Understanding the Role of AI in Predictive Maintenance
In today's fast-paced industrial landscape, the integration of artificial intelligence (AI) into predictive maintenance strategies is not just a trend—it's a necessity. Imagine a world where machines can predict their own failures before they happen, saving companies from costly downtime and repairs. This is not a distant dream; it's the reality that AI is helping to create. By leveraging vast amounts of data and sophisticated algorithms, AI enhances the ability to foresee potential issues, leading to improved operational efficiency and significant cost savings across various industries.
So, what exactly is predictive maintenance? At its core, it's a proactive approach to maintenance that uses data analysis tools to predict equipment failures before they occur. This means less unplanned downtime and a longer lifespan for machinery. With AI, the predictive maintenance process becomes even more powerful. AI technologies can sift through massive datasets at lightning speed, uncovering patterns and insights that would be impossible for humans to identify alone. This capability not only enhances decision-making processes but also leads to better maintenance strategies that are tailored to the specific needs of each piece of equipment.
But how does AI fit into the larger picture of predictive maintenance? It starts with data collection. Effective predictive maintenance relies on robust data collection methods, and this is where sensors and IoT (Internet of Things) devices come into play. These technologies continuously gather real-time data from machinery, providing a treasure trove of information that AI algorithms can analyze. For instance, imagine a factory floor where every machine is equipped with smart sensors that monitor their operational status. This continuous stream of data enables AI to make accurate predictions about when maintenance should occur, ensuring that machines are serviced just in time to prevent failures.
Moreover, the integration of AI in predictive maintenance is not without its challenges. Organizations often face hurdles such as data quality issues and integration complexities with existing systems. High-quality data is crucial for accurate predictions, yet many companies struggle with inconsistent or incomplete data. This can hinder the effectiveness of AI-driven solutions. Additionally, integrating AI with legacy systems can be complex and may require significant investment in technology and training. However, the benefits—such as reduced maintenance costs, improved asset reliability, and enhanced operational efficiency—far outweigh these challenges.
As we look to the future, the role of AI in predictive maintenance is only set to grow. Emerging machine learning techniques promise to enhance predictive capabilities, allowing for more sophisticated analyses and improved accuracy in failure predictions. Furthermore, as automation technology continues to evolve, we can expect to see even more autonomous systems capable of self-diagnosing and resolving issues. In this dynamic environment, companies that embrace AI-driven predictive maintenance will not only stay ahead of the competition but will also redefine what it means to operate efficiently in the industrial sector.
- What is predictive maintenance?
Predictive maintenance is a proactive maintenance strategy that uses data analysis tools to predict equipment failures before they occur, minimizing downtime and extending the lifespan of machinery. - How does AI enhance predictive maintenance?
AI enhances predictive maintenance by analyzing large datasets quickly, identifying patterns, and providing insights that lead to more accurate predictions and better decision-making. - What are the main challenges in implementing AI for predictive maintenance?
Some challenges include data quality issues, integration complexities with existing systems, and the need for skilled personnel to manage AI systems. - What are the benefits of AI-driven predictive maintenance?
Benefits include reduced maintenance costs, improved asset reliability, and enhanced operational efficiency.
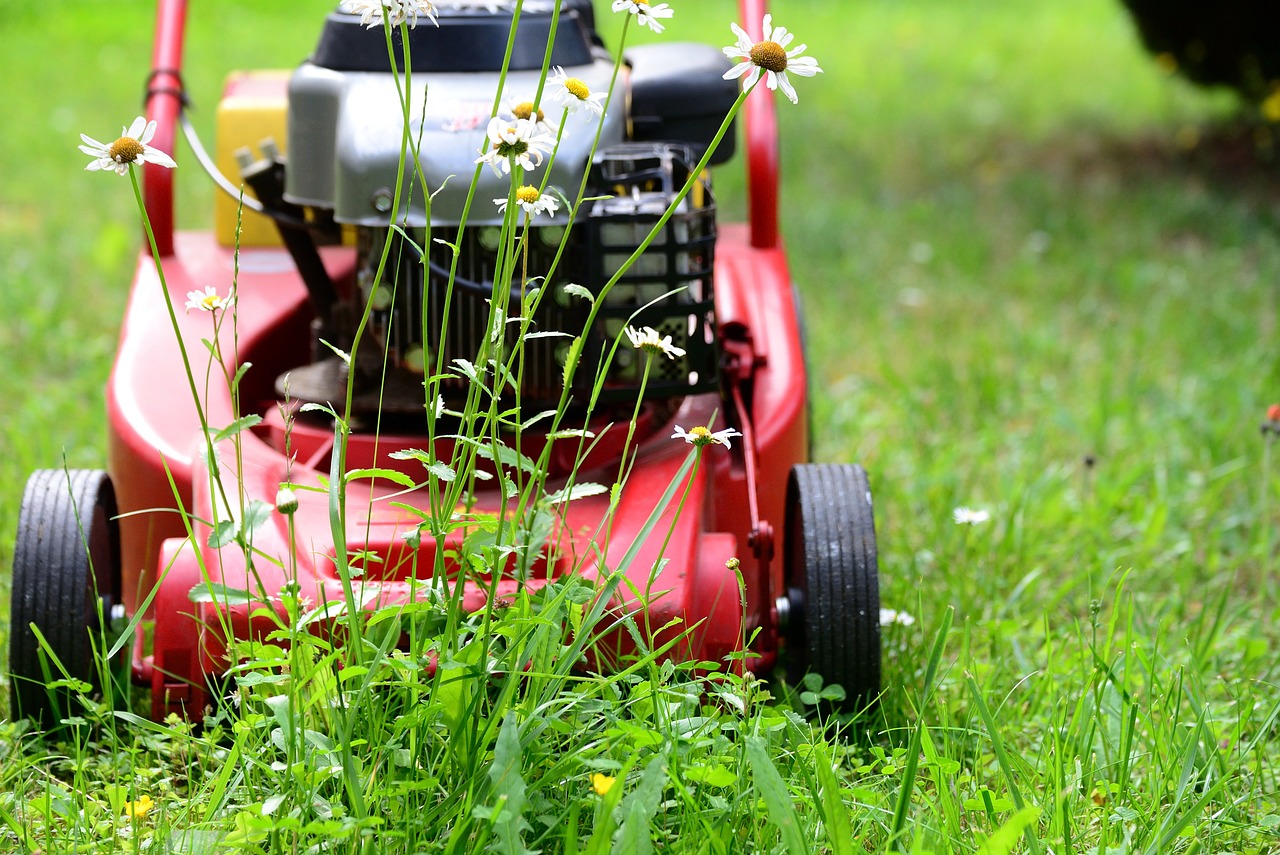
What is Predictive Maintenance?
Predictive maintenance is a game-changing strategy that uses data analysis to foresee equipment failures before they actually happen. Imagine being able to predict the weather; you wouldn't head out without an umbrella if you knew rain was coming, right? Similarly, predictive maintenance empowers organizations to anticipate equipment issues, allowing them to take proactive measures. This approach not only minimizes unexpected downtime but also extends the lifespan of machinery, ultimately leading to significant cost savings.
At its core, predictive maintenance leverages various tools and technologies to analyze data collected from machinery. This data can come from a range of sources, including sensors that monitor equipment performance in real-time. By utilizing advanced analytics, organizations can identify patterns and trends that might indicate an impending failure. This proactive approach is akin to getting a health check-up; just as regular check-ups can catch health issues before they escalate, predictive maintenance can spot potential equipment failures before they disrupt operations.
One of the most fascinating aspects of predictive maintenance is its reliance on big data and machine learning. These technologies allow for the processing of vast amounts of data at lightning speed. For instance, companies can collect data on various parameters, such as:
- Vibration levels
- Temperature fluctuations
- Operational hours
- Wear and tear indicators
By analyzing this data, organizations can create a detailed picture of their equipment's health and performance. This not only helps in predicting failures but also aids in scheduling maintenance activities at optimal times, thereby reducing the overall maintenance burden.
In summary, predictive maintenance is a proactive approach that transforms how organizations manage their assets. By harnessing the power of data, companies can not only avoid costly downtimes but also enhance the efficiency and longevity of their equipment. As we delve deeper into the role of AI in predictive maintenance, it becomes clear that this innovative strategy is set to revolutionize various industries.
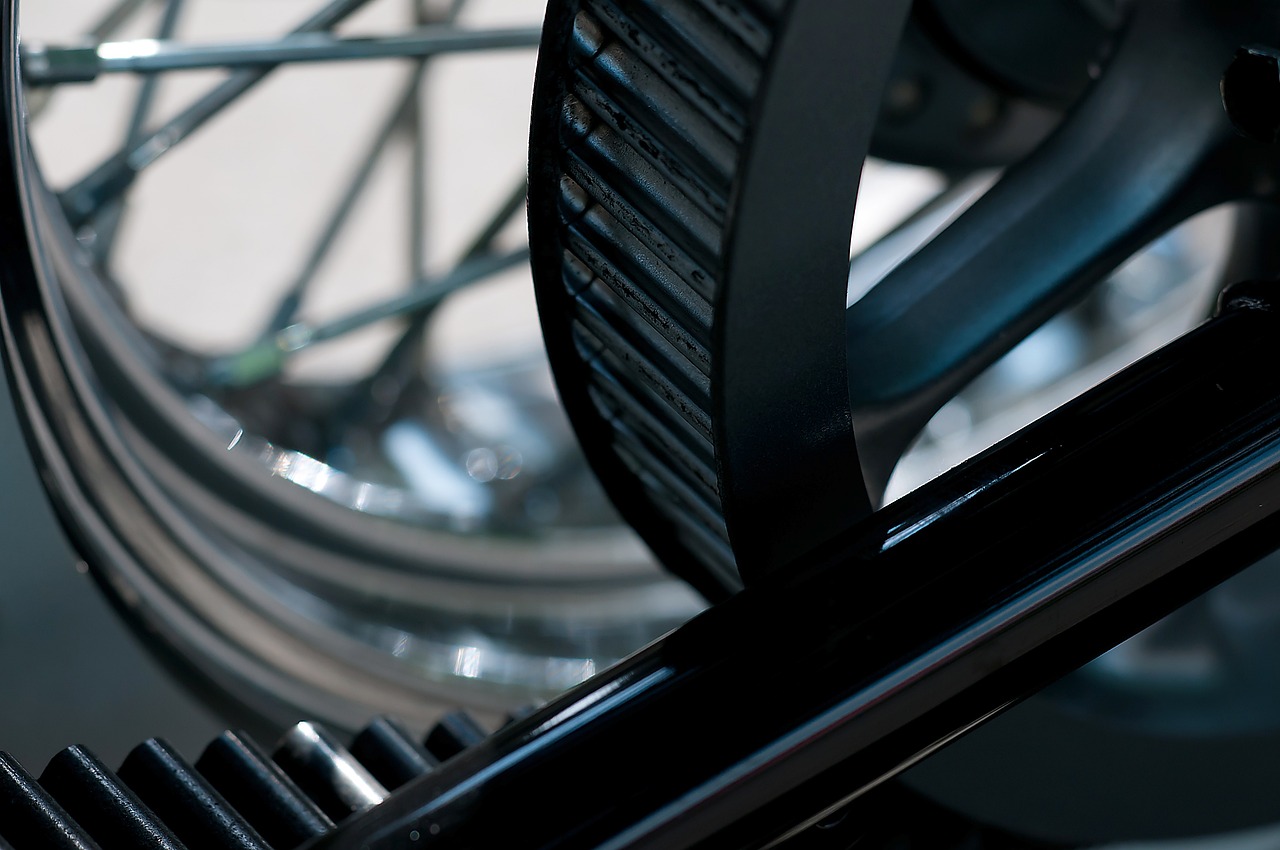
The Importance of AI in Predictive Maintenance
In today's fast-paced industrial landscape, the integration of artificial intelligence (AI) into predictive maintenance strategies is not just a trend; it's a game changer. The importance of AI in this realm cannot be overstated, as it significantly enhances the accuracy and efficiency of maintenance operations. By leveraging AI technologies, organizations can sift through vast amounts of data at lightning speed, allowing them to make informed decisions that were once impossible. Imagine trying to find a needle in a haystack—AI acts like a magnet, pulling out the critical insights needed to prevent equipment failures before they occur.
