Deciphering the AI and Ethics Conundrum
The rise of artificial intelligence (AI) has ushered in a new era of innovation, but it also brings with it a myriad of ethical dilemmas that we cannot ignore. As we dive deeper into the world of AI, we find ourselves navigating through a complex web of moral questions, societal implications, and unforeseen consequences. How do we ensure that these powerful tools serve humanity positively and equitably? This article aims to unravel the intricate relationship between AI and ethics, shedding light on the challenges we face and exploring potential solutions that can help align AI development with our core moral values and societal norms.
At its core, the AI and ethics conundrum isn't merely a technical issue; it’s a philosophical one that requires us to reflect on what it means to be human in an age dominated by machines. As AI systems become increasingly integrated into our daily lives—ranging from autonomous vehicles to personal assistants—the stakes are higher than ever. We must ask ourselves: Are we prepared to trust these systems with our lives, our data, and our futures? The answers to these questions lie in understanding the ethical landscape surrounding AI.
In this exploration, we will delve into the foundational principles that guide AI development, such as fairness, accountability, and transparency. These principles are not just buzzwords; they are essential for ensuring that AI technologies are deployed responsibly. By examining the ethical implications of AI, we can better understand how to create systems that reflect our values and promote the greater good. So, buckle up as we navigate through the challenges and possibilities that lie ahead in the fascinating world of AI and ethics!
Understanding the ethical landscape surrounding AI involves examining the foundational principles that guide its development and deployment, including fairness, accountability, and transparency, which are crucial for responsible AI usage. Fairness ensures that AI systems treat all individuals equitably, while accountability holds developers and organizations responsible for the outcomes of their AI technologies. Transparency, on the other hand, allows users to understand how decisions are made, fostering trust in these systems.
As we navigate this ethical landscape, it's important to recognize that the challenges we face are not just technical but deeply human. The implications of AI stretch beyond algorithms and data; they touch on our values, our rights, and our very existence. How do we ensure that technology reflects the best of humanity rather than its worst? This question is at the heart of the ongoing discourse surrounding AI ethics.
Bias in AI algorithms poses significant ethical challenges, as it can lead to discrimination and inequality. This section delves into the sources of bias and strategies for mitigating its impact on AI systems. Imagine a world where a job application is filtered through an AI system that has been trained on biased data; the consequences could be devastating, perpetuating stereotypes and denying opportunities to deserving candidates.
Identifying sources of bias in data and algorithms is essential for creating fair AI systems. Common pitfalls include:
- Historical Bias: Data reflecting past prejudices can lead to biased outcomes.
- Sampling Bias: Non-representative data can skew results, affecting the system's fairness.
- Confirmation Bias: Algorithms may reinforce existing biases if not carefully monitored.
Recognizing these biases in AI development processes is crucial for creating systems that are truly equitable.
Data quality plays a pivotal role in AI performance and fairness. The importance of using diverse and representative datasets cannot be overstated. Poor quality data can lead to misleading conclusions and unfair treatment of certain groups. By prioritizing data quality, we can minimize bias in AI outcomes and foster a more inclusive technological landscape.
Algorithmic transparency is crucial for accountability in AI systems. Without clear explanations of how algorithms make decisions, users and stakeholders may be left in the dark. This lack of transparency can erode trust and lead to skepticism about AI technologies. Therefore, it’s essential to develop frameworks that promote transparency and allow users to understand the decision-making processes behind AI systems.
Mitigating bias in AI requires proactive strategies, including algorithm audits and inclusive design practices. Regular audits can help identify and rectify biases in algorithms before they cause harm. Furthermore, involving diverse teams in the design process can lead to more equitable AI solutions. By ensuring that multiple perspectives are considered, we can create AI systems that reflect the richness of human experience.
AI technologies often raise significant privacy concerns, particularly regarding data collection and usage. With the ability to analyze vast amounts of personal data, AI systems can inadvertently compromise individual privacy. This section explores the ethical implications of AI on individual privacy and potential regulatory measures that can safeguard our rights.
Data protection regulations, such as GDPR, play a crucial role in safeguarding privacy in AI applications. These regulations establish guidelines for how personal data should be handled, giving individuals more control over their information. Understanding the impact of these regulations on AI development is essential for ensuring that user rights are respected in an increasingly data-driven world.
Ethical data usage is essential for maintaining trust in AI systems. This involves not just compliance with regulations but also a commitment to responsible data handling practices. Informed consent must be prioritized, ensuring that individuals understand how their data will be used. By fostering a culture of ethical data usage, we can build trust in AI technologies and encourage their responsible deployment.
The future of ethical AI hinges on collaborative efforts among technologists, ethicists, and policymakers. As we envision a landscape where AI aligns with human values and societal goals, it becomes clear that we must work together to address the ethical challenges that lie ahead. By fostering dialogue and collaboration, we can create AI systems that not only enhance our lives but also uphold our shared values.
- What is AI ethics? AI ethics refers to the moral implications and responsibilities associated with the development and deployment of artificial intelligence technologies.
- Why is bias in AI a concern? Bias in AI can lead to unfair treatment and discrimination, perpetuating existing inequalities in society.
- How can we ensure transparency in AI? Transparency can be achieved through clear communication about how algorithms make decisions and regular audits of AI systems.
- What role do regulations play in AI ethics? Regulations help protect individual rights and ensure that AI technologies are developed and used responsibly.
