responses and personalized health information, leading to improved patient engagement and satisfaction. Patients can ask questions about their symptoms or treatment options and receive instant, accurate replies. This level of accessibility empowers patients, making them feel more involved in their healthcare journey.
NLP algorithms are particularly valuable in clinical data analysis. They facilitate the extraction of critical information from clinical notes and research papers, which can inform treatment decisions and medical research. For example, by analyzing thousands of clinical notes, NLP can identify patterns and correlations that might not be immediately apparent to human analysts. This capability can lead to breakthroughs in understanding diseases and improving patient outcomes.
To summarize, the integration of NLP in healthcare is not just about technology; it's about enhancing the quality of care that patients receive. By improving patient interactions, streamlining administrative tasks, and enabling more effective clinical data analysis, NLP is paving the way for a more efficient and patient-centered healthcare system.
- What is Natural Language Processing?
NLP is a branch of AI that focuses on the interaction between computers and humans through natural language. It enables machines to understand, interpret, and generate human language. - How does NLP improve patient care?
NLP enhances patient care by automating responses through chatbots, analyzing clinical data for better diagnosis, and streamlining administrative tasks to allow healthcare professionals to focus more on patient interactions. - What are some challenges of using NLP in healthcare?
Challenges include language ambiguity, the need for diverse datasets, and ensuring that the algorithms are free from bias, which can affect the quality of care provided.

Patient Interaction Enhancement
In today's fast-paced world, where time is of the essence, the healthcare sector is witnessing a remarkable transformation, thanks to the advent of AI-driven chatbots and virtual assistants. These technologies are not just a passing trend; they are revolutionizing the way patients interact with healthcare providers. Imagine being able to get answers to your health queries at any hour of the day without having to wait on hold or schedule an appointment. This is the new reality that AI is creating.
By leveraging natural language processing (NLP), these virtual assistants can understand and respond to patient inquiries in a conversational manner. This not only enhances patient engagement but also significantly improves overall satisfaction. Patients can receive personalized health information tailored to their specific needs, making them feel valued and understood. For instance, if a patient asks about their medication side effects, a chatbot can provide immediate, accurate information, helping them make informed decisions without unnecessary delays.
Moreover, the implementation of AI in patient interactions leads to a more efficient healthcare system. By automating routine inquiries and administrative tasks, healthcare professionals can focus on what they do best—providing care. The reduction in administrative burdens allows for more time spent on patient-centered activities, ultimately leading to better health outcomes. Just consider the time saved when a chatbot can handle appointment scheduling or follow-up reminders, allowing staff to dedicate their efforts to more complex patient needs.
However, it's important to recognize that while these advancements are impressive, they come with their own set of challenges. For instance, ensuring that the AI systems are equipped to handle sensitive patient information securely is paramount. Additionally, the technology must be constantly updated to reflect the latest medical guidelines and practices. This is where ongoing development and rigorous testing are crucial to maintaining trust and reliability in these AI systems.
In conclusion, the enhancement of patient interactions through AI-driven solutions is a game changer for the healthcare industry. It not only fosters improved communication but also streamlines operations, leading to a more efficient and responsive healthcare environment. As we move forward, the integration of these technologies will likely continue to evolve, paving the way for a future where healthcare is more accessible and personalized than ever before.
- What is AI-driven patient interaction? AI-driven patient interaction refers to the use of artificial intelligence technologies, such as chatbots and virtual assistants, to facilitate communication between patients and healthcare providers.
- How do chatbots improve patient engagement? Chatbots improve patient engagement by providing immediate responses to inquiries, offering personalized health information, and reducing wait times for assistance.
- What challenges do AI systems face in healthcare? AI systems in healthcare face challenges such as ensuring data security, maintaining up-to-date medical information, and managing sensitive patient interactions appropriately.
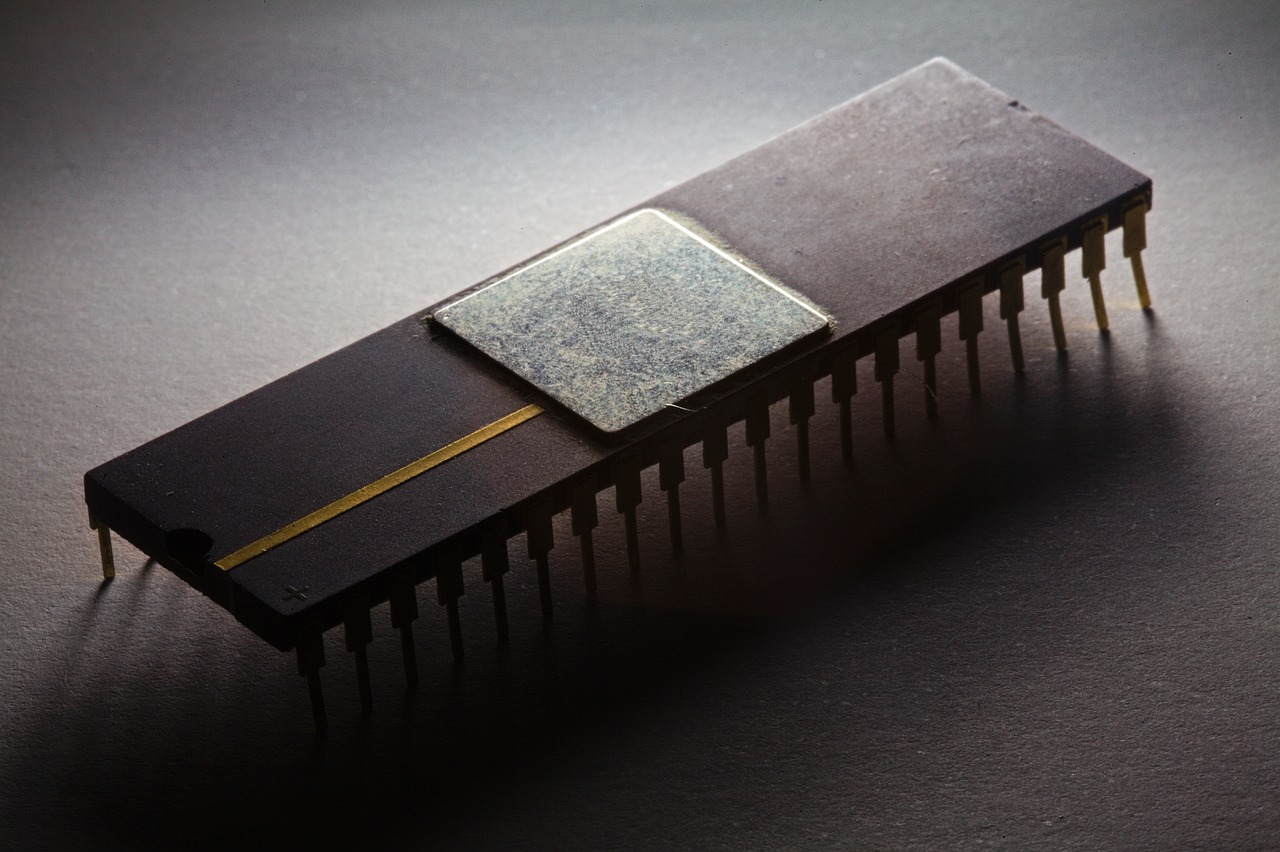
Clinical Data Analysis
In the realm of healthcare, has become a game changer, thanks to the power of Natural Language Processing (NLP). By leveraging advanced algorithms, NLP tools can sift through mountains of unstructured data, such as clinical notes, research papers, and patient records, to extract valuable insights. This capability not only streamlines the workflow for healthcare professionals but also enhances the quality of patient care. Imagine a physician being able to quickly access relevant patient history, treatment outcomes, and even the latest research findings—all at their fingertips. This is the future of healthcare, and it's powered by NLP.