One of the most compelling advantages of AI in predictive maintenance is its ability to analyze historical and real-time data to identify patterns that indicate potential issues. For instance, consider a manufacturing plant where machines are constantly in operation. By utilizing AI, the system can learn from past breakdowns, recognize similar patterns in real-time data, and alert maintenance teams to potential failures. This proactive approach not only minimizes unplanned downtime but also extends the lifespan of machinery, ultimately leading to significant cost savings.
Furthermore, AI enhances decision-making processes by providing predictive insights that are data-driven rather than intuition-based. This shift from reactive to proactive maintenance strategies means that organizations can allocate resources more efficiently. For example, instead of scheduling routine maintenance checks at arbitrary intervals, AI allows for maintenance to be performed based on actual equipment condition and usage patterns. This tailored approach ensures that maintenance efforts are focused where they are most needed, maximizing operational efficiency.
In addition to improving predictions and decision-making, AI also plays a crucial role in fostering a culture of continuous improvement within organizations. By continuously learning from new data, AI systems evolve and refine their predictive capabilities over time. This adaptability is essential in industries where technology and operational parameters are constantly changing. As a result, organizations that embrace AI-driven predictive maintenance are not only better equipped to handle current challenges but are also prepared for future advancements.
To summarize, the integration of AI into predictive maintenance strategies offers a multitude of benefits, including:
- Enhanced Accuracy: AI's ability to analyze large datasets ensures more precise predictions.
- Cost Efficiency: Reducing unplanned downtime leads to significant savings.
- Extended Equipment Lifespan: Timely interventions help maintain machinery in optimal condition.
- Data-Driven Decision Making: Decisions are based on actual data rather than guesswork.
As we move forward, the importance of AI in predictive maintenance will only grow, paving the way for smarter, more efficient operations across various industries. Organizations that harness the power of AI will not only stay ahead of the competition but will also redefine the standards of operational excellence.
Q: What is predictive maintenance?
A: Predictive maintenance is a proactive approach that uses data analysis tools to predict equipment failures before they occur, minimizing unplanned downtime and extending machinery lifespan.
Q: How does AI improve predictive maintenance?
A: AI enhances predictive maintenance by analyzing large datasets quickly, identifying patterns, and providing data-driven insights for better decision-making.
Q: What are the main benefits of AI-driven predictive maintenance?
A: The main benefits include reduced maintenance costs, improved asset reliability, and enhanced operational efficiency across industries.
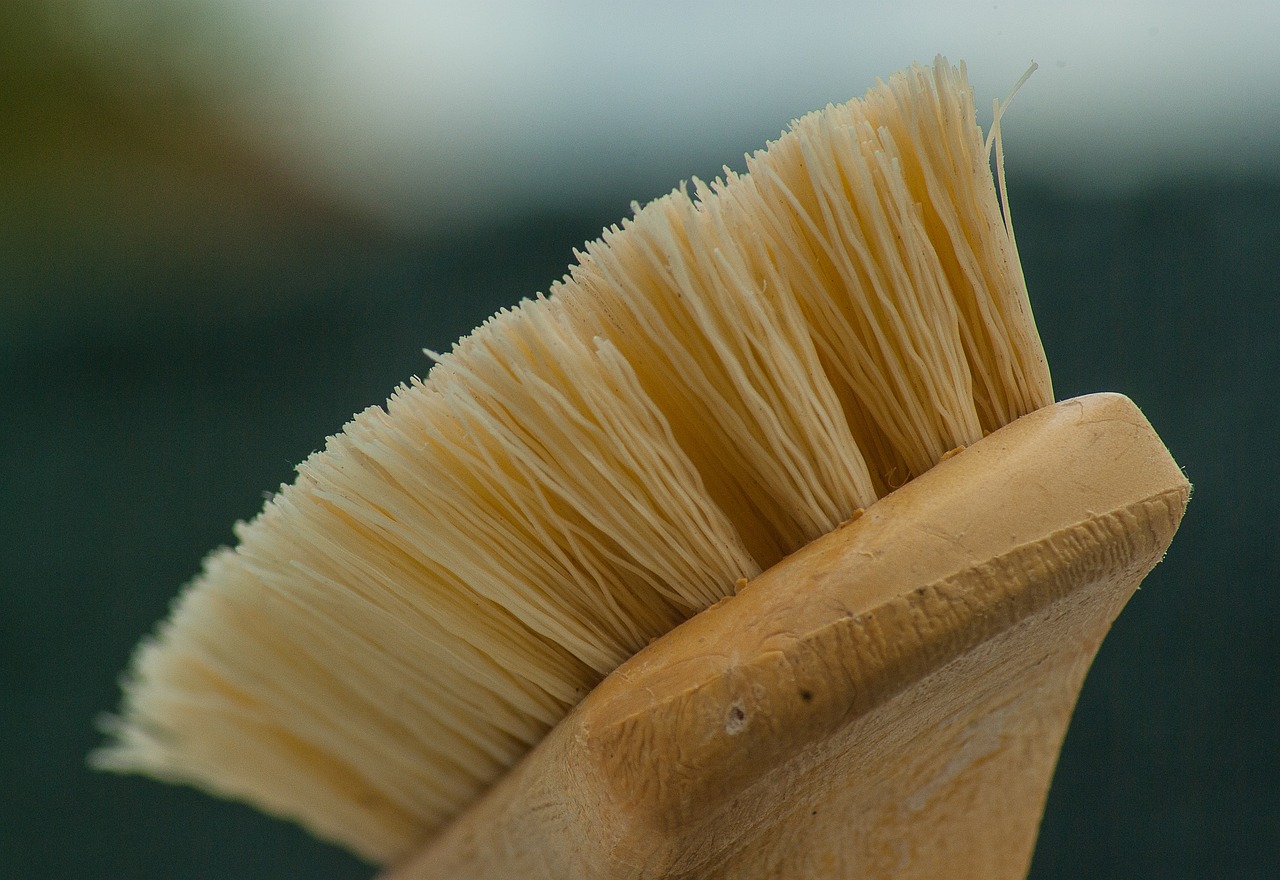
Data Collection Techniques
When it comes to predictive maintenance, the foundation of success lies in effective . The goal is to gather accurate and timely data that can be analyzed to forecast potential equipment failures. To achieve this, organizations increasingly rely on advanced technologies such as sensors and the Internet of Things (IoT). These tools not only facilitate real-time monitoring but also provide a wealth of information that enhances the predictive capabilities of AI systems.
One of the primary methods of data collection involves the use of various sensors strategically placed on machinery. These sensors can monitor different parameters, including:
- Vibration Sensors: These detect unusual vibrations in equipment, which can indicate wear and tear or potential failures.
- Temperature Sensors: Monitoring temperature fluctuations helps in identifying overheating issues before they lead to significant damage.
- Acoustic Sensors: These capture sound waves, allowing for the detection of unusual noises that may suggest mechanical problems.
Integrating these sensors with IoT technology creates a powerful synergy. IoT devices enable continuous data transmission to centralized systems, where AI algorithms can analyze the information in real-time. This integration allows organizations to make informed decisions based on current equipment conditions rather than relying solely on historical data. The continuous flow of data also means that maintenance teams can react swiftly to any anomalies, thereby preventing costly downtime.
Moreover, the data collected through these techniques can be enriched with additional contextual information, such as operational schedules and environmental conditions. This holistic approach provides a comprehensive view of equipment health, allowing for more accurate predictions and better maintenance strategies. For instance, understanding how environmental factors like humidity and temperature affect machinery can lead to more tailored maintenance plans.
As organizations embrace these data collection techniques, they must also consider the importance of data quality. High-quality data is essential for effective predictive maintenance. Inconsistent or incomplete data can lead to misinterpretations and potentially costly mistakes. Therefore, investing in reliable data collection methods and ensuring proper calibration of sensors is crucial for maximizing the benefits of predictive maintenance.
In summary, effective data collection techniques form the backbone of successful predictive maintenance strategies. By leveraging sensors and IoT integration, organizations can gather valuable insights that enhance their predictive capabilities, ultimately leading to improved operational efficiency and reduced costs.
- What is predictive maintenance?
Predictive maintenance is a proactive approach that uses data analysis to predict equipment failures before they occur, minimizing unplanned downtime. - How does AI enhance predictive maintenance?
AI analyzes large datasets quickly, leading to more accurate predictions and better decision-making processes for maintenance strategies. - What role do sensors play in predictive maintenance?
Sensors monitor various parameters of machinery, providing real-time data that is crucial for identifying potential failures. - What challenges are associated with implementing AI for predictive maintenance?
Challenges include data quality issues, integration complexities, and the need for skilled personnel to manage AI systems.
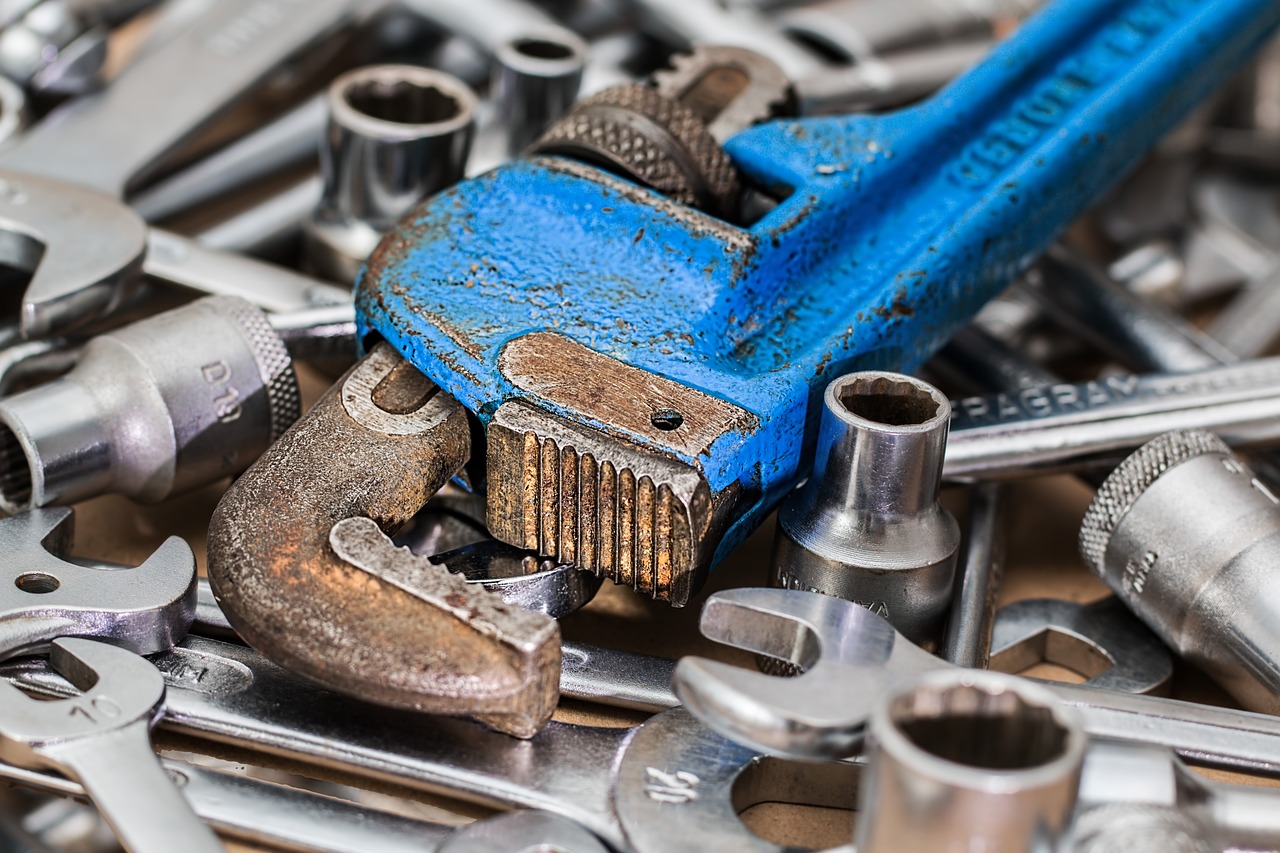
IoT Integration
Integrating the Internet of Things (IoT) into predictive maintenance strategies is like giving your equipment a voice—it allows them to communicate their needs in real-time. Imagine walking into a room filled with machinery that can tell you exactly when they need servicing or if something is about to go wrong. This level of insight is not just a dream; it's a reality made possible through IoT integration.