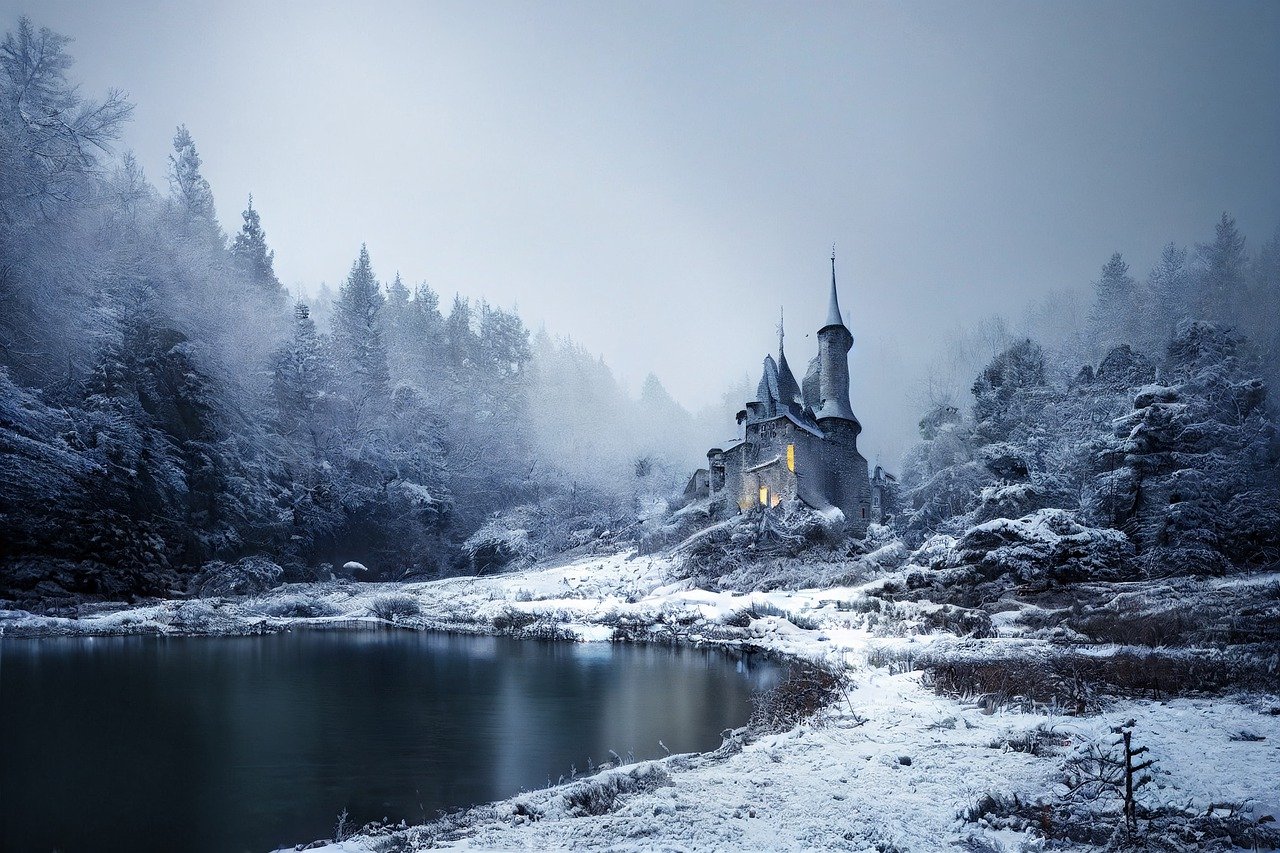
The Ethical Landscape of AI
Understanding the ethical landscape surrounding artificial intelligence (AI) is akin to navigating a complex maze filled with moral dilemmas and societal implications. At its core, the development and deployment of AI technologies must be guided by foundational principles that ensure they serve humanity positively. These principles include fairness, accountability, and transparency. Each of these elements plays a crucial role in fostering responsible AI usage and mitigating the potential for harm.
Imagine a world where AI systems are designed with a moral compass; this vision can only become a reality if developers and stakeholders prioritize ethical considerations from the outset. Fairness, for instance, is not merely a buzzword; it's a commitment to ensuring that AI systems do not perpetuate existing biases or create new forms of discrimination. When we talk about accountability, it’s about holding developers and organizations responsible for the decisions made by their AI systems. This means establishing frameworks that allow for scrutiny and oversight, ensuring that AI technologies are not operating in a black box.
Transparency is equally vital. Users should have a clear understanding of how AI systems make decisions that affect their lives. This transparency builds trust and allows individuals to question and challenge the outcomes produced by AI. Without it, we risk creating a society where people are at the mercy of algorithms they do not understand. The ethical landscape of AI isn't just a theoretical discussion; it has real-world implications that affect everything from hiring practices to law enforcement.
To illustrate the importance of these principles, let’s consider a few key examples:
- Fairness: In hiring algorithms, if the training data is biased, the AI may unfairly favor certain demographics over others, leading to a lack of diversity in the workplace.
- Accountability: When an autonomous vehicle is involved in an accident, who is responsible? The manufacturer, the software developer, or the owner of the vehicle?
- Transparency: In healthcare, AI systems that assist in diagnosis must be able to explain their reasoning to doctors and patients to ensure informed decision-making.
These examples highlight the delicate balance that must be maintained in AI development. As we forge ahead into an era dominated by AI technologies, it is essential to keep these ethical principles at the forefront of our discussions and decisions. The future of AI should not only focus on innovation and efficiency but also on how these advancements align with our shared moral values and societal norms.
Q: Why is fairness important in AI?
A: Fairness ensures that AI systems do not reinforce existing biases or create new forms of discrimination, promoting equality and justice in their outcomes.
Q: How can accountability be established in AI systems?
A: Accountability can be established through regulatory frameworks that require transparency and oversight of AI technologies, ensuring that developers are responsible for their systems' decisions.
Q: What role does transparency play in AI?
A: Transparency allows users to understand how AI systems make decisions, fostering trust and enabling individuals to question and challenge outcomes.