The process of clinical data analysis involves several key steps:
- Data Extraction: NLP algorithms can identify and extract pertinent information from various sources, including electronic health records (EHRs) and clinical trial reports.
- Information Synthesis: Once the data is extracted, NLP systems can synthesize this information, allowing healthcare providers to see trends, correlations, and anomalies that may not be immediately apparent.
- Decision Support: By analyzing historical data, NLP can assist in clinical decision-making, offering recommendations based on similar past cases and current best practices.
For instance, consider a scenario where a doctor needs to make a diagnosis based on a patient's symptoms. Instead of manually reviewing hundreds of pages of clinical notes, an NLP system can quickly analyze this data and highlight relevant cases, treatment outcomes, and even suggest possible diagnoses. This not only saves time but also reduces the risk of human error, ultimately leading to better patient outcomes.
Furthermore, the integration of NLP in clinical data analysis supports research initiatives. Researchers can utilize NLP to conduct literature reviews, summarize findings, and even identify gaps in existing research. This accelerates the pace of medical research and fosters innovation in treatment methodologies. As a result, the healthcare industry is not only improving its operational efficiency but is also paving the way for groundbreaking discoveries.
In summary, the impact of NLP on clinical data analysis is profound. By transforming how healthcare professionals access and utilize information, NLP is enhancing patient care, streamlining administrative tasks, and driving forward medical research. The potential is immense, and as these technologies continue to evolve, we can expect even greater advancements that will benefit both healthcare providers and patients alike.
- What is Natural Language Processing (NLP)? NLP is a field of artificial intelligence that focuses on the interaction between computers and humans through natural language.
- How is NLP used in healthcare? NLP is used in healthcare for tasks such as clinical data analysis, patient interaction enhancement, and streamlining administrative processes.
- What are the benefits of using NLP in clinical data analysis? The benefits include improved accuracy in data extraction, enhanced decision-making capabilities, and faster access to critical information.
- What challenges does NLP face in healthcare? Challenges include language ambiguity, the need for diverse datasets, and ensuring the ethical use of AI technologies.
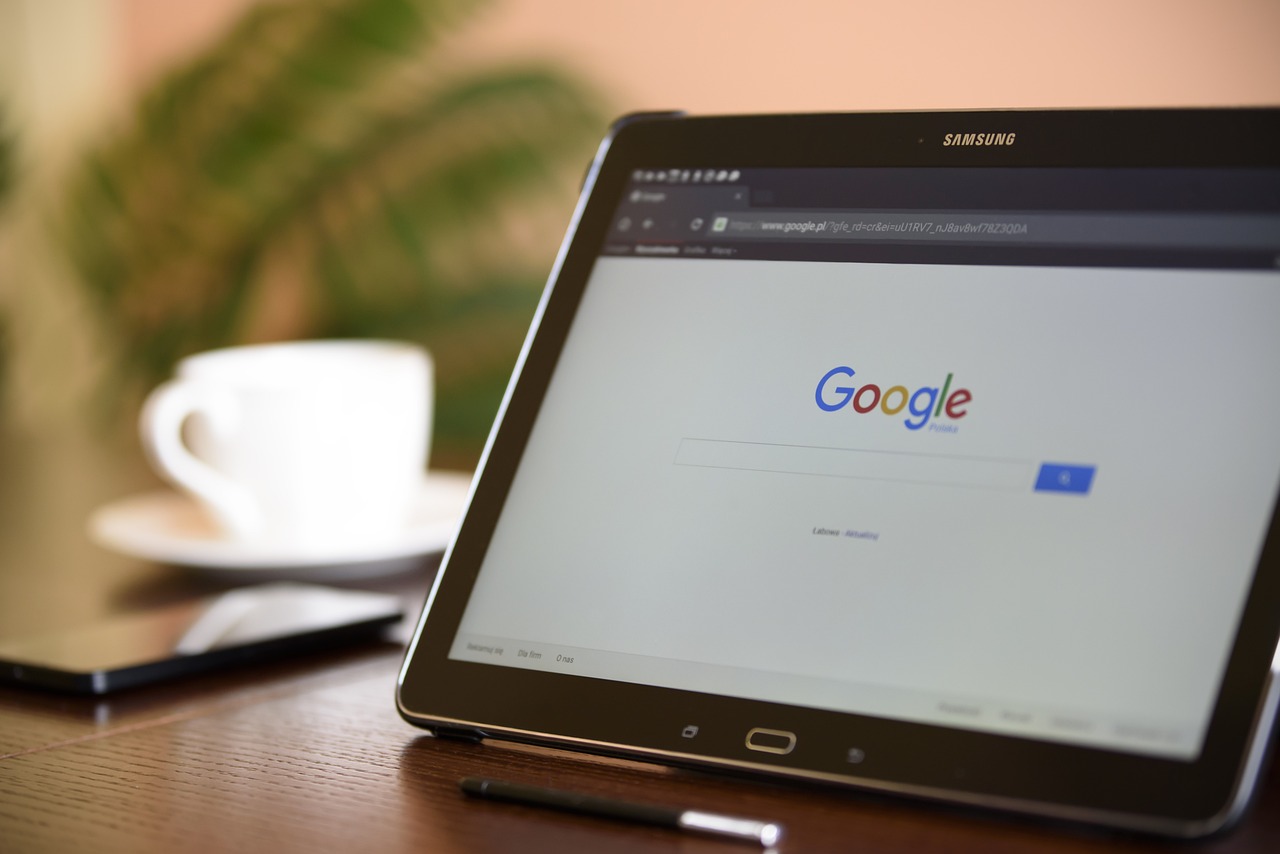
Financial Sector Innovations
The financial sector is undergoing a profound transformation thanks to the integration of Natural Language Processing (NLP) technologies. As organizations strive to harness the immense data generated daily, NLP emerges as a powerful tool for analyzing sentiments, detecting fraud, and managing risks. Imagine having a financial advisor that can process vast amounts of information in seconds, providing insights that would take humans days, if not weeks, to uncover. This is not just a dream; it's the reality that NLP is creating.
One of the most significant applications of NLP in finance is sentiment analysis. This involves analyzing social media posts, news articles, and financial reports to gauge public sentiment about a particular stock or market trend. By understanding investor emotions, financial institutions can make informed decisions, potentially leading to improved investment strategies. For instance, a sudden spike in negative sentiment about a company could prompt a swift response from investors, mitigating potential losses.
Moreover, fraud detection has been revolutionized through NLP. Traditional methods of identifying fraudulent transactions often rely on rigid rules and historical data, which can be limiting. However, NLP allows for the analysis of unstructured data, such as transaction descriptions and customer interactions. By employing advanced algorithms, financial institutions can identify patterns indicative of fraud, thus enhancing security and protecting customers. The ability to detect anomalies in real-time can be the difference between preventing a fraudulent transaction and suffering significant losses.