At its core, IoT involves connecting devices to the internet, enabling them to send and receive data. In the context of predictive maintenance, IoT devices—like sensors and smart meters—continuously monitor equipment performance and gather vast amounts of data. This data is critical because it helps AI algorithms identify patterns that may indicate potential failures. For instance, a vibration sensor on a motor can detect unusual vibrations that signal a problem before it escalates into a costly breakdown.
One of the key benefits of IoT integration is the ability to perform real-time monitoring. This means that maintenance teams can access live data from anywhere, allowing for quicker decision-making. Instead of waiting for a scheduled maintenance check, organizations can act on the insights provided by IoT devices. This proactive approach not only minimizes downtime but also helps in planning maintenance activities more effectively.
Moreover, the data collected through IoT devices can be visualized in dashboards, making it easier for maintenance teams to interpret the information. These dashboards can display metrics such as:
- Operating temperatures
- Vibration levels
- Energy consumption
By having access to this data, decision-makers can prioritize maintenance tasks based on urgency and potential impact, ensuring that resources are allocated efficiently.
However, integrating IoT into existing systems does come with its challenges. Organizations must ensure that their infrastructure can support the influx of data and that they have the necessary cybersecurity measures in place to protect sensitive information. Additionally, training personnel to utilize these advanced systems effectively is crucial for maximizing the benefits of IoT integration.
In summary, the integration of IoT into predictive maintenance strategies is a transformative step that enhances operational efficiency and reliability. By empowering machinery to communicate their status, organizations can stay ahead of potential issues, reduce downtime, and ultimately save costs. It's a game-changer that shifts maintenance from a reactive to a proactive approach, paving the way for smarter, more efficient operations.
- What is IoT integration in predictive maintenance?
IoT integration involves connecting devices to the internet to gather real-time data, allowing for proactive maintenance based on equipment performance. - How does IoT improve predictive maintenance?
IoT enhances predictive maintenance by providing continuous monitoring and data collection, enabling quicker decision-making and minimizing downtime. - What challenges are associated with IoT integration?
Challenges include ensuring data quality, managing cybersecurity risks, and training personnel to effectively use IoT systems.
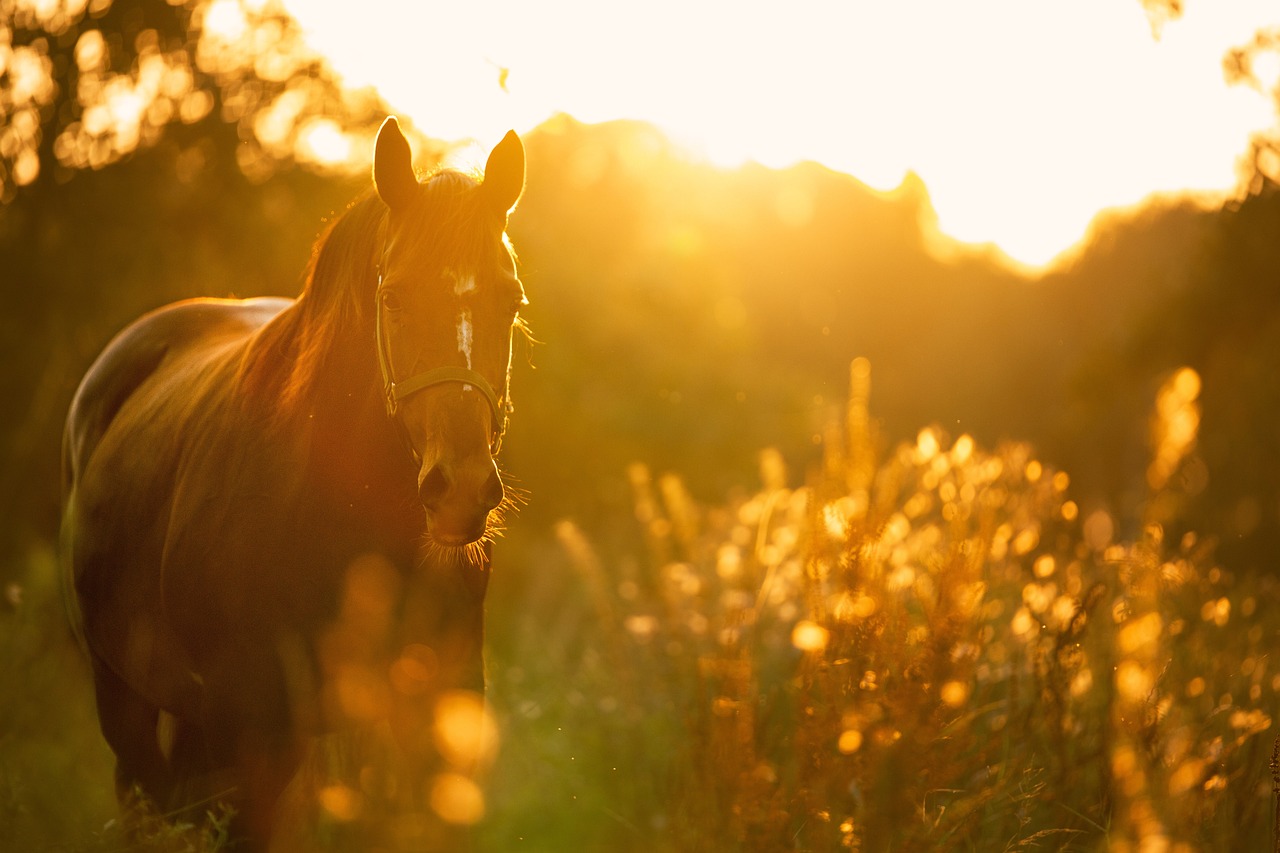
Sensor Technologies
In the realm of predictive maintenance, serve as the backbone of data collection, providing crucial insights into the operational status of machinery. These sensors act like the senses of a machine, constantly monitoring various parameters and sending real-time data that can be analyzed for potential issues. Imagine trying to diagnose a health problem without any symptoms to guide you; that's how predictive maintenance would function without sensors.
There are several types of sensors utilized in predictive maintenance, each designed to monitor specific conditions. For instance, vibration sensors are essential for detecting imbalances or misalignments in rotating machinery. When a machine vibrates more than usual, it can indicate wear and tear, allowing maintenance teams to take action before a catastrophic failure occurs. Similarly, temperature sensors monitor the heat levels of equipment. Overheating is often a sign of impending failure, and early detection can prevent costly breakdowns.
Another important category is acoustic sensors, which listen for sounds that may indicate mechanical issues. These sensors can pick up the subtle sounds of friction or grinding that might go unnoticed by human ears. By analyzing these sounds, predictive maintenance systems can identify problems long before they escalate. The integration of these various sensor technologies creates a comprehensive monitoring system that can significantly enhance maintenance strategies.
Moreover, the data collected from these sensors can be visualized and analyzed through advanced AI algorithms, which helps in identifying patterns and predicting failures with greater accuracy. To illustrate, consider the following table that summarizes key sensor types and their applications:
Sensor Type | Application | Benefits |
---|---|---|
Vibration Sensors | Detect imbalances in rotating equipment | Prevents unexpected failures by identifying wear |
Temperature Sensors | Monitor heat levels in machinery | Prevents overheating and associated damage |
Acoustic Sensors | Listen for unusual sounds in machinery | Identifies mechanical issues before they escalate |
In conclusion, sensor technologies are indispensable in the predictive maintenance landscape. They not only provide the data necessary for effective analysis but also empower organizations to proactively address equipment issues, ultimately leading to improved operational efficiency and reduced costs. As technology continues to advance, the role of these sensors will only become more critical, paving the way for smarter maintenance strategies.
- What types of sensors are most commonly used in predictive maintenance?
The most common types include vibration sensors, temperature sensors, and acoustic sensors, each serving a unique purpose in monitoring equipment health.
- How does AI enhance the effectiveness of sensor data?
AI algorithms analyze the data collected from sensors to identify patterns and predict potential failures, allowing for timely interventions.
- Can predictive maintenance reduce operational costs?
Absolutely! By preventing unexpected breakdowns, organizations can save significantly on repair costs and downtime.
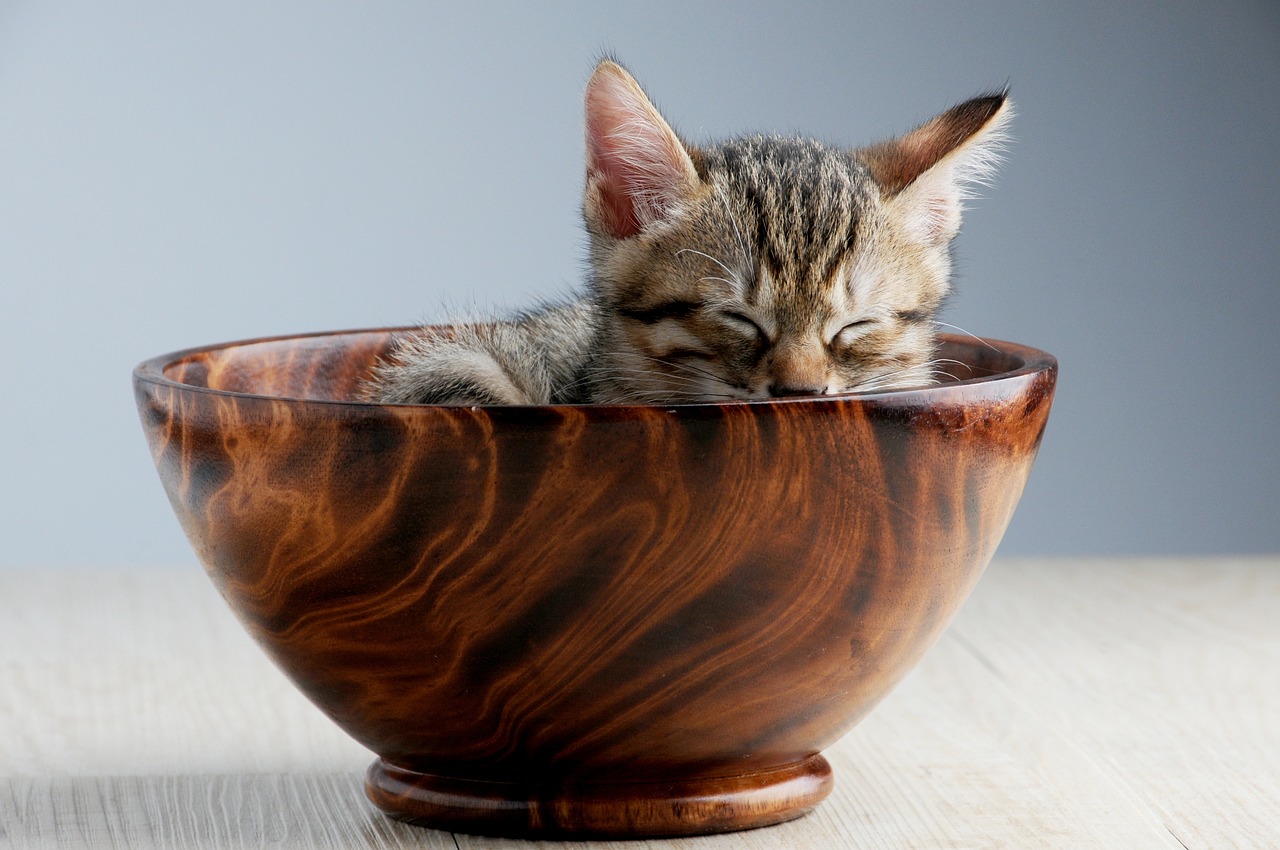
AI Algorithms in Predictive Maintenance
When it comes to predictive maintenance, AI algorithms are the unsung heroes that help transform raw data into actionable insights. These algorithms are designed to analyze vast amounts of data quickly and accurately, identifying patterns that might be invisible to the human eye. Imagine having a super-smart assistant that can sift through mountains of information, pinpointing potential issues before they escalate into costly failures. That’s precisely what these AI algorithms do!