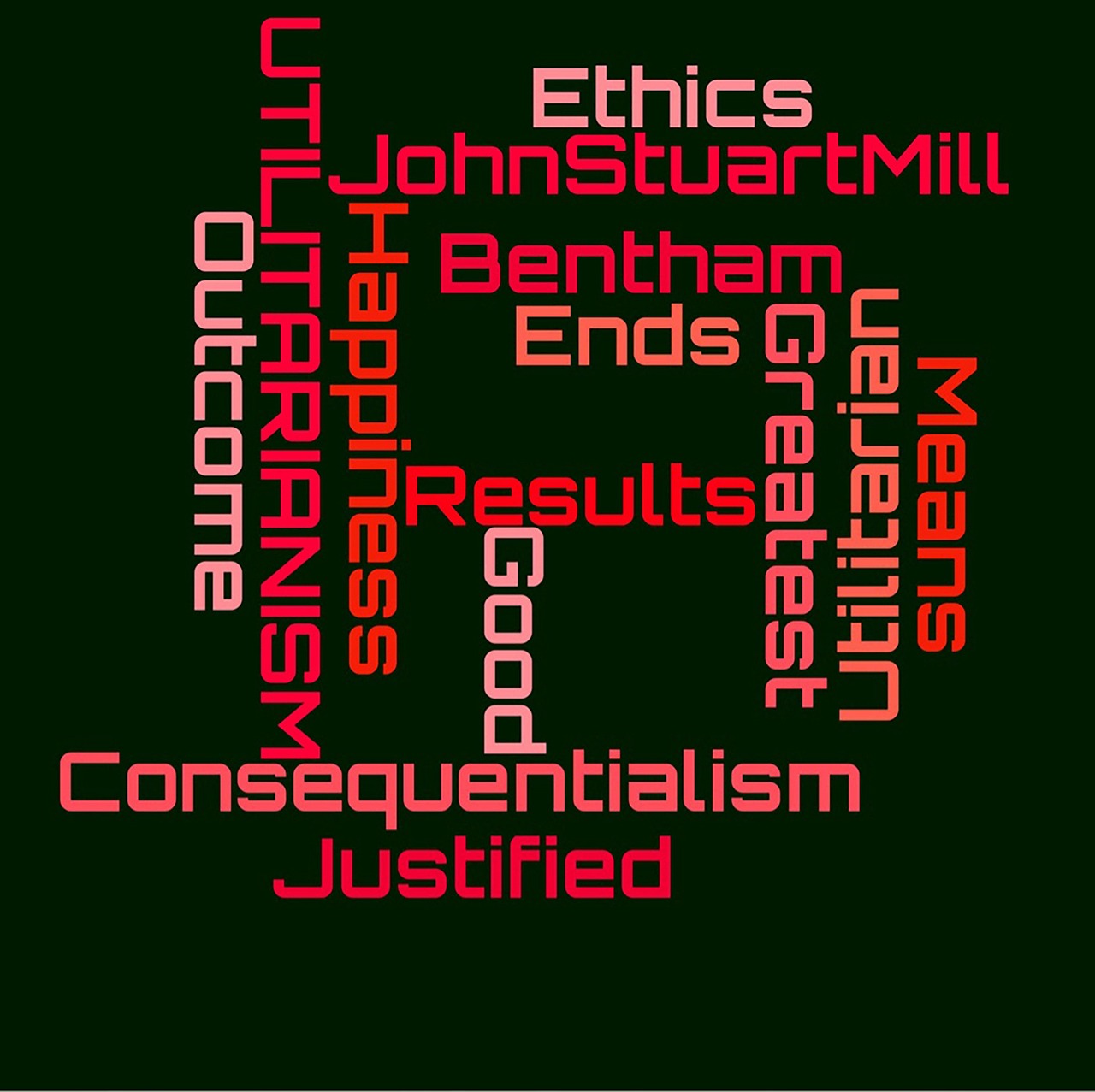
Bias in AI Algorithms
Bias in AI algorithms poses significant ethical challenges that cannot be ignored. Imagine walking into a store and finding that the products on display only cater to a specific demographic, while others are entirely overlooked. This is akin to what happens when AI systems are trained on biased data. The implications of biased algorithms can lead to discrimination, inequality, and a lack of trust in technology. As AI becomes increasingly integrated into our daily lives, understanding the sources of bias and how to mitigate its effects is crucial for fostering a fair and just society.
One of the primary sources of bias in AI algorithms stems from the data used to train these systems. If the data reflects historical inequalities or societal prejudices, the AI will likely perpetuate those biases. For instance, if an AI system is trained on hiring data that predominantly features candidates from a particular background, it may inadvertently disadvantage applicants from other backgrounds. This is not just a technical issue; it’s a moral one that can have real-world consequences.
To tackle this issue, it’s essential to identify the common pitfalls that lead to biased outcomes. Here are a few key sources:
- Skewed Datasets: Datasets that lack diversity can result in algorithms that do not perform well across different demographic groups.
- Historical Bias: If past data reflects societal biases, the AI will likely learn and replicate these biases.
- Labeling Errors: Inaccurate labeling of training data can lead to misinterpretations by the AI, further entrenching biases.
The role of data quality cannot be overstated. High-quality, diverse, and representative datasets are essential for developing fair AI systems. When datasets include a wide range of perspectives and experiences, the AI can learn to make decisions that are more inclusive and equitable. For example, in healthcare AI, using diverse patient data can lead to better health outcomes for underrepresented groups. On the other hand, if the data is biased, the AI may produce skewed results, leading to poor decision-making and reinforcing existing inequalities.
Another critical aspect of addressing bias in AI is algorithmic transparency. Users and stakeholders deserve to understand how decisions are made by AI systems. Without transparency, it becomes challenging to hold these systems accountable. Imagine if you received a rejection letter from a job application, but you had no idea why. This lack of clarity can foster distrust and frustration. Therefore, developers must strive for clear explanations of how algorithms function and the factors that influence their decisions.