Risk management is another area where NLP shines. Financial organizations face myriad risks, from market volatility to regulatory compliance. NLP tools can analyze regulatory documents, news reports, and market data to provide insights into potential risks. For example, a bank can utilize NLP to monitor compliance with evolving regulations, ensuring that it remains within legal boundaries while minimizing the risk of penalties. This proactive approach not only helps in adhering to regulations but also enhances the overall stability of the financial institution.
To illustrate the impact of NLP in the financial sector, consider the following table that summarizes key innovations:
Innovation | Application | Benefits |
---|---|---|
Sentiment Analysis | Market trend evaluation | Informed investment strategies |
Fraud Detection | Transaction monitoring | Enhanced security and loss prevention |
Risk Management | Compliance monitoring | Proactive risk mitigation |
As we look to the future, the innovations in NLP within the financial sector are set to expand further. With the increasing reliance on data-driven decisions, financial institutions that embrace NLP technologies will not only gain a competitive edge but also enhance their ability to serve customers effectively. The integration of these advanced technologies will lead to more personalized financial services and a more secure banking environment.
- What is NLP? Natural Language Processing is a field of artificial intelligence that focuses on the interaction between computers and humans through natural language.
- How is NLP used in finance? NLP is used for sentiment analysis, fraud detection, and risk management, helping organizations make data-driven decisions.
- What are the benefits of using NLP in the financial sector? Benefits include improved investment strategies, enhanced security, and proactive risk management.
- Can NLP help in regulatory compliance? Yes, NLP can analyze regulatory documents and ensure compliance with evolving laws and regulations.

Challenges in NLP Development
Despite the remarkable progress in the field of Natural Language Processing (NLP), several challenges continue to hinder its development. One of the most pressing issues is language ambiguity, which arises when words or phrases have multiple meanings. This can lead to confusion and misinterpretation, particularly in nuanced conversations. For instance, consider the word "bank." It could refer to a financial institution or the side of a river, depending on the context. NLP systems must be adept at understanding these subtleties to provide accurate responses.
Another significant challenge is the need for cultural context understanding. Language is deeply intertwined with culture, and phrases that make perfect sense in one cultural setting may be completely alien in another. This can create barriers in communication, especially for systems designed to operate globally. Imagine a chatbot programmed to assist customers in various countries; without cultural awareness, it might inadvertently offend or confuse users, leading to poor user experiences.
The diversity of datasets is also a crucial factor in the effectiveness of NLP models. Many existing models are trained on datasets that lack representation from different demographics, which can result in biased outcomes. For example, if a model primarily learns from English-speaking data, it might struggle with understanding idiomatic expressions used in other languages or dialects. This lack of diversity not only affects accuracy but also raises ethical concerns about fairness and inclusivity in AI applications.
Moreover, the complexity of human language itself poses a challenge. Languages are not static; they evolve over time, adopting new words and phrases while discarding others. This dynamic nature means that NLP systems must continually adapt to stay relevant. Keeping up with slang, jargon, and emerging linguistic trends requires constant updates and retraining of models, which can be resource-intensive.
In summary, while NLP has made significant strides, it is not without its challenges. Addressing issues such as language ambiguity, cultural context, data diversity, and the evolving nature of language is essential for the continued advancement of NLP technologies. By focusing on these areas, developers can create more robust and inclusive systems that better serve users across the globe.
- What is Natural Language Processing (NLP)? NLP is a branch of artificial intelligence that focuses on the interaction between computers and humans through natural language.