There are several types of AI algorithms commonly employed in predictive maintenance, each with its unique strengths. For instance, machine learning algorithms can learn from historical data and improve their predictions over time. They analyze past equipment performance, maintenance records, and operational conditions to forecast when a machine is likely to fail. This process is akin to teaching a child to recognize patterns; the more examples they see, the better they get at predicting what comes next.
Another powerful tool in the AI arsenal is deep learning. This subset of machine learning mimics the way our brains process information, using neural networks to analyze complex datasets. Deep learning is particularly effective in identifying intricate patterns in data, such as subtle changes in vibration or temperature that could indicate an impending failure. It’s like having a highly trained detective who can notice the tiniest clues that others might overlook.
To illustrate how these algorithms work in practice, let’s consider a hypothetical example involving a manufacturing facility. The facility uses a variety of machines, each equipped with sensors that monitor performance metrics such as temperature, vibration, and operational speed. The data collected from these sensors feeds into machine learning algorithms, which analyze the information to predict when a machine might need maintenance. The outcome? The facility can schedule maintenance during planned downtimes, which minimizes disruptions and maximizes productivity.
Moreover, AI algorithms can also be categorized based on their approach to data analysis. Some may use supervised learning, where the algorithm is trained on labeled datasets, while others might employ unsupervised learning, which looks for hidden patterns without prior labels. This versatility allows organizations to select the right approach based on their specific needs and the type of data they have at their disposal.
In summary, the role of AI algorithms in predictive maintenance cannot be overstated. They not only enhance the accuracy of predictions but also empower organizations to make informed decisions that lead to significant cost savings and improved operational efficiency. By leveraging these advanced technologies, companies can stay one step ahead of potential failures, ensuring that their operations run smoothly and efficiently.
- What is predictive maintenance?
Predictive maintenance is a proactive maintenance strategy that uses data analysis tools to predict equipment failures before they occur, minimizing unplanned downtime. - How does AI improve predictive maintenance?
AI enhances predictive maintenance by analyzing large datasets quickly to provide accurate predictions, which leads to better decision-making and improved maintenance strategies. - What types of AI algorithms are used in predictive maintenance?
Common AI algorithms used include machine learning and deep learning, which analyze data patterns to predict potential equipment failures. - What challenges might organizations face when implementing AI for predictive maintenance?
Organizations may encounter issues such as data quality, integration complexities, and the need for skilled personnel to manage AI systems.
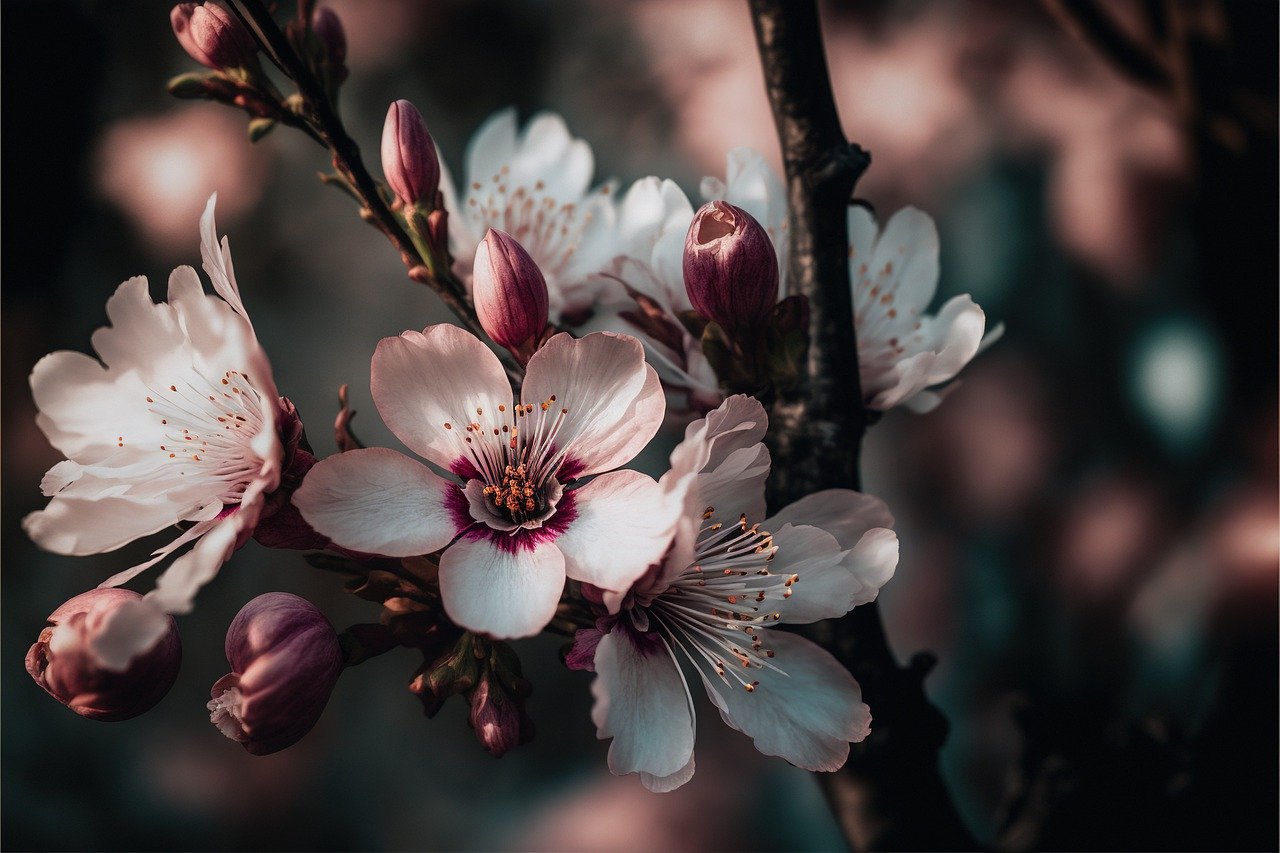
Benefits of AI-Driven Predictive Maintenance
AI-driven predictive maintenance is revolutionizing the way industries approach equipment upkeep and management. By leveraging advanced algorithms and real-time data analysis, organizations can unlock a treasure trove of benefits that not only enhance operational efficiency but also contribute to substantial cost savings. Imagine a world where machines tell you when they're about to fail—sounds like science fiction, right? But that's the power of AI in predictive maintenance!
One of the most significant advantages of implementing AI in predictive maintenance is the reduction in maintenance costs. Traditional maintenance strategies often rely on scheduled maintenance, which can lead to unnecessary downtime and wasted resources. In contrast, AI enables organizations to anticipate equipment failures before they occur, allowing for timely interventions. This proactive approach means that companies can avoid the hefty expenses associated with unexpected breakdowns and emergency repairs. For instance, a manufacturing plant that previously faced frequent machinery failures might see a drastic reduction in repair costs after adopting AI-driven strategies.
Moreover, AI enhances asset reliability. With continuous monitoring and analysis, businesses can ensure that their equipment operates at optimal levels, minimizing the risk of failure. This leads to improved overall performance and productivity. For example, in industries such as aviation and manufacturing, where precision and reliability are paramount, AI-driven predictive maintenance can significantly reduce the likelihood of operational disruptions.
Another noteworthy benefit is the enhanced lifespan of machinery. By ensuring that equipment is serviced at the right time and under the right conditions, organizations can extend the operational life of their assets. This not only translates to cost savings but also reduces the environmental impact associated with manufacturing and disposing of machinery. In a world increasingly focused on sustainability, this aspect of AI-driven predictive maintenance cannot be overlooked.
To further illustrate the benefits of AI-driven predictive maintenance, consider the following table that summarizes key advantages:
Benefit | Description |
---|---|
Cost Reduction | Minimizes unexpected breakdowns and associated repair costs. |
Asset Reliability | Ensures equipment operates optimally, reducing the risk of failures. |
Improved Equipment Lifespan | Extends the life of machinery through timely maintenance interventions. |
Data-Driven Decisions | Enhances decision-making processes with actionable insights from data. |
In conclusion, the integration of AI in predictive maintenance is not just a trend; it’s a game-changer for industries looking to optimize their operations. By embracing these technologies, organizations can unlock a wealth of benefits that lead to improved efficiency, reduced costs, and a more sustainable approach to equipment management. So, why wait? The future of maintenance is here, and it’s driven by AI!
- What is predictive maintenance? Predictive maintenance refers to the use of data analysis tools to predict equipment failures before they happen, allowing for proactive maintenance.
- How does AI improve predictive maintenance? AI enhances predictive maintenance by analyzing large datasets quickly and accurately, leading to better decision-making and maintenance strategies.
- What types of data are collected for predictive maintenance? Data is collected through sensors and IoT devices, capturing real-time information on equipment performance.
- What challenges do organizations face when implementing AI for predictive maintenance? Challenges include data quality issues, integration complexities with existing systems, and the need for skilled personnel to manage AI technologies.
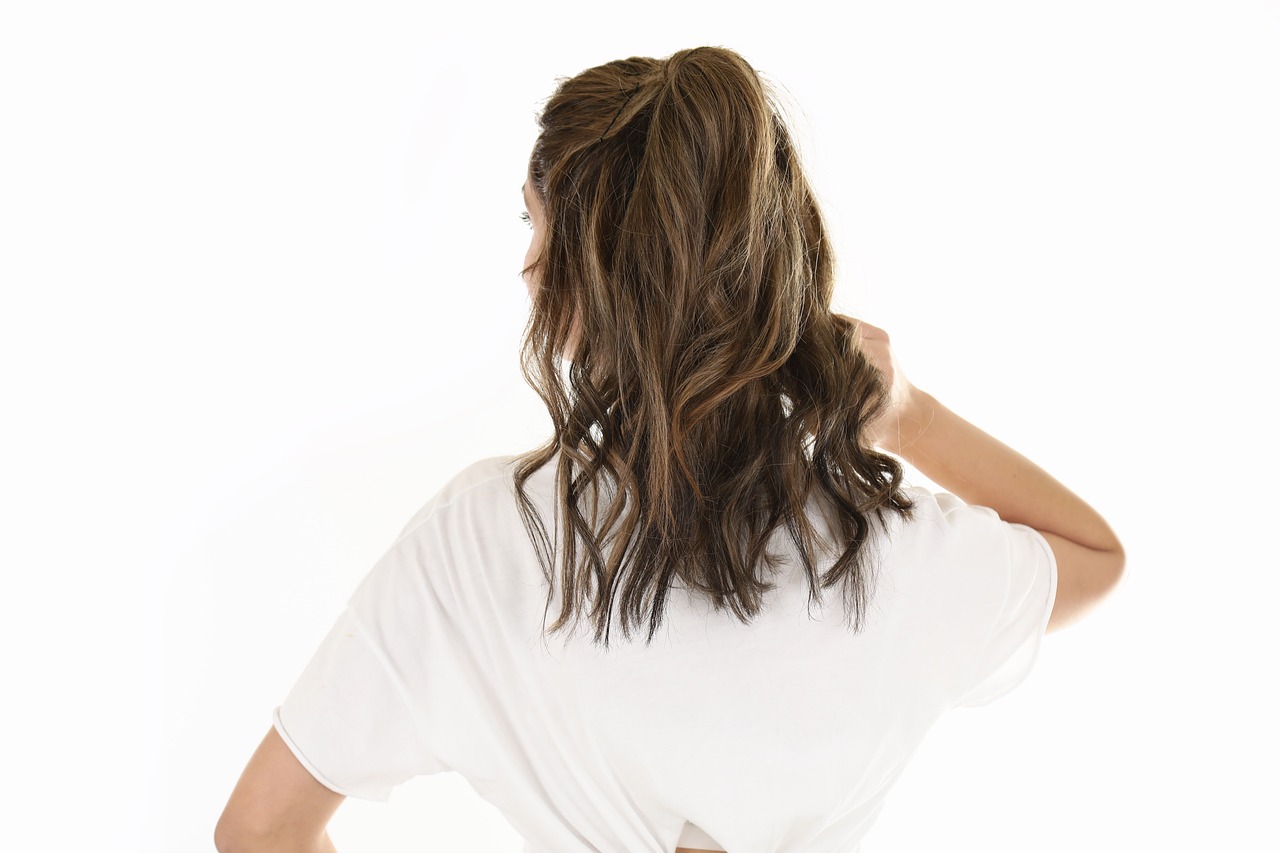
Cost Reduction
When it comes to running a business, every penny counts, right? That's where AI-driven predictive maintenance steps in like a superhero, swooping in to save the day—and your budget. By predicting equipment failures before they happen, organizations can significantly cut down on maintenance costs associated with unexpected breakdowns and repairs. Think about it: if you could foresee a problem with your machinery, wouldn't you want to fix it before it spirals into a costly disaster?