In conclusion, addressing bias in AI algorithms requires a multifaceted approach that includes improving data quality, enhancing transparency, and actively seeking to understand and mitigate bias. By doing so, we can create AI systems that not only perform better but also align with our shared values of fairness and equality. The journey toward unbiased AI is ongoing, but with concerted effort and vigilance, we can work towards a future where technology serves all of humanity fairly.
- What is bias in AI? Bias in AI refers to the tendency of algorithms to produce results that are systematically prejudiced due to erroneous assumptions in the machine learning process.
- How does bias affect AI decisions? Bias can lead to unfair treatment of individuals or groups, resulting in discriminatory practices in areas like hiring, lending, and law enforcement.
- What can be done to reduce bias in AI? Strategies include using diverse datasets, conducting regular audits of algorithms, and ensuring transparency in how decisions are made.
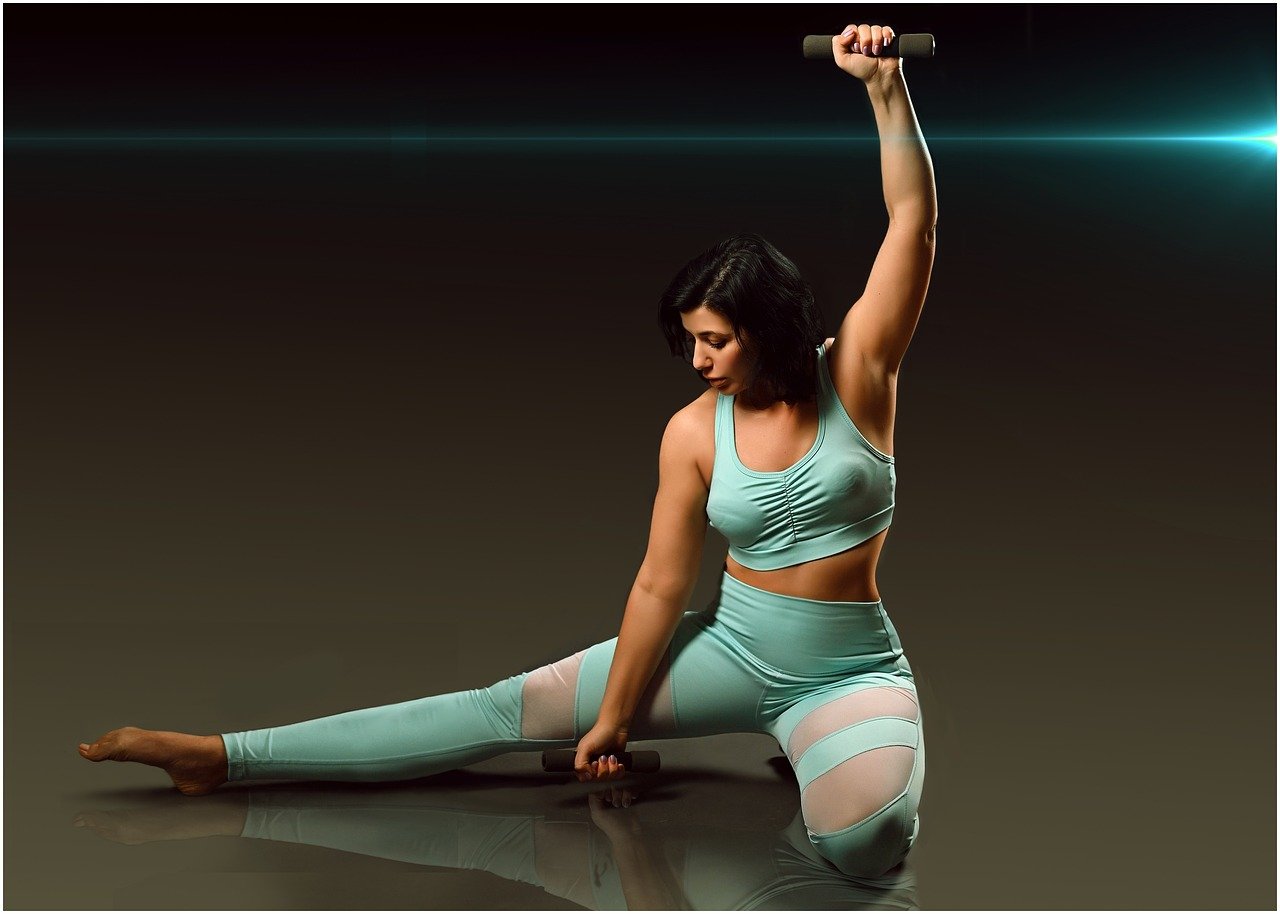
Identifying Sources of Bias
Identifying sources of bias in artificial intelligence (AI) is a critical step toward creating systems that are not only effective but also fair and equitable. Bias can creep into AI systems in various ways, often stemming from the data used to train these algorithms. Imagine trying to bake a cake with rotten ingredients; no matter how skilled the baker, the end result will be subpar. Similarly, if the data fed into AI systems is flawed or skewed, the outputs will reflect those imperfections, leading to biased outcomes.
One of the primary sources of bias is historical bias. This occurs when the data reflects past prejudices or inequalities. For instance, if an AI system is trained on historical hiring data from a company that has a history of gender discrimination, it may inadvertently learn to favor male candidates over equally qualified female candidates. This is not just a technical failure; it’s a moral one that can have real-world consequences for individuals and communities.
Another significant source is sampling bias, which arises when the data collected is not representative of the population it aims to serve. For example, if an AI system designed for healthcare is trained predominantly on data from a specific demographic, it may not perform well for individuals outside that group. This can lead to disparities in treatment recommendations, further exacerbating existing inequalities in healthcare access and outcomes.