- What are some common applications of NLP? NLP is used in various applications, including chatbots, sentiment analysis, language translation, and voice recognition.
- What challenges does NLP face? NLP faces challenges like language ambiguity, cultural context understanding, data diversity, and the dynamic nature of human language.
- How can bias in NLP models be minimized? Ensuring diverse and representative training datasets is crucial for minimizing bias and achieving fair outcomes in NLP applications.
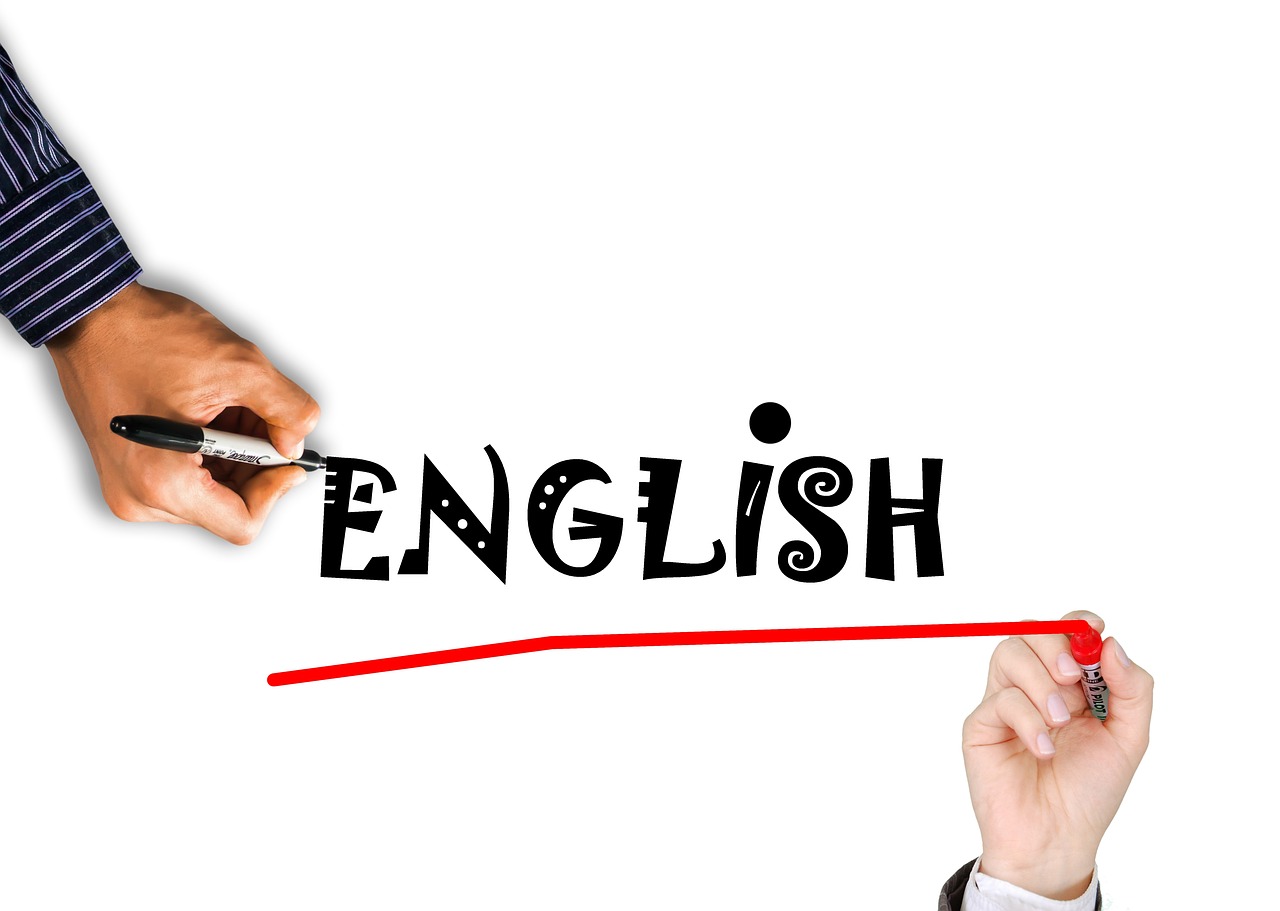
Language Ambiguity Issues
Language ambiguity is like a double-edged sword; it can enrich communication but also complicate it. When we speak or write, many words and phrases can have multiple meanings depending on context. For instance, consider the word "bank." It could refer to a financial institution where you deposit money, or it could mean the side of a river. This multiplicity of meanings poses significant challenges for Natural Language Processing (NLP)
To illustrate, let’s look at some common types of language ambiguity:
- Lexical Ambiguity: This occurs when a word has more than one meaning. For example, "bat" can refer to a flying mammal or a piece of sports equipment.
- Syntactic Ambiguity: This happens when a sentence can be parsed in multiple ways. For example, "I saw the man with the telescope" can mean that you used a telescope to see the man or that the man had a telescope.
- Semantic Ambiguity: This arises when a sentence has multiple interpretations. For example, "He gave her cat food" could imply he gave food intended for cats or food for her cat.
These ambiguities can lead to misunderstandings and misinterpretations, which is particularly problematic in applications like chatbots or virtual assistants. Imagine asking a voice assistant to "play the bank." Depending on the context, it might play a song about a riverbank or give you information about your bank account! This is where the challenge lies: NLP systems need to discern the intended meaning from the context in which words are used.
Moreover, cultural context adds another layer of complexity. Different cultures may use the same words in unique ways, leading to varied interpretations. For instance, idiomatic expressions can confuse NLP systems. The phrase "kick the bucket" means to die in English, but if taken literally, it could lead to a rather strange response from an AI. Therefore, understanding cultural nuances is crucial for enhancing the effectiveness of NLP applications.
In summary, while advancements in NLP are paving the way for better human-computer interactions, addressing language ambiguity remains a significant hurdle. Researchers and developers are continuously working on algorithms that can better understand context, thereby improving the accuracy of language interpretation. As we move forward, the goal is to create systems that not only understand words but also the underlying meanings, emotions, and cultural nuances that come with them.