This proactive approach means less time spent on emergency repairs and more time focusing on what really matters—keeping your operations running smoothly. For instance, instead of scrambling to find a technician on a Friday night because a critical piece of equipment failed, you could schedule maintenance at your convenience. This shift not only saves money but also enhances the overall productivity of your workforce.
Moreover, the cost benefits extend beyond just immediate repairs. Consider the long-term implications of having a well-maintained machine. With AI, companies can analyze historical data and identify patterns that lead to equipment failure. By addressing these issues proactively, businesses can reduce the frequency of repairs and extend the lifespan of their assets. In fact, a study by the American Society of Mechanical Engineers found that predictive maintenance can reduce maintenance costs by up to 30%!
To illustrate this point further, let's take a look at a hypothetical scenario involving a manufacturing plant. Imagine a plant that relies on several critical machines to keep production flowing. Without predictive maintenance, a single failure could halt production for hours or even days, leading to lost revenue and increased labor costs. However, with AI-driven predictive maintenance, the plant can schedule maintenance during off-hours, ensuring that production remains uninterrupted. The table below summarizes the potential cost savings:
Scenario | Cost Without Predictive Maintenance | Cost With Predictive Maintenance |
---|---|---|
Emergency Repairs | $10,000 | $3,000 |
Downtime Losses | $20,000 | $5,000 |
Total Cost | $30,000 | $8,000 |
As you can see, the difference is staggering! By implementing AI-driven predictive maintenance, the plant could save a whopping $22,000 in a single incident. This is just one example, but it highlights how predictive maintenance can transform the financial landscape of any organization.
In conclusion, embracing AI for predictive maintenance is not just a smart move; it's a game-changer. By reducing maintenance costs, improving asset reliability, and enhancing operational efficiency, businesses can not only survive but thrive in today's competitive market. So, if you haven't already, it's time to consider how AI can help your organization cut costs and boost productivity!
- What is predictive maintenance?
Predictive maintenance is a proactive approach that uses data analysis tools to predict equipment failures before they occur, minimizing unplanned downtime. - How does AI enhance predictive maintenance?
AI enhances predictive maintenance by analyzing large datasets quickly, improving the accuracy of predictions and decision-making processes. - What are the benefits of AI-driven predictive maintenance?
Benefits include reduced maintenance costs, improved asset reliability, and enhanced operational efficiency. - What challenges do organizations face in implementing AI for predictive maintenance?
Challenges include data quality issues, integration complexities with existing systems, and the need for skilled personnel.
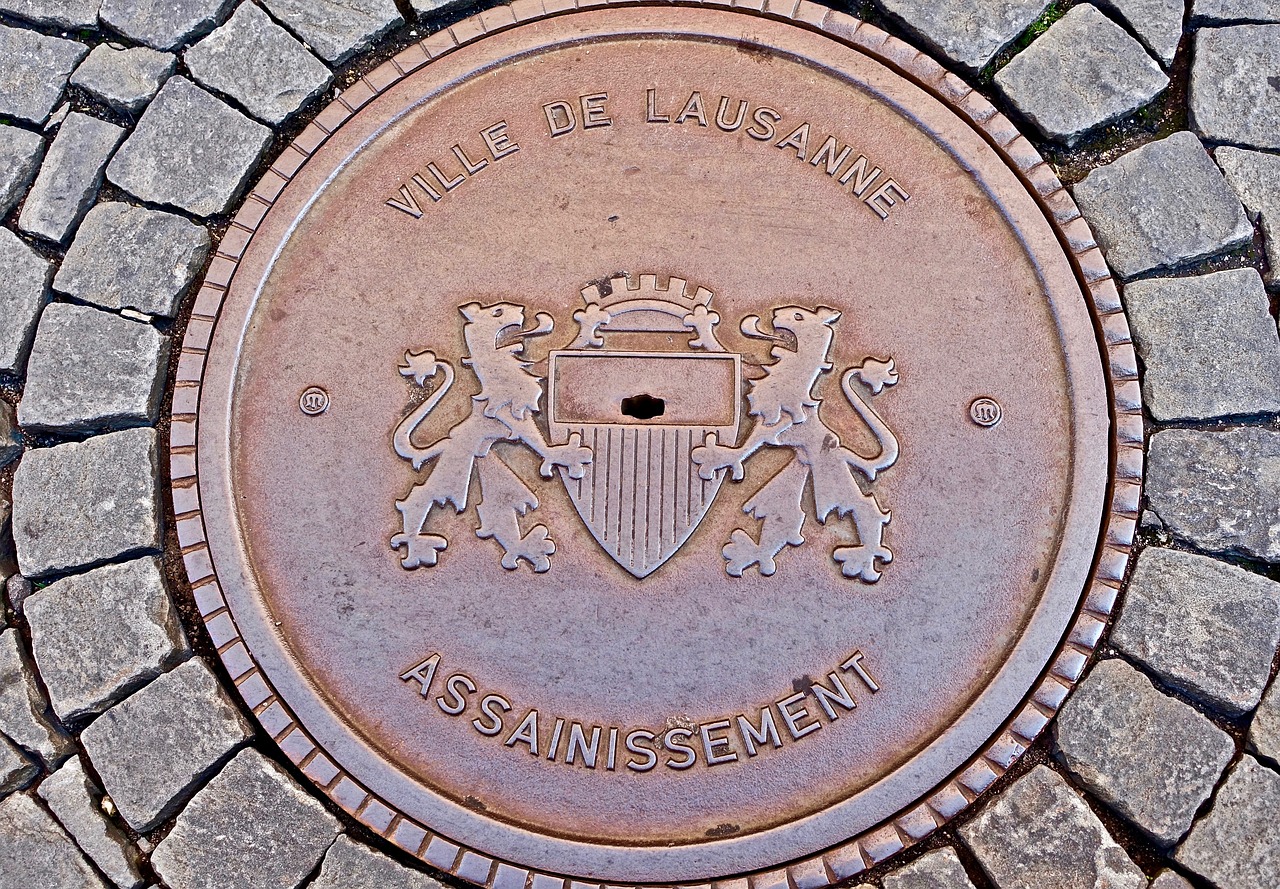
Improved Equipment Lifespan
In today's fast-paced industrial landscape, ensuring that machinery operates at peak performance is not just a goal; it's a necessity. One of the most significant advantages of AI-driven predictive maintenance is its ability to enhance the lifespan of equipment. Imagine your machinery as a finely-tuned sports car. Just as a sports car requires regular maintenance to perform at its best, so too does industrial equipment. Predictive maintenance acts as that regular check-up, ensuring everything runs smoothly and efficiently.
By leveraging AI technologies, organizations can monitor the health of their equipment continuously. This real-time oversight allows for timely interventions that prevent minor issues from escalating into major failures. For example, consider a manufacturing plant where a critical piece of machinery experiences a slight vibration. In the past, this might have gone unnoticed until it caused a catastrophic failure, leading to expensive repairs and extended downtime. However, with predictive maintenance powered by AI, that vibration can be detected early, prompting maintenance before a breakdown occurs.
Furthermore, the integration of sensor technologies plays a crucial role in this process. Various sensors, such as vibration, temperature, and acoustic sensors, collect data that AI algorithms analyze to identify patterns and anomalies. This data-driven approach not only helps in predicting failures but also optimizes operating conditions. When equipment operates under optimal conditions, it experiences less wear and tear, ultimately leading to a longer lifespan.
To illustrate the impact of AI on equipment longevity, consider the following benefits:
- Timely Maintenance: Regularly scheduled maintenance based on AI predictions helps in addressing issues before they escalate.
- Optimal Operating Conditions: AI ensures that machines operate within their ideal parameters, reducing stress on components.
- Data-Driven Insights: Continuous data collection and analysis provide insights that inform better maintenance practices.
In conclusion, embracing AI in predictive maintenance is akin to investing in a high-quality insurance policy for your equipment. It not only safeguards your assets from unexpected failures but also extends their operational life. As industries continue to evolve, the emphasis on maintaining equipment longevity through advanced technologies will only grow stronger. Organizations that recognize and act on this will undoubtedly enjoy a competitive edge in their respective markets.
- What is predictive maintenance? Predictive maintenance is a proactive maintenance strategy that uses data analysis to predict equipment failures before they occur.
- How does AI improve predictive maintenance? AI enhances predictive maintenance by quickly analyzing large datasets for patterns, leading to more accurate failure predictions.
- What types of sensors are used in predictive maintenance? Common sensors include vibration, temperature, and acoustic sensors, which gather essential data from machinery.
- What are the benefits of AI-driven predictive maintenance? Benefits include reduced maintenance costs, improved asset reliability, and enhanced operational efficiency.
- What challenges do organizations face when implementing AI? Challenges include data quality issues, integration complexities, and the need for skilled personnel.