Moreover, labeling bias can also play a pivotal role. When humans label data, their own biases can influence the categorization process. For instance, if a dataset used for training a facial recognition system contains predominantly images of light-skinned individuals, the algorithm may struggle to accurately identify individuals with darker skin tones. This is a clear example of how bias can manifest through human error, leading to technologies that fail to serve everyone equally.
To effectively identify these biases, developers need to implement rigorous testing and evaluation processes. This can include:
- Conducting audits of training datasets to ensure diversity and representation.
- Engaging in regular assessments of AI outputs to identify any patterns of bias.
- Collaborating with diverse teams to bring multiple perspectives to the data analysis process.
In conclusion, recognizing and addressing sources of bias is not just a technical challenge; it's a moral imperative. By taking proactive steps to identify and mitigate bias, we can work towards AI systems that genuinely reflect our diverse society and uphold the principles of justice and equality.

The Role of Data Quality
When it comes to artificial intelligence, the phrase "garbage in, garbage out" couldn't be more accurate. The quality of data used in AI systems is not just a technical detail; it's the very foundation upon which ethical AI is built. If the data is flawed, biased, or unrepresentative, the AI's decisions will reflect those same issues. Imagine trying to bake a cake with spoiled ingredients; no matter how skilled the baker, the end result will be a disaster. Similarly, AI systems trained on poor-quality data can lead to disastrous outcomes, including discrimination and misrepresentation.
Data quality encompasses several factors, including accuracy, completeness, consistency, and timeliness. Each of these elements plays a critical role in ensuring that AI systems not only function effectively but also uphold ethical standards. For instance, if an AI system is trained on incomplete data, it may develop a skewed understanding of reality, leading to biased conclusions. In a world increasingly reliant on AI for decision-making, ensuring high data quality is not just a technical requirement; it’s a moral imperative.
To illustrate the importance of data quality, consider the following table that outlines key aspects of data quality and their implications for AI:
Data Quality Aspect | Description | Implications for AI |
---|---|---|
Accuracy | The degree to which data is correct and free from error. | Inaccurate data can lead to faulty predictions and decisions. |
Completeness | The extent to which all required data is present. | Missing data can lead to gaps in decision-making and analysis. |
Consistency | The degree to which data is uniform across different datasets. | Inconsistent data can confuse algorithms and lead to unreliable outcomes. |
Timeliness | The relevance of data based on its age and how up-to-date it is. | Outdated data can misinform AI systems, leading to irrelevant or incorrect decisions. |
Furthermore, the importance of diversity in datasets cannot be overstated. A diverse dataset reflects a wide range of perspectives and experiences, which helps to minimize bias. Think of it this way: if you only gather opinions from a single group of people, you're likely to miss out on valuable insights from others. By incorporating a variety of data sources, AI developers can create systems that are more equitable and better aligned with the needs of all users.
In summary, the role of data quality in AI development is paramount. By ensuring that data is accurate, complete, consistent, timely, and diverse, we can build AI systems that not only perform well but also adhere to ethical standards. As we continue to navigate the complex landscape of AI, prioritizing data quality will be essential in fostering trust and accountability in these technologies.
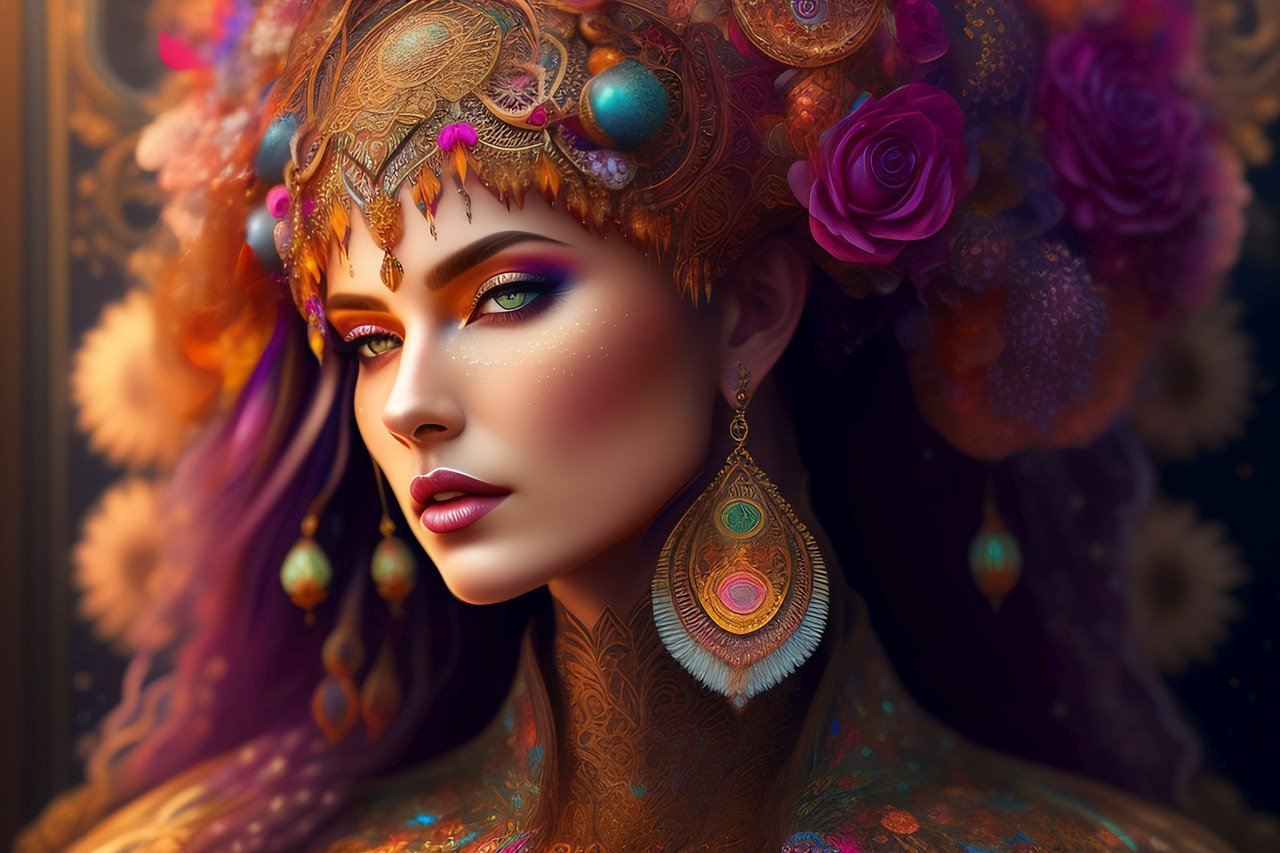
Algorithmic Transparency
In the realm of artificial intelligence, serves as a beacon of accountability. Imagine navigating a maze where every twist and turn is shrouded in darkness—this is how users often feel when interacting with AI systems that lack transparency. When we talk about algorithmic transparency, we are essentially asking for clarity on how decisions are made by these complex systems. This is not just a technical issue; it’s a matter of trust, ethics, and societal impact.