- What is language ambiguity? Language ambiguity refers to situations where a word, phrase, or sentence can have multiple meanings or interpretations.
- Why is language ambiguity a problem for NLP? It complicates the task of accurately interpreting human language, leading to misunderstandings and incorrect responses from AI systems.
- How can NLP systems overcome language ambiguity? By improving contextual understanding and utilizing diverse datasets that include various language uses and cultural contexts.
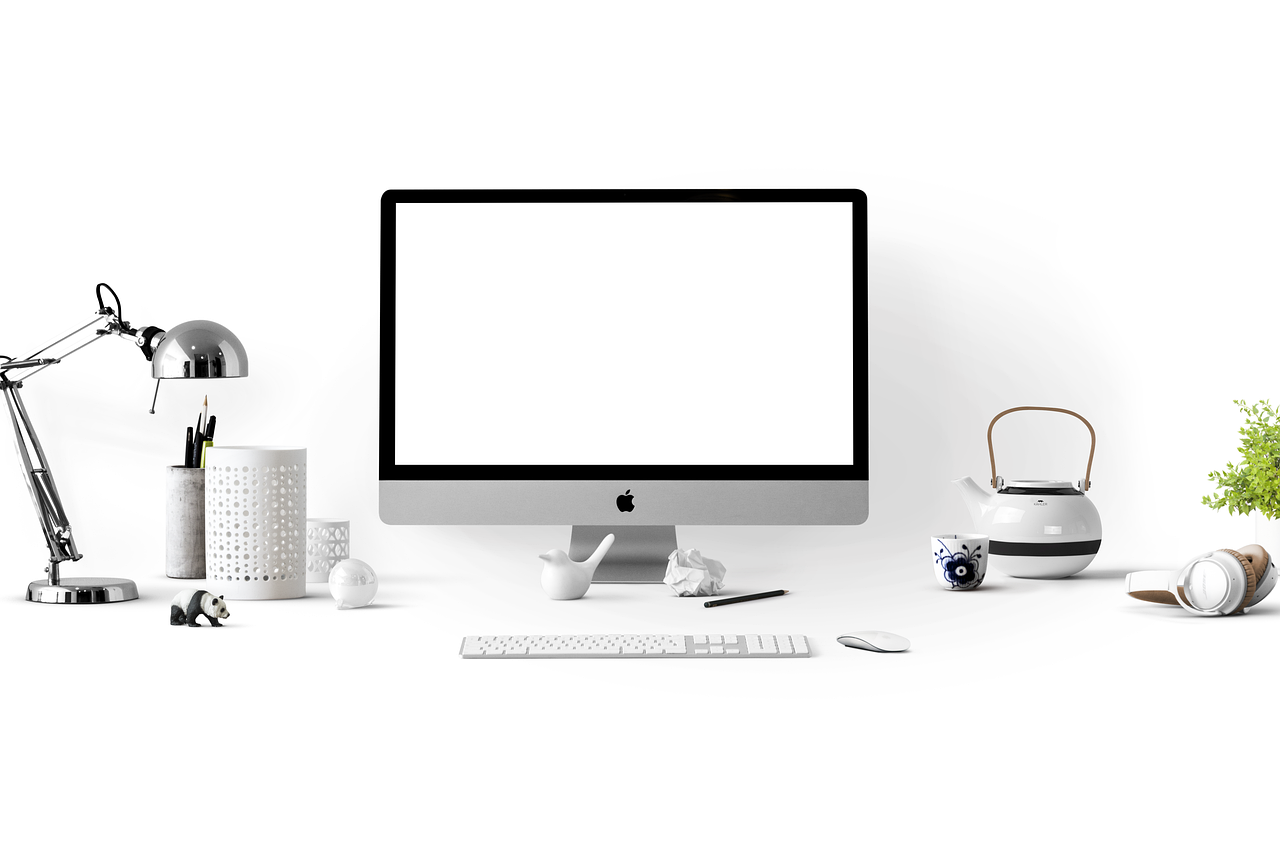
Data Diversity and Bias
In the ever-evolving world of Natural Language Processing (NLP), one of the most pressing issues that researchers and developers face is the challenge of data diversity and bias. Imagine trying to learn a new language by only listening to a handful of speakers from one region; your understanding would be limited and skewed. Similarly, NLP models trained on non-diverse datasets can lead to skewed interpretations and outputs. This lack of diversity can manifest in various ways, including cultural biases, gender stereotypes, and even regional dialect misunderstandings.
To put it simply, if an NLP model is predominantly trained on data from a specific demographic, it may struggle to accurately understand or generate language for individuals outside that group. This can result in significant implications, especially in applications that require fairness and inclusivity, such as healthcare, finance, and customer service. For example, a virtual assistant trained primarily on data from one cultural background might misinterpret requests from users of different backgrounds, leading to frustrating experiences.
Moreover, the presence of bias in NLP systems can perpetuate existing inequalities. Consider the following points:
- Gender Bias: NLP models may reflect societal biases, leading to outputs that reinforce stereotypes. For instance, associating certain professions with a specific gender can skew user interactions.
- Cultural Bias: Models that lack exposure to diverse cultural contexts may misinterpret idioms, slang, or colloquialisms, resulting in inaccurate responses.
- Regional Bias: Language varies significantly across regions. An NLP model trained predominantly on American English might struggle with British English nuances, leading to misunderstandings.
To combat these challenges, it is essential to curate datasets that are not only diverse but also representative of the wide range of human experiences. This means actively seeking out and including voices from various backgrounds, cultures, and languages in the training data. Additionally, employing techniques such as adversarial training and data augmentation can help to mitigate bias by exposing models to a broader spectrum of language use.
Ultimately, addressing data diversity and bias in NLP is not just a technical challenge; it’s a social responsibility. As we continue to develop and deploy these technologies, we must remain vigilant and proactive in ensuring that they serve all users fairly and equitably. By fostering inclusivity in our datasets and being mindful of the implications of bias, we can create NLP systems that are not only more accurate but also more just.