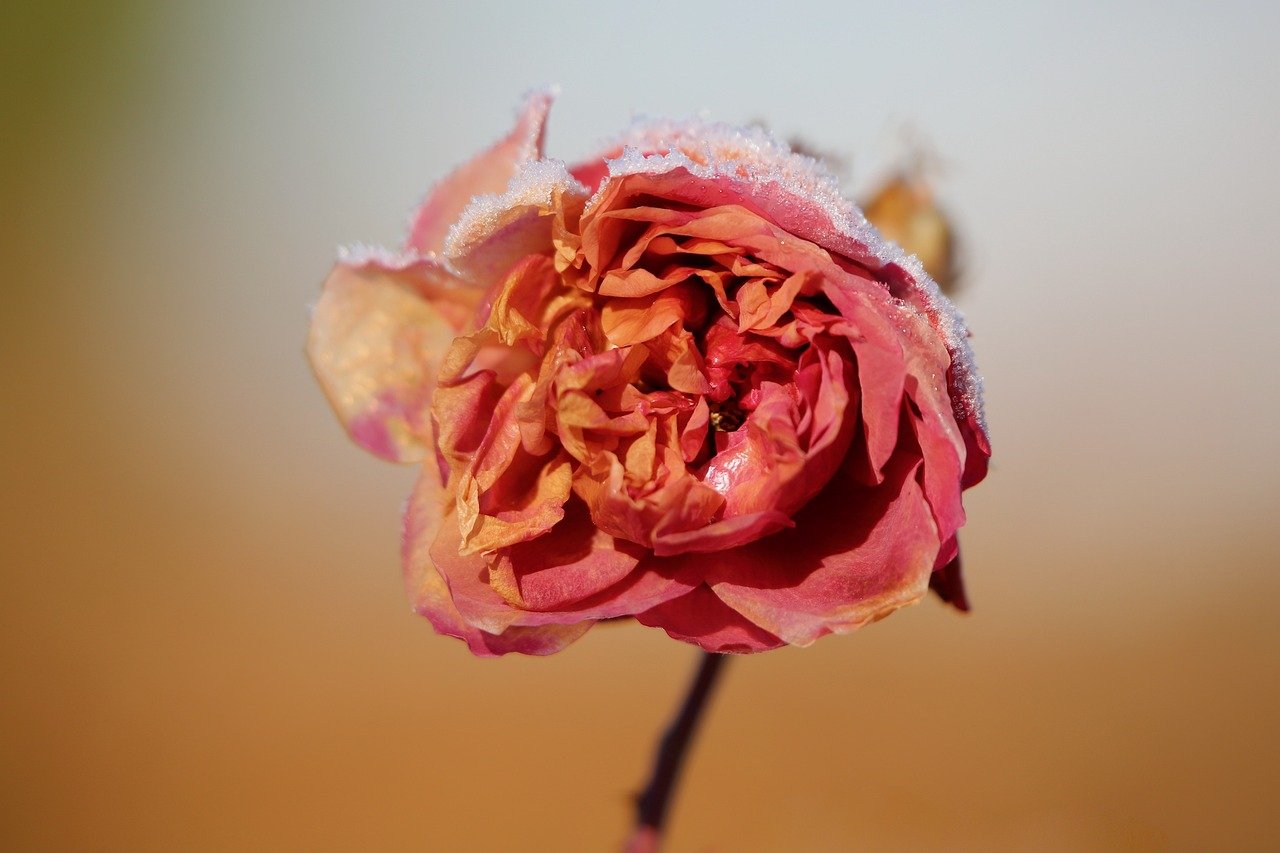
Challenges in Implementing AI for Predictive Maintenance
While the promise of AI in predictive maintenance is incredibly exciting, it's not all smooth sailing. Organizations often encounter a variety of challenges that can hinder the successful implementation of these advanced technologies. One of the primary issues is data quality. For AI algorithms to function effectively, they need access to high-quality, consistent data. Unfortunately, many organizations struggle with incomplete or noisy data, which can lead to inaccurate predictions and ultimately undermine the entire predictive maintenance strategy.
Another significant hurdle is the integration with existing systems. Many companies still rely on legacy systems that may not be compatible with modern AI solutions. This integration process can be complex and often requires substantial investment in both technology and training. Imagine trying to fit a square peg into a round hole; that’s what it can feel like when attempting to merge old systems with new AI capabilities. Moreover, the need for skilled personnel to manage and interpret AI systems adds another layer of complexity. Organizations may find themselves in a tight spot, having to hire or train staff who are proficient in both AI technologies and the specific industry knowledge required for effective predictive maintenance.
Furthermore, organizations face cultural resistance when introducing AI technologies. Employees may be apprehensive about how AI will affect their roles, leading to pushback against new systems. It’s essential to foster a culture of openness and education surrounding AI, so everyone understands that the goal is not to replace jobs but to enhance operational efficiency. To address these challenges, companies must develop a comprehensive strategy that includes:
- Investing in data management tools to ensure high-quality data collection.
- Creating a phased integration plan that allows for gradual adoption of AI technologies.
- Providing training and resources to employees to ease the transition into AI-driven processes.
In summary, while the road to implementing AI for predictive maintenance is filled with challenges, recognizing and addressing these issues head-on can pave the way for a successful and efficient predictive maintenance strategy. With the right approach, organizations can harness the power of AI to revolutionize their maintenance practices and achieve significant operational improvements.
Q: What is predictive maintenance?
A: Predictive maintenance is a proactive maintenance strategy that uses data analysis tools to predict equipment failures before they occur, thereby minimizing unplanned downtime and extending the lifespan of machinery.
Q: How does AI enhance predictive maintenance?
A: AI enhances predictive maintenance by analyzing large datasets quickly and accurately, leading to better decision-making and improved maintenance strategies.
Q: What are the main challenges in implementing AI for predictive maintenance?
A: The main challenges include data quality issues, integration with existing systems, the need for skilled personnel, and cultural resistance to change.
Q: What are some benefits of AI-driven predictive maintenance?
A: Benefits include reduced maintenance costs, improved asset reliability, and enhanced operational efficiency across various industries.
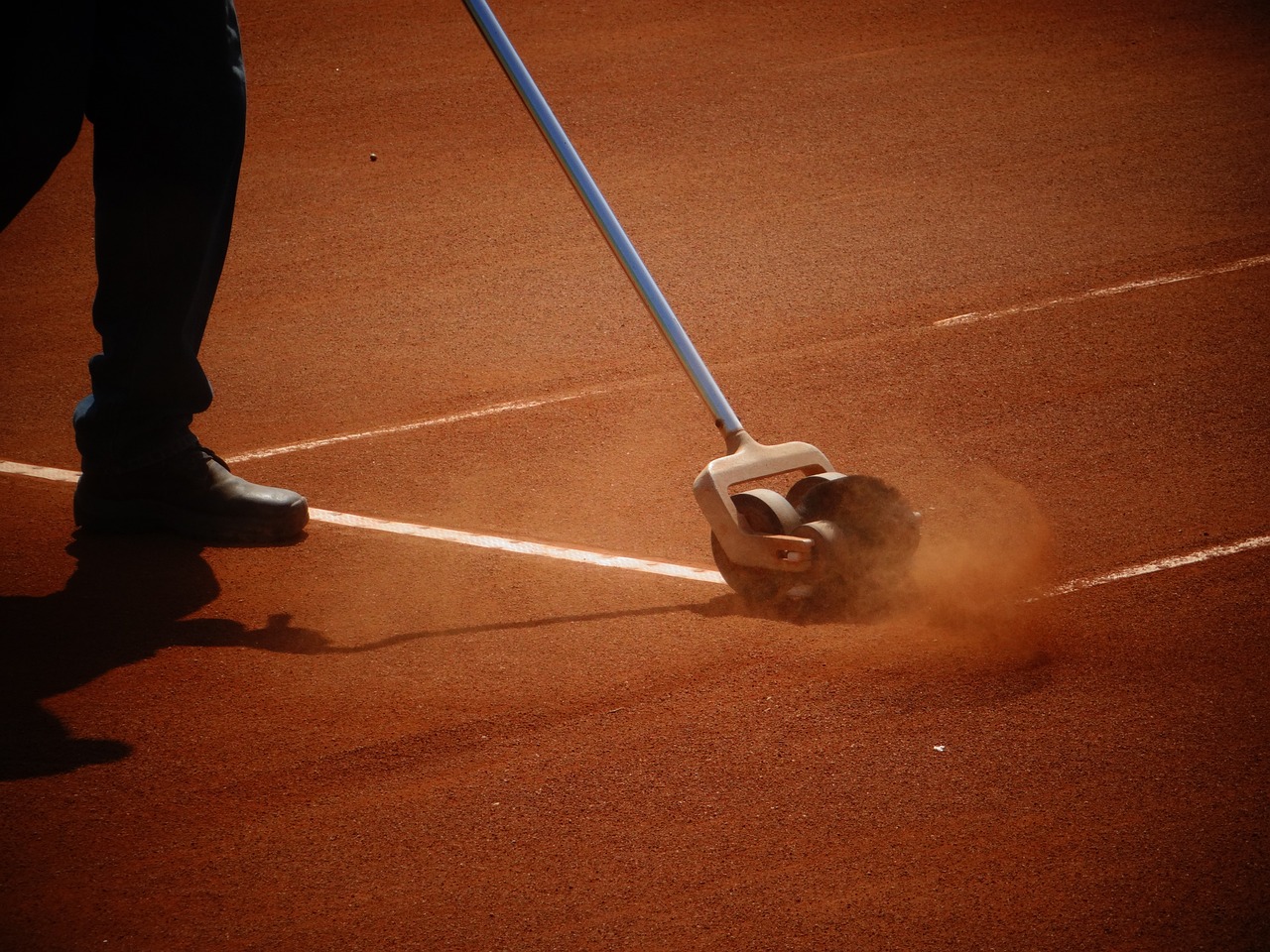
Data Quality Issues
When it comes to implementing AI for predictive maintenance, one of the most critical challenges organizations face is . Imagine trying to solve a puzzle with missing pieces; that’s exactly what companies are up against when they deal with inconsistent or incomplete data. High-quality data is the backbone of effective predictive maintenance strategies. Without it, the accuracy of AI predictions can be severely compromised, leading to misguided maintenance decisions and potentially costly downtime.
To put it simply, if the data fed into AI algorithms is flawed, the outcomes will be flawed as well. Organizations often struggle with a variety of data quality issues, including:
- Inconsistent Data: Variations in data formats and structures can create confusion and hinder effective analysis.
- Incomplete Data: Missing data points can lead to skewed predictions, as AI models rely on comprehensive datasets to learn and make informed decisions.
- Outdated Data: Using stale information can result in predictions that do not reflect current conditions, making maintenance strategies ineffective.
Addressing these data quality issues requires a multi-faceted approach. Organizations must invest in robust data governance frameworks to ensure that data is collected, stored, and processed consistently. This involves not only the integration of advanced data management tools but also the establishment of best practices for data entry and maintenance. Moreover, training personnel to understand the importance of data quality can foster a culture that prioritizes accurate and reliable information.
Furthermore, organizations can benefit from implementing data cleansing techniques to enhance the quality of their datasets. This might include regular audits, validation checks, and the use of machine learning algorithms to identify anomalies in the data. By focusing on data quality, companies can significantly improve the effectiveness of their predictive maintenance strategies, ultimately leading to better operational efficiency and reduced costs.
- What is predictive maintenance? Predictive maintenance involves using data analysis tools to predict equipment failures before they occur, minimizing unplanned downtime.
- Why is data quality important in predictive maintenance? High-quality data is essential for accurate predictions; poor data can lead to ineffective maintenance strategies and increased costs.
- How can organizations improve data quality? Organizations can improve data quality by implementing robust data governance frameworks, conducting regular audits, and training personnel on data management best practices.