Transparency in algorithms means that both developers and users can understand the logic behind AI decisions. It’s like having a recipe that not only lists the ingredients but also explains the cooking process. Without this understanding, users may find themselves at the mercy of a system that could perpetuate biases or make errors without any way to challenge them. For instance, if an AI system denies a loan application, the applicant deserves to know why. Was it based on their credit score, income, or perhaps a flawed dataset? The lack of clarity can lead to feelings of injustice and frustration, which only erodes trust in technology.
To illustrate the importance of algorithmic transparency, consider the following key aspects:
- Explainability: Users should be able to receive explanations for AI decisions in a language they can understand. This fosters a sense of empowerment and control.
- Accountability: Clear algorithms allow stakeholders to hold developers accountable for the outcomes produced by AI. If something goes wrong, there should be a clear path to address the issue.
- Ethical Compliance: Transparency ensures that AI systems adhere to ethical standards and societal norms, making it easier to identify and rectify any potential misconduct.
Moreover, the implications of algorithmic transparency extend beyond individual users. Organizations and governments have a vested interest in ensuring that AI systems operate fairly and justly. For example, public agencies using AI for law enforcement must be transparent about how predictive policing algorithms are developed and deployed. This is crucial to prevent discriminatory practices that can arise from biased data or flawed algorithms.
In essence, algorithmic transparency is not merely a technical requirement; it is a fundamental ethical obligation. As we look to the future, the demand for transparency will only grow. Consumers will increasingly expect to know how their data is being used and how decisions are being made. Therefore, developers must embrace this transparency, not just as a compliance measure, but as a core value that aligns with the broader goals of ethical AI development.
- What is algorithmic transparency? Algorithmic transparency refers to the clarity and openness regarding how AI algorithms make decisions, allowing users to understand the reasoning behind outcomes.
- Why is algorithmic transparency important? It is crucial for building trust, ensuring accountability, and preventing biases in AI systems, ultimately leading to fairer outcomes.
- How can organizations improve algorithmic transparency? Organizations can improve transparency by providing clear explanations of their algorithms, conducting audits, and engaging with stakeholders to discuss AI decision-making processes.
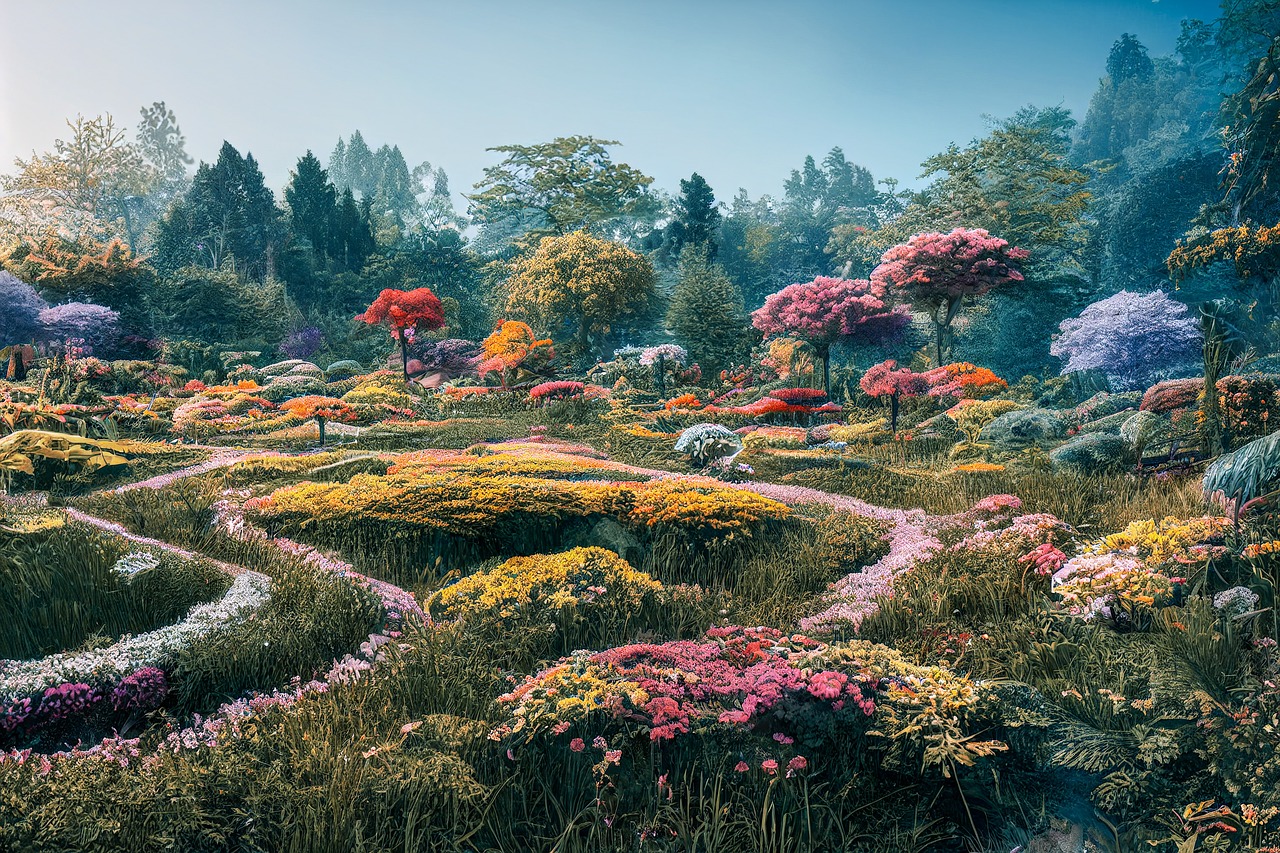
Mitigating Bias in AI
Mitigating bias in AI is not just a technical challenge; it's a moral imperative. As we integrate AI into more aspects of our daily lives, the consequences of biased algorithms can be severe, affecting everything from hiring practices to law enforcement. To tackle this issue effectively, we need to adopt a multi-faceted approach that includes rigorous algorithm audits, diverse data collection, and inclusive design practices. By doing so, we can ensure that AI systems serve all segments of society fairly and equitably.