- What is data diversity in NLP?
Data diversity refers to the inclusion of a wide range of demographic, cultural, and linguistic backgrounds in the datasets used to train NLP models. This helps ensure that the models can understand and generate language accurately across different groups. - How does bias affect NLP models?
Bias in NLP models can lead to unfair or inaccurate outputs, reinforcing stereotypes and misrepresenting users from underrepresented demographics. - What can be done to reduce bias in NLP?
To reduce bias, it is crucial to use diverse datasets, implement adversarial training techniques, and continuously evaluate and update models to reflect a broader range of human experiences.

The Future of NLP Technologies
The future of NLP technologies is not just bright; it's dazzling! As we stand on the brink of a new era in artificial intelligence, the advancements in natural language processing are set to redefine how we interact with machines. Imagine a world where your devices understand you as well as your closest friends do. This isn't just a dream—it's becoming a reality!
One of the most exciting areas of development is in contextual understanding. Current NLP systems often struggle with nuances, sarcasm, and emotional undertones that are second nature to humans. However, ongoing research is paving the way for machines to better grasp these subtleties. For instance, future NLP models will be able to differentiate between a casual comment and a serious concern, enhancing their ability to respond appropriately. This kind of advancement will lead to more engaging and satisfying interactions, making technology feel more human-like.
Moreover, the push for multilingual capabilities is gaining momentum. In our interconnected world, language barriers can hinder communication and collaboration. Imagine a scenario where a business meeting includes participants from different countries, and everyone can communicate seamlessly in their preferred language. With the development of multilingual NLP models, this is becoming increasingly feasible. By breaking down these barriers, we can foster inclusivity and accessibility, allowing more people to participate in global conversations.
To illustrate the potential impact of these advancements, consider the following table that outlines some key future trends in NLP technologies:
Trend | Description |
---|---|
Contextual Understanding | Improving machines' ability to understand nuances in conversation. |
Multilingual Capabilities | Facilitating seamless communication across different languages. |
Ethical Considerations | Integrating ethical frameworks into AI systems to ensure responsible use. |
Personalized Interactions | Creating tailored experiences based on user preferences and behavior. |
Additionally, the incorporation of ethical considerations into NLP development cannot be overstated. As AI systems become more integrated into our daily lives, ensuring that they are designed responsibly is crucial. This includes addressing issues like bias in language processing and ensuring that all voices are represented fairly. By prioritizing ethics, we can build trust in these technologies, making them more acceptable and beneficial to society.
Finally, the idea of personalized interactions is becoming a reality. Future NLP systems will not only respond to commands but will also learn from users over time. They'll adapt to individual preferences, making interactions feel more intuitive and tailored. This evolution will revolutionize customer service, education, and even entertainment, creating experiences that are not just reactive but proactively engaging.
- What is NLP? Natural Language Processing (NLP) is a field of AI that focuses on the interaction between computers and humans through natural language.
- How will NLP improve in the future? Future improvements will focus on enhancing contextual understanding, multilingual capabilities, and ethical considerations.
- Why is ethical consideration important in NLP? Ethical considerations are essential to prevent bias and ensure that AI systems are fair and representative of all users.
- What industries will benefit from advancements in NLP? Industries such as healthcare, finance, and education will see significant benefits from advancements in NLP technologies.
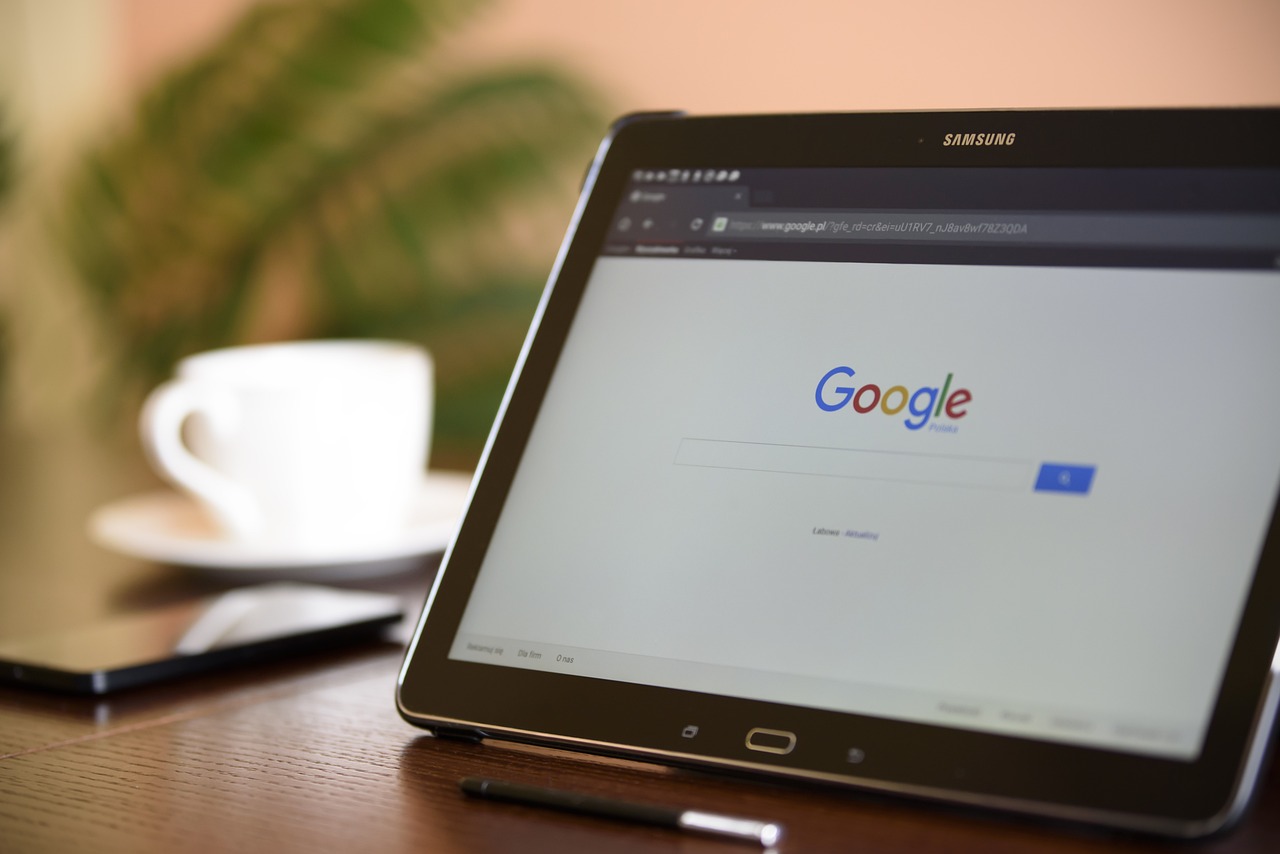
Contextual Understanding Improvements
As we dive deeper into the realm of natural language processing (NLP), one of the most exciting advancements is in the area of contextual understanding. Imagine having a conversation with a friend where every nuance, every subtle shift in tone, and every underlying emotion is perfectly understood. This is the future that NLP is striving to achieve. The ability of machines to comprehend context not only enhances their conversational abilities but also makes interactions feel more human.