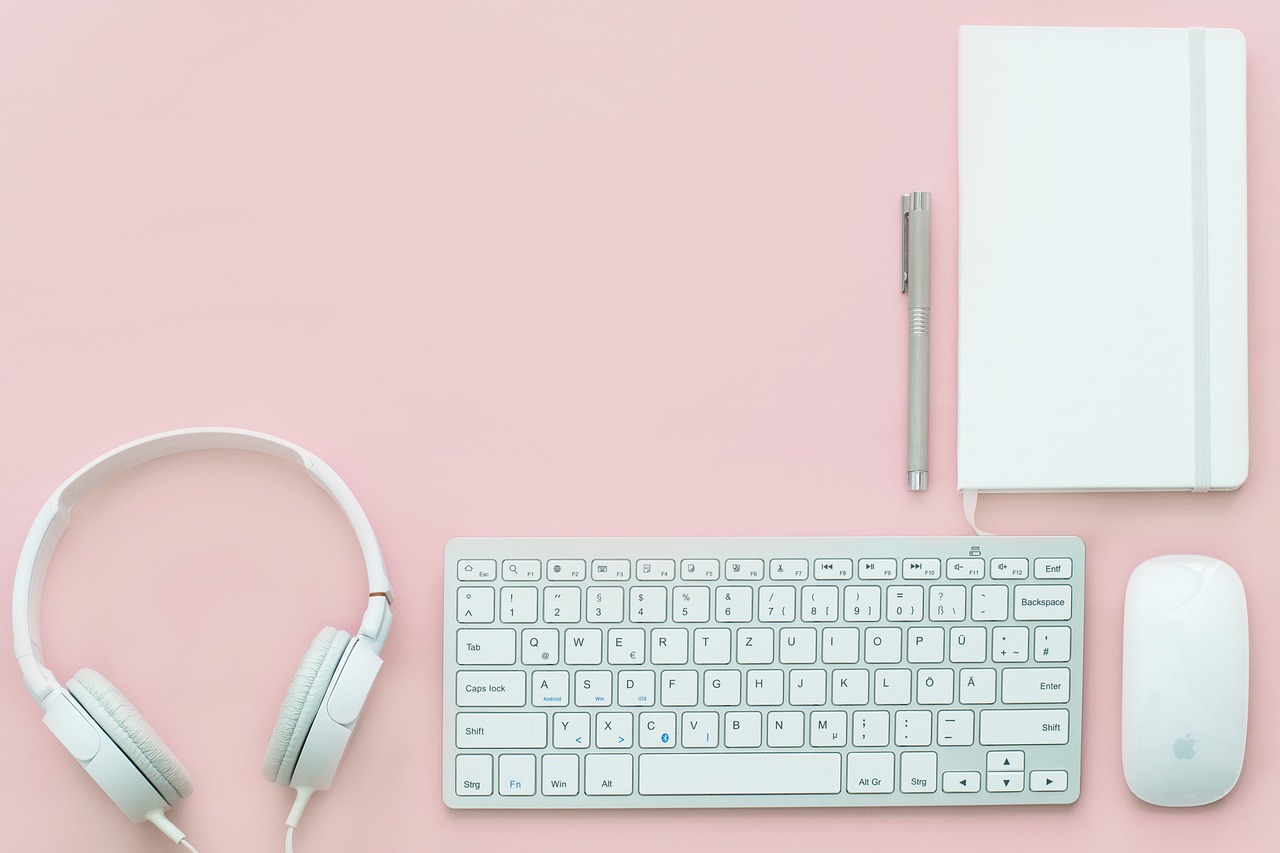
Integration with Existing Systems
Integrating artificial intelligence (AI) solutions with existing systems can be a daunting task for many organizations. Imagine trying to fit a square peg into a round hole; that’s often how it feels when businesses aim to incorporate advanced AI technologies into their legacy systems. These older systems, while reliable, may not be designed to communicate with modern AI tools, creating a significant barrier to seamless integration.
One of the primary challenges lies in the data compatibility between old and new systems. Legacy systems often store data in formats that are not easily accessible or analyzable by AI algorithms. This can lead to a frustrating situation where valuable data remains trapped, unable to contribute to predictive maintenance strategies. To overcome this, organizations may need to invest in data transformation tools that can convert legacy data into a more usable format.
Moreover, the integration process can be complex and time-consuming. It requires a thorough understanding of both the existing systems and the new AI technologies. Teams may need to undergo extensive training to ensure they can effectively manage and operate the integrated systems. This often leads to increased costs and resource allocation, which can be a significant hurdle for smaller organizations.
Despite these challenges, the benefits of successfully integrating AI into existing systems can be transformative. Organizations can achieve:
- Enhanced Efficiency: Automated processes can streamline operations and reduce manual errors.
- Improved Decision-Making: With AI analyzing data in real-time, businesses can make informed decisions faster.
- Cost Savings: By reducing downtime and maintenance costs, the initial investment in integration can pay off significantly.
In conclusion, while integrating AI solutions with existing systems presents challenges, it is a crucial step towards achieving effective predictive maintenance. Organizations must be prepared to invest time, resources, and training to ensure a smooth transition. By doing so, they can unlock the full potential of AI technologies, leading to improved operational efficiency and reduced costs.
- What is predictive maintenance? Predictive maintenance involves using data analysis tools to predict equipment failures before they occur, minimizing unplanned downtime.
- How does AI enhance predictive maintenance? AI analyzes large datasets quickly to provide accurate predictions, improving decision-making and maintenance strategies.
- What are the main challenges in integrating AI? The main challenges include data compatibility issues, the complexity of integration, and the need for skilled personnel.
- What benefits can organizations expect from AI-driven predictive maintenance? Organizations can expect reduced maintenance costs, improved asset reliability, and enhanced operational efficiency.
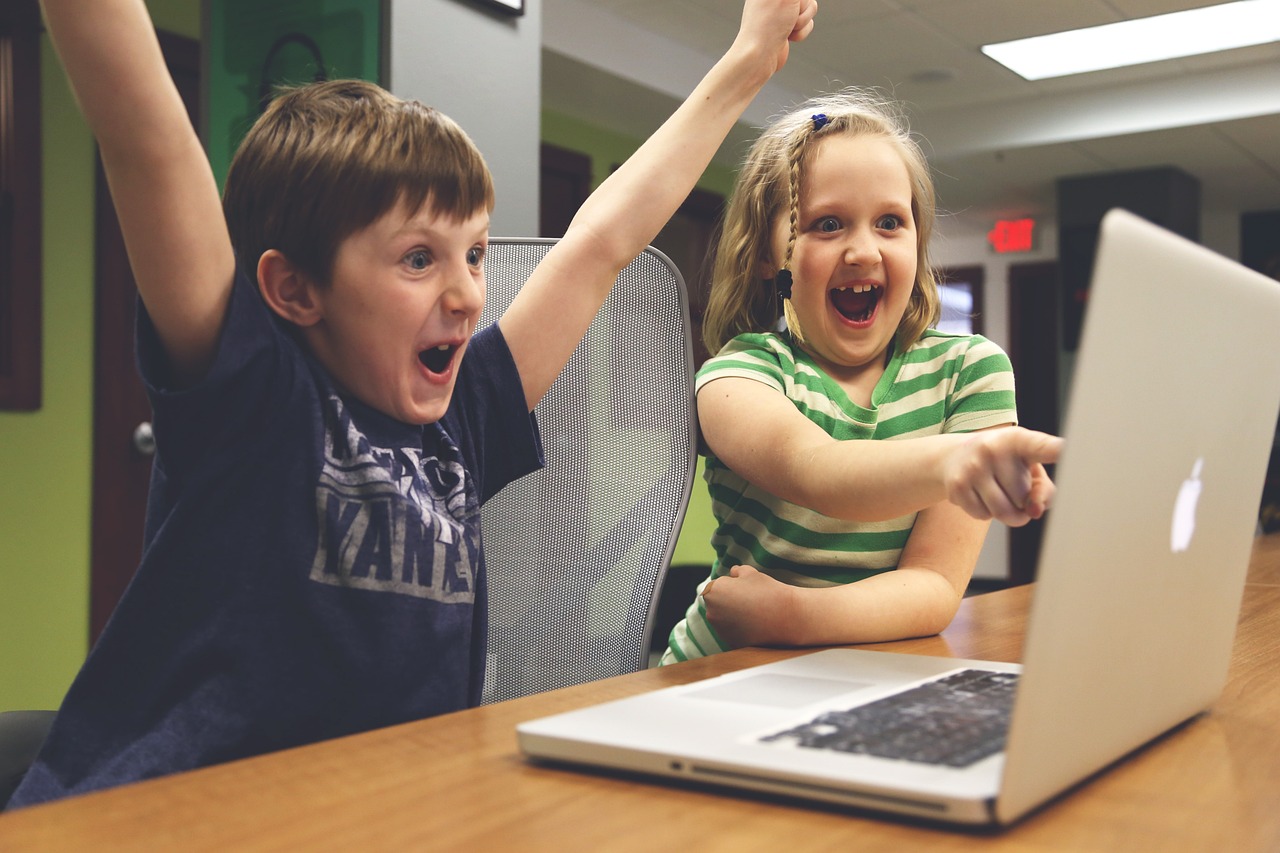
Future Trends in AI and Predictive Maintenance
The future of AI in predictive maintenance is not just bright; it's practically dazzling! As technology continues to evolve, we can expect a wave of innovations that will revolutionize how industries approach maintenance strategies. One of the most exciting trends is the advancement in machine learning. With emerging techniques, machine learning is set to enhance predictive maintenance capabilities significantly. This means that algorithms will become more sophisticated, allowing for deeper analysis of data patterns and improved accuracy in predicting equipment failures. Imagine a world where machines can not only predict their own failures but also suggest the best course of action to prevent them!
Moreover, increased automation is on the horizon, which will further transform the landscape of predictive maintenance. As automation technology advances, we will see more autonomous systems that can self-diagnose issues and even resolve them without human intervention. This leap forward is akin to having a personal mechanic for every machine, tirelessly working in the background to ensure everything runs smoothly. The integration of AI will facilitate these systems to operate more efficiently, leading to reduced downtime and increased productivity.
Another key trend is the growing importance of data analytics. With the explosion of data generated by IoT devices and sensors, organizations will need to harness this information effectively. Advanced analytics tools will empower companies to extract actionable insights from their data, enabling them to make informed decisions about maintenance schedules and resource allocation. This shift towards a data-driven approach will not only enhance operational efficiency but also foster a culture of continuous improvement within organizations.
To give you a clearer picture of how these trends will shape the future, let’s look at the potential benefits:
Trend | Potential Benefits |
---|---|
Advancements in Machine Learning | Improved predictive accuracy, reduced false positives, and enhanced decision-making. |
Increased Automation | Lower maintenance costs, reduced downtime, and improved operational efficiency. |
Enhanced Data Analytics | Better insights, proactive maintenance strategies, and optimized resource allocation. |
In conclusion, the future trends in AI and predictive maintenance are set to create a paradigm shift in how businesses operate. By embracing these advancements, organizations can not only streamline their maintenance processes but also significantly enhance their overall performance. The question remains: are you ready to embrace the future of predictive maintenance?
- What is predictive maintenance?
Predictive maintenance is a proactive approach that uses data analysis to predict equipment failures before they occur, minimizing downtime and extending machinery lifespan. - How does AI enhance predictive maintenance?
AI enhances predictive maintenance by analyzing large datasets quickly, improving prediction accuracy, and enabling better decision-making. - What are the benefits of AI-driven predictive maintenance?
Benefits include reduced maintenance costs, improved asset reliability, and enhanced operational efficiency across various industries. - What challenges do organizations face when implementing AI?
Challenges include data quality issues, integration complexities with existing systems, and the need for skilled personnel to manage AI technologies.