One of the most effective strategies involves conducting regular algorithm audits. These audits help identify biases that may be lurking within the system. Think of it like a routine health check-up for your AI; just as you would want to catch any health issues early, the same principle applies here. Regular evaluations can uncover hidden biases and allow for timely adjustments. For instance, if an AI system used in recruitment shows a preference for candidates from a specific demographic, an audit can reveal this bias, prompting corrective measures.
Another crucial aspect is the inclusivity of design practices. When developing AI systems, it’s essential to involve a diverse group of people in the design process. This diversity can help identify potential biases that a homogeneous team might overlook. Imagine a group of chefs preparing a meal; if they all come from the same culinary background, the dish may lack the richness of flavors that come from different cuisines. Similarly, diverse teams can bring varied perspectives that enhance the AI's fairness and effectiveness.
Moreover, the quality of data used in training AI models cannot be overstated. Using datasets that are representative of the entire population is vital for minimizing bias. For example, if an AI model trained on data predominantly from one demographic group is then applied to a broader audience, it may not perform well for those outside the original group. Thus, ensuring data diversity is key. This can involve actively seeking out underrepresented groups in data collection efforts, thereby enriching the dataset and improving the AI's performance across different demographics.
To further enhance our efforts in mitigating bias, organizations should implement continuous feedback mechanisms. This involves creating channels for users and stakeholders to report issues they encounter with AI systems. For instance, if a user feels that a recommendation system is biased, they should have an easy way to flag this concern. This feedback can then be analyzed and used to make necessary adjustments, ensuring that the AI evolves and improves over time.
Lastly, education plays a pivotal role in this journey. Training developers and stakeholders on the ethical implications of AI and the importance of bias mitigation can foster a culture of responsibility. By understanding the impact of their work, developers are more likely to prioritize fairness and equity in their designs. It’s akin to teaching a driver about road safety; the more they know, the more cautious they will be.
In summary, mitigating bias in AI requires a comprehensive strategy that includes algorithm audits, inclusive design, quality data practices, continuous feedback, and education. By embracing these approaches, we can work towards creating AI systems that are not only advanced but also just and equitable, ensuring they benefit everyone.
- What is bias in AI? Bias in AI refers to systematic and unfair discrimination against certain groups of individuals, often resulting from the data used to train the AI models.
- How can algorithm audits help? Algorithm audits help identify and rectify biases in AI systems by evaluating their decision-making processes and outputs.
- Why is data diversity important? Data diversity ensures that AI systems are trained on a wide range of inputs, making them more accurate and fair for all users.
- What role does education play in mitigating bias? Education raises awareness among developers and stakeholders about the ethical implications of AI, encouraging them to prioritize fairness in their work.

AI and Privacy Concerns
In our rapidly advancing digital age, the intersection of artificial intelligence and privacy has become a hotbed of discussion and concern. As AI technologies evolve, they often require vast amounts of data to function effectively. This data can include everything from personal information to behavioral patterns, raising significant ethical questions about how this information is collected, used, and protected. Imagine walking through a crowded street, your every move monitored and analyzed by unseen algorithms—sounds unsettling, right? That's the reality many face as AI systems become more integrated into our daily lives.
The implications of AI on individual privacy are profound. As AI systems are designed to learn from user interactions, the risk of data misuse becomes a pressing issue. For instance, consider the case of facial recognition technology. While it can enhance security, it also poses a threat to personal privacy, as individuals may be tracked without their consent. This duality of AI technology creates a tension between innovation and ethical responsibility. How do we harness the power of AI while ensuring that individual rights are respected?
To navigate these complex waters, regulatory frameworks are essential. In the European Union, for example, the General Data Protection Regulation (GDPR) has set a precedent for data protection, providing individuals with rights over their personal data. This regulation mandates that organizations must obtain explicit consent before collecting data, ensuring that users are informed about how their information will be used. Such regulations not only protect individuals but also foster trust in AI technologies, which is crucial for their widespread acceptance.