Recent developments in NLP technologies have led to the emergence of models that can analyze context in a way that was previously unimaginable. These models are designed to consider the surrounding words and phrases, allowing them to interpret meaning based on context rather than relying solely on keyword matching. For instance, the word "bank" can refer to a financial institution or the side of a river. A model equipped with contextual understanding can discern the intended meaning based on the rest of the conversation.
To illustrate the significance of contextual understanding, consider the following scenarios:
- Customer Support: When a customer asks about a refund, a context-aware system can recognize the preceding conversation about a faulty product, thus providing a more accurate and relevant response.
- Content Generation: In writing applications, context-aware models can generate text that aligns with the tone and style of the previous content, making it more cohesive and engaging.
- Sentiment Analysis: By understanding the context in which words are used, NLP systems can better gauge the sentiment behind a statement, allowing businesses to respond appropriately to customer feedback.
One of the key breakthroughs in achieving this level of understanding is the development of transformer-based models, such as BERT (Bidirectional Encoder Representations from Transformers) and GPT (Generative Pre-trained Transformer). These models utilize attention mechanisms that allow them to weigh the importance of different words in a sentence based on their context. This is akin to how we, as humans, focus on specific words or phrases during a conversation to grasp the overall message.
Furthermore, ongoing research is focused on improving these models' ability to handle longer contexts. Traditional NLP systems often struggle with maintaining coherence over extended conversations or texts, but advancements in memory-augmented networks are paving the way for more effective long-term contextual understanding.
As we look to the future, the implications of enhanced contextual understanding in NLP are profound. From more intuitive virtual assistants that can engage in meaningful dialogues to sophisticated content creation tools that generate articles or stories with depth and relevance, the possibilities are endless. However, it's crucial to approach these advancements with caution, ensuring that ethical considerations are integrated into the development of these technologies.
In summary, the improvements in contextual understanding represent a significant leap forward in the evolution of NLP. The journey towards achieving a truly conversational AI is well underway, and as these technologies continue to evolve, we can expect a future where machines not only understand our words but also the emotions and intentions behind them.
- What is contextual understanding in NLP?
Contextual understanding refers to the ability of NLP systems to interpret the meaning of words and phrases based on their surrounding context, rather than relying solely on individual words.
- How do transformer models improve contextual understanding?
Transformer models, like BERT and GPT, utilize attention mechanisms that allow them to focus on relevant parts of the input text, enabling better interpretation of context.
- What are the practical applications of enhanced contextual understanding?
Applications include improved customer support, more coherent content generation, and more accurate sentiment analysis, among others.
- What challenges remain in achieving perfect contextual understanding?
Challenges include handling long-term context, understanding cultural nuances, and ensuring ethical use of AI technologies.
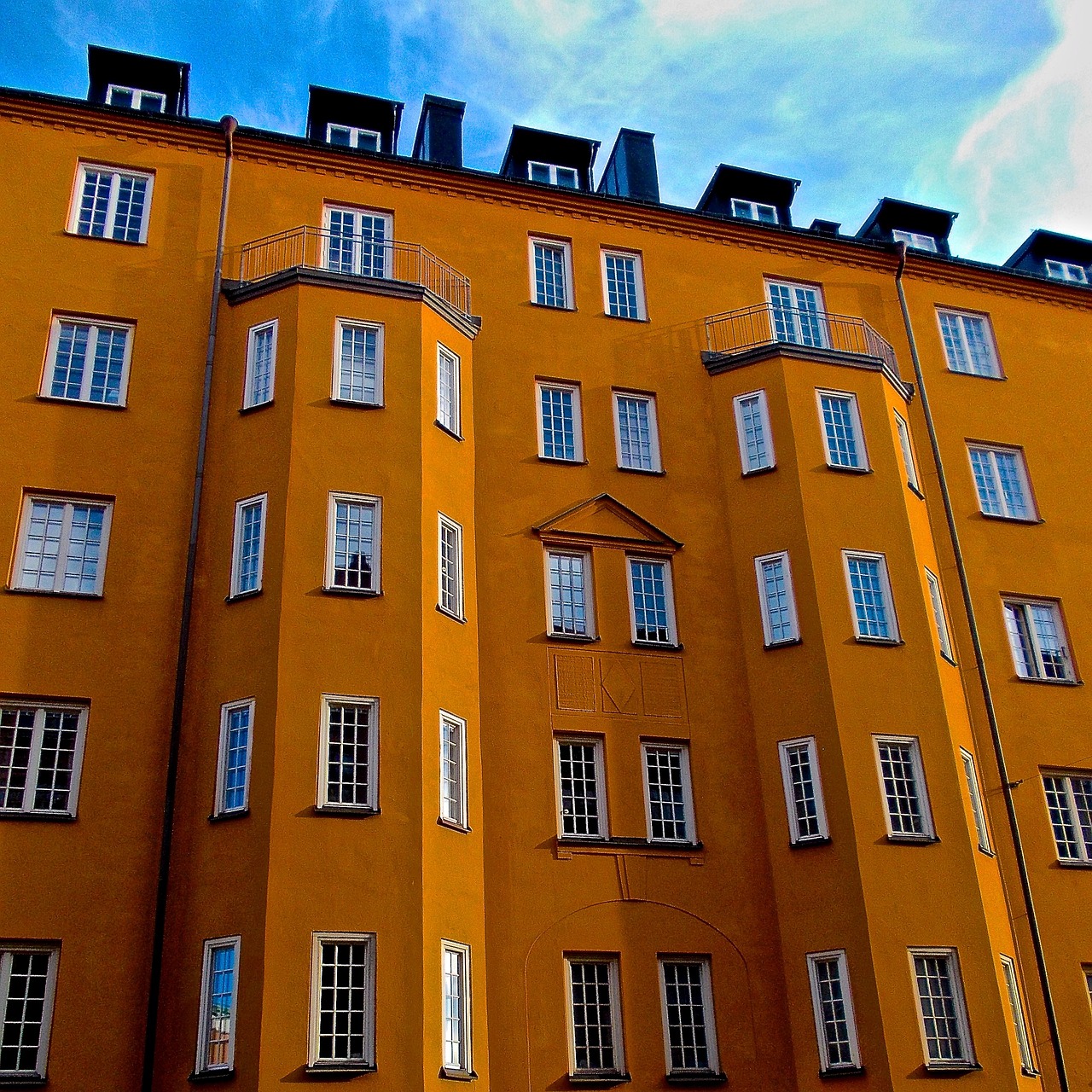
Multilingual Capabilities
In our increasingly interconnected world, the demand for in natural language processing (NLP) has never been more critical. Imagine a world where language barriers dissolve, allowing people from different cultures and backgrounds to communicate effortlessly. This is not just a dream; it’s becoming a reality thanks to the rapid advancements in NLP technologies. By developing multilingual models, we are paving the way for seamless communication and collaboration across the globe.
One of the key innovations in multilingual NLP is the ability to understand and generate text in various languages without needing separate models for each one. This is achieved through techniques such as transfer learning, where knowledge gained from one language can be applied to another. Consequently, organizations can save time and resources while also enhancing the user experience. For example, a single AI model can handle customer queries in multiple languages, providing consistent and accurate responses.