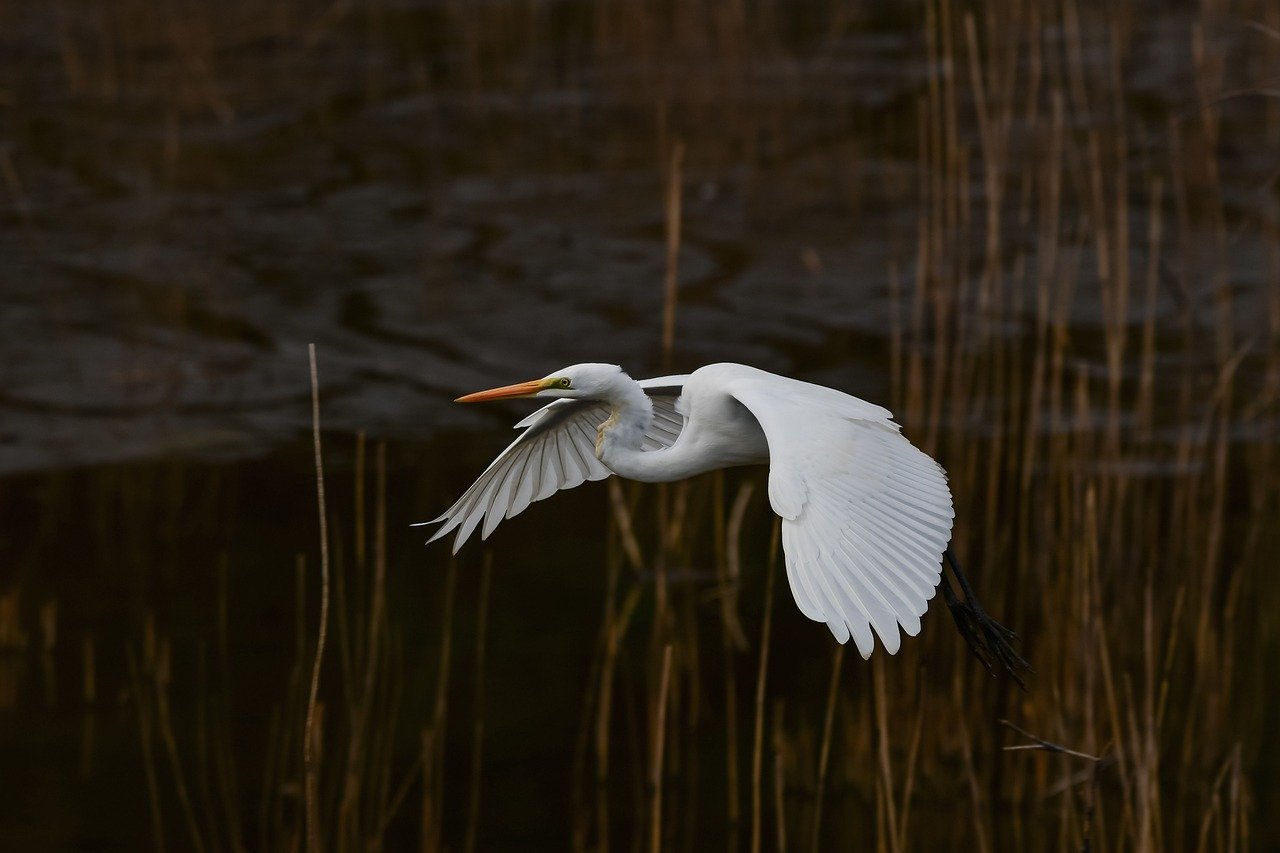
Advancements in Machine Learning
Machine learning is revolutionizing the landscape of predictive maintenance, offering a plethora of advancements that are reshaping how industries approach equipment management. Imagine a world where machines can not only diagnose their own issues but also predict future failures before they even occur. This isn’t just a pipe dream; it’s the reality that machine learning is making possible. By leveraging complex algorithms and vast datasets, machine learning models can identify subtle patterns and anomalies in equipment behavior that would be invisible to the human eye.
One significant advancement in machine learning is the development of self-learning algorithms. These algorithms continuously improve their predictive accuracy by learning from new data. As they process more information, they refine their predictions, making them increasingly reliable over time. This capability is crucial in predictive maintenance, where the cost of unplanned downtime can be astronomical. For instance, a manufacturing plant might experience losses of thousands of dollars per hour due to equipment failure. By implementing self-learning algorithms, these plants can drastically reduce the risk of such failures.
Moreover, the integration of deep learning techniques has opened new avenues for analyzing complex datasets. Deep learning, a subset of machine learning, utilizes neural networks with many layers to process data in a way that mimics human thought processes. This allows for the analysis of unstructured data, such as images and sound waves, which are often overlooked in traditional predictive maintenance strategies. For example, using deep learning, a company can analyze vibration data from machinery to detect irregular patterns that indicate potential failure, all while eliminating the noise that can lead to false alarms.
Another exciting development is the use of predictive analytics powered by machine learning. Predictive analytics combines historical data with real-time information to forecast future events. In the context of predictive maintenance, this means that organizations can anticipate when a machine is likely to fail based on its past performance and current condition. The result? Organizations can schedule maintenance during planned downtimes, minimizing disruptions and maximizing productivity.
To illustrate the impact of these advancements, consider the following table that summarizes key machine learning techniques and their applications in predictive maintenance:
Machine Learning Technique | Application in Predictive Maintenance |
---|---|
Self-Learning Algorithms | Continuously improve predictions based on new data |
Deep Learning | Analyze unstructured data for complex pattern recognition |
Predictive Analytics | Forecast future equipment failures based on historical trends |
As we look to the future, the advancements in machine learning will continue to evolve, offering even more sophisticated tools for predictive maintenance. These innovations promise not only to enhance the accuracy of predictions but also to automate many aspects of maintenance management, allowing organizations to focus on their core operations rather than reactive repairs. With machine learning at the helm, the potential for improved efficiency and cost savings is boundless, paving the way for a new era in industrial maintenance.
- What is predictive maintenance? Predictive maintenance is a proactive maintenance strategy that uses data analysis tools to predict equipment failures before they occur, minimizing downtime and extending machinery lifespan.
- How does AI enhance predictive maintenance? AI enhances predictive maintenance by analyzing large datasets quickly, allowing for more accurate predictions and better decision-making.
- What role do sensors play in predictive maintenance? Sensors collect real-time data from machinery, which is essential for effective predictive maintenance strategies.
- What are some challenges of implementing AI in predictive maintenance? Challenges include data quality issues, integration complexities with existing systems, and the need for skilled personnel to manage AI systems.
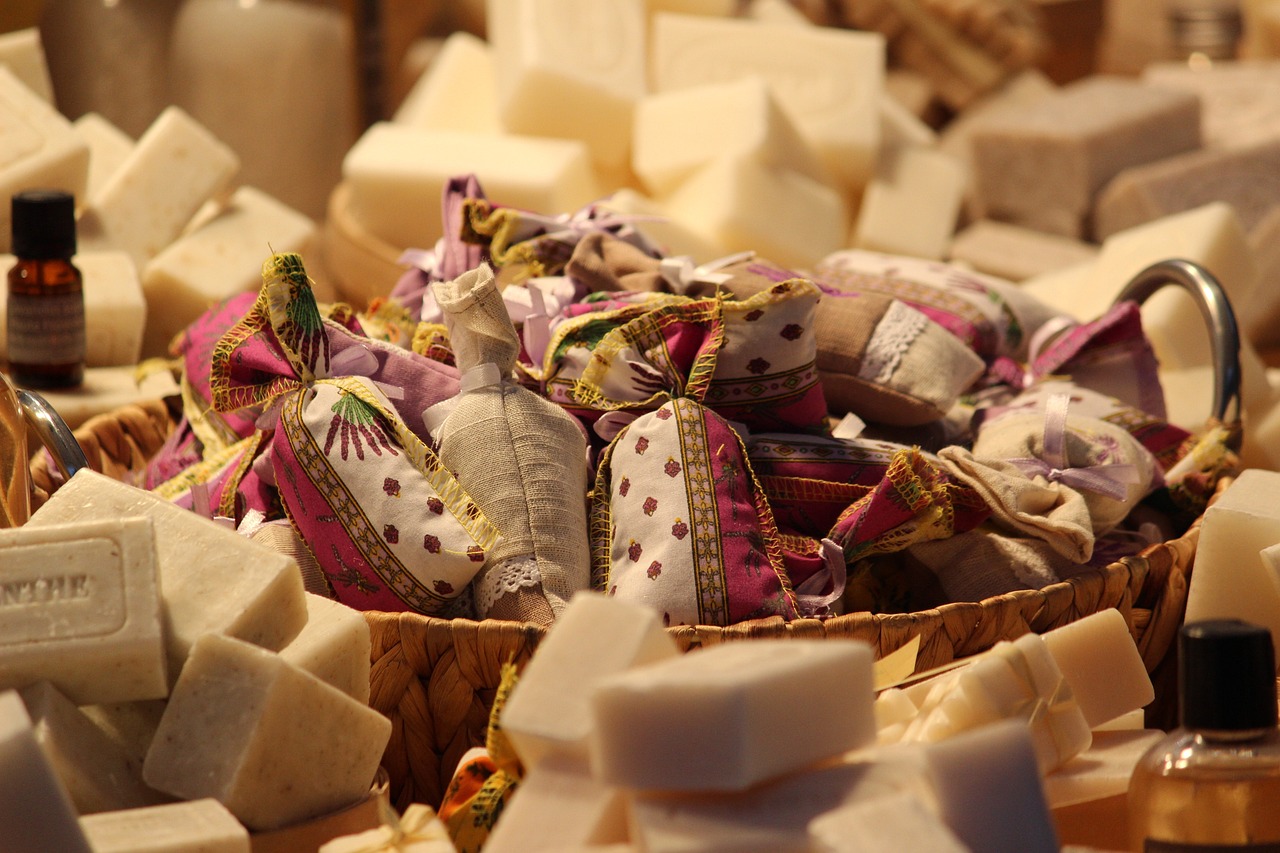
Increased Automation
As we venture deeper into the age of technology, is rapidly transforming the landscape of predictive maintenance. Imagine a world where machines not only report their own health but also autonomously diagnose and rectify issues without human intervention. This is not just a dream; it's becoming a reality thanks to the integration of artificial intelligence.
Automation in predictive maintenance means that systems can continuously monitor their performance and make decisions based on predefined algorithms. This capability leads to a significant reduction in human error and enhances operational efficiency. For instance, consider a manufacturing plant where machinery is equipped with AI-driven systems that can predict wear and tear. These systems can analyze vast amounts of data collected from various sensors, such as temperature, vibration, and pressure, to forecast potential failures.
Furthermore, the integration of automation in predictive maintenance offers several key advantages:
- Real-Time Monitoring: Automated systems can provide continuous insights into equipment performance, allowing for immediate action when abnormalities are detected.
- Self-Diagnosis: With advanced algorithms, machines can identify their own issues, reducing the need for manual inspections and increasing uptime.
- Predictive Alerts: Automation enables timely alerts for maintenance needs, ensuring that interventions occur before failures happen.
However, while the benefits are clear, implementing increased automation does come with its challenges. Organizations must invest in the right technologies and training to ensure that their workforce can effectively manage these advanced systems. Additionally, the transition from traditional maintenance practices to automated solutions requires a cultural shift within the organization.
In summary, the future of predictive maintenance is undeniably intertwined with increased automation. As technology continues to advance, we can expect machines to become even more intelligent, capable of not just predicting failures but also resolving them autonomously. This shift will not only enhance operational efficiency but also allow human workers to focus on more strategic tasks, ultimately driving innovation and growth in various industries.
- What is predictive maintenance?
Predictive maintenance is a proactive approach that utilizes data analysis to predict equipment failures before they occur, minimizing unplanned downtime. - How does AI enhance predictive maintenance?
AI enhances predictive maintenance by analyzing large datasets quickly, leading to more accurate predictions and improved decision-making. - What role do sensors play in predictive maintenance?
Sensors collect real-time data from machinery, which is crucial for informing predictive maintenance strategies. - What are the challenges of implementing AI in predictive maintenance?
Challenges include data quality issues, integration complexities, and the need for skilled personnel to manage AI systems.
Frequently Asked Questions
- What is predictive maintenance?
Predictive maintenance is a proactive approach that uses data analysis tools to predict equipment failures before they happen. By anticipating issues, organizations can minimize unplanned downtime and extend the lifespan of their machinery.
- How does AI enhance predictive maintenance?
AI enhances predictive maintenance by quickly analyzing large datasets to make more accurate predictions. This leads to improved decision-making and ultimately better maintenance strategies, ensuring that equipment runs smoothly and efficiently.
- What role do IoT devices play in predictive maintenance?
IoT devices are crucial for continuous monitoring of equipment performance. They gather real-time data that AI algorithms analyze, providing valuable insights that inform maintenance strategies and help predict potential failures.
- What types of sensors are used in predictive maintenance?
Various sensors such as vibration, temperature, and acoustic sensors are used to capture essential data. These sensors help detect anomalies in equipment performance, enabling timely interventions before failures occur.
- What are the benefits of AI-driven predictive maintenance?
AI-driven predictive maintenance offers several advantages, including reduced maintenance costs, improved asset reliability, and enhanced operational efficiency. By predicting failures, organizations can avoid unexpected breakdowns and ensure optimal performance.
- What challenges do organizations face when implementing AI for predictive maintenance?
Organizations often encounter challenges such as data quality issues, integration complexities with existing systems, and the need for skilled personnel to manage AI technologies. These hurdles can hinder the effectiveness of predictive maintenance strategies.
- What future trends can we expect in AI and predictive maintenance?
The future of predictive maintenance looks bright, with advancements in machine learning and increased automation. These trends will enhance predictive capabilities, allowing for more sophisticated analyses and the development of autonomous systems capable of self-diagnosing issues.