Furthermore, the integration of multilingual capabilities in NLP can significantly enhance the accessibility of information. Consider the impact on education: students from non-English speaking backgrounds can access high-quality learning materials in their native languages. This not only fosters inclusivity but also promotes a more diverse learning environment. In healthcare, multilingual NLP can help bridge communication gaps between patients and providers, ensuring that everyone receives the care they need, regardless of language proficiency.
However, developing effective multilingual NLP systems is not without its challenges. The nuances of different languages, including idioms, cultural references, and grammatical structures, can complicate the training of these models. To tackle this, researchers are focusing on creating more robust datasets that accurately represent the diversity of languages. For example, consider the following table that outlines some of the key factors influencing multilingual NLP development:
Factor | Description |
---|---|
Language Diversity | Incorporating a wide range of languages to ensure comprehensive understanding. |
Cultural Context | Understanding cultural nuances that affect language use and interpretation. |
Data Quality | Ensuring high-quality, representative datasets to minimize bias. |
Technological Integration | Combining NLP with other AI technologies for enhanced performance. |
As we look to the future, the potential for multilingual NLP is vast. With ongoing research and development, we can expect to see even more sophisticated models that not only understand multiple languages but also adapt to the specific needs of users. The goal is to create systems that are not just tools, but companions that enhance our ability to connect with one another. Imagine AI that can not only translate your words but also convey the emotion and intent behind them, making conversations richer and more meaningful.
In conclusion, the advancements in multilingual capabilities within NLP are transforming how we communicate. By breaking down language barriers, we are fostering a more inclusive and connected world. As we continue to innovate and improve these technologies, the possibilities are endless, and the dream of global communication is becoming more tangible every day.
- What is multilingual NLP? Multilingual NLP refers to the ability of natural language processing systems to understand and generate text in multiple languages.
- How does multilingual NLP benefit businesses? It allows businesses to serve a global audience, improve customer service, and enhance user experiences by providing support in various languages.
- What are the challenges of developing multilingual NLP? Key challenges include language diversity, cultural context understanding, and the need for high-quality, representative datasets.
- What is the future of multilingual NLP? The future looks promising, with advancements aimed at improving contextual understanding and creating more inclusive AI systems.
Frequently Asked Questions
- What is Natural Language Processing (NLP)?
NLP is a field of artificial intelligence that focuses on the interaction between computers and humans through natural language. It enables machines to understand, interpret, and generate human language in a way that is both meaningful and useful.
- How are advancements in NLP benefiting industries?
Advancements in NLP are transforming various industries by enhancing data analysis, automating customer service, and personalizing learning experiences. For instance, in healthcare, NLP helps in extracting insights from unstructured data, while in finance, it aids in sentiment analysis and fraud detection.
- What are some challenges faced by NLP technologies?
NLP technologies face several challenges, including language ambiguity, which complicates interpretation, and the need for diverse datasets to minimize bias. These issues can lead to inaccuracies in understanding and responding to human language.
- How does NLP improve patient interactions in healthcare?
NLP enhances patient interactions through AI-driven chatbots and virtual assistants that provide timely responses and personalized health information, ultimately improving patient engagement and satisfaction.
- What is the future of NLP technologies?
The future of NLP looks bright, with ongoing research focusing on improving contextual understanding, developing multilingual capabilities, and integrating ethical considerations. These advancements aim to create more sophisticated and inclusive systems that foster better communication across different languages.
- Can NLP understand multiple languages?
Yes, the development of multilingual NLP models is underway, which aims to break down language barriers. This allows for seamless communication and collaboration in a globalized world, promoting inclusivity and accessibility.