Moreover, ethical data usage is vital in maintaining this trust. Companies developing AI systems must prioritize responsible data handling practices. This includes implementing measures to anonymize data, ensuring that sensitive information is not easily identifiable. By doing so, organizations can minimize the risks associated with data breaches and unauthorized access, ultimately safeguarding user privacy. The question remains: how can companies balance the need for data with the necessity of protecting individual rights?
As we look to the future, the conversation around AI and privacy will continue to evolve. It's not just about compliance with regulations; it's about fostering a culture of ethical responsibility. Companies must engage with ethicists, technologists, and the public to create AI systems that not only advance technology but also respect individual privacy. This collaborative approach can lead to innovative solutions that prioritize user rights while still leveraging the benefits of AI.
In conclusion, the relationship between AI and privacy is intricate and multifaceted. As we embrace the potential of AI, we must remain vigilant about the ethical implications of its use. Striking a balance between technological advancement and privacy protection is not just a challenge; it's an imperative for a future where AI serves humanity without compromising our fundamental rights.
- What are the main privacy concerns related to AI? The main concerns include data collection, consent, surveillance, and data breaches.
- How does GDPR protect individual privacy in AI? GDPR requires explicit consent for data collection and gives individuals rights over their personal data.
- What can companies do to ensure ethical data usage? Companies can implement data anonymization, obtain informed consent, and prioritize transparency in their data practices.
- Is it possible to balance AI innovation with privacy protection? Yes, through collaborative efforts among stakeholders, ethical frameworks, and regulatory compliance, a balance can be achieved.
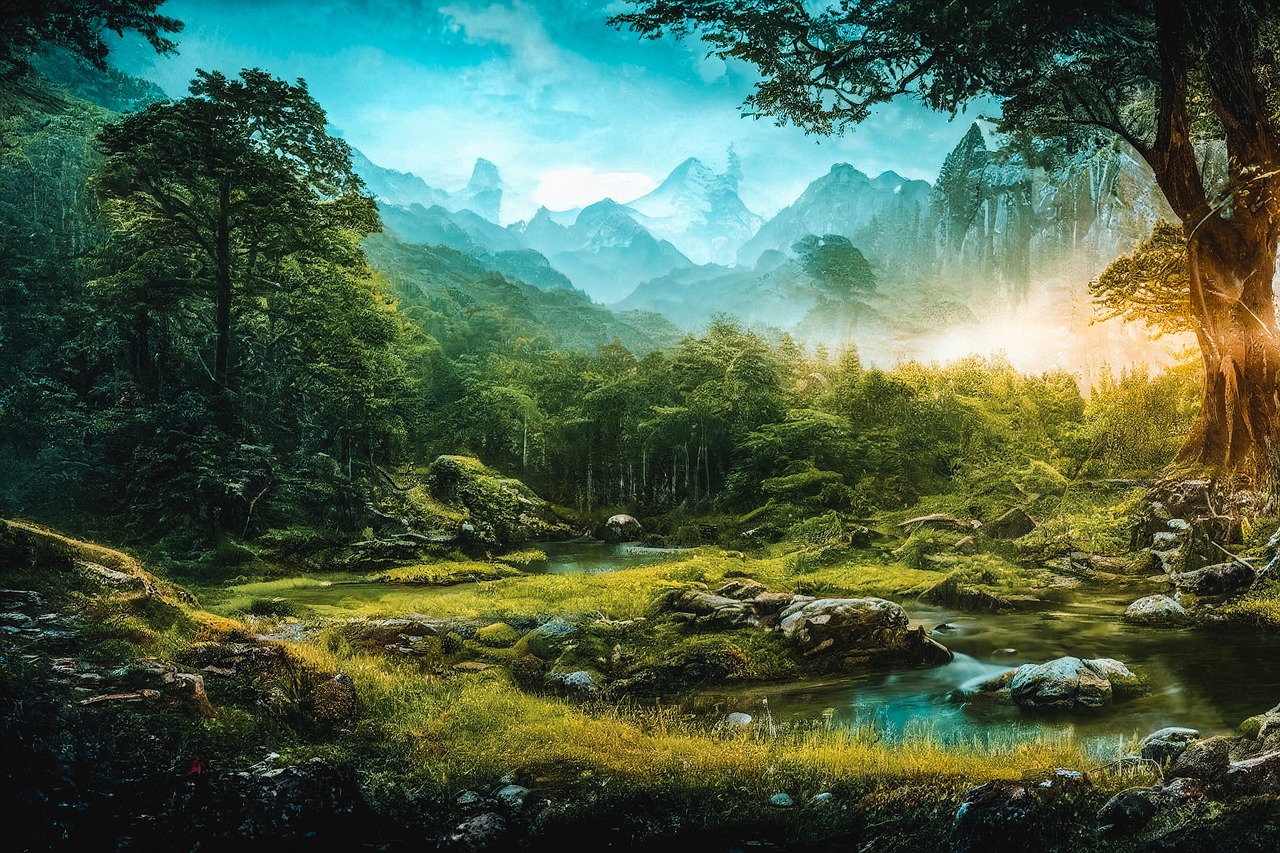
Data Protection Regulations
In today's digital age, where artificial intelligence (AI) systems are rapidly evolving, the importance of cannot be overstated. These regulations serve as a crucial framework to ensure that individuals' personal information is handled with care and respect. One of the most notable regulations is the General Data Protection Regulation (GDPR), which was implemented by the European Union in 2018. GDPR not only sets stringent guidelines for data collection and processing but also empowers individuals with rights over their own data. This means that users can access, modify, and even delete their personal information held by companies.
Moreover, the implications of these regulations extend beyond just compliance; they shape the very fabric of trust between consumers and AI technologies. When users know that their data is protected, they are more likely to engage with AI applications. However, the challenge lies in ensuring that all AI developers and companies adhere to these regulations. Non-compliance can lead to hefty fines and a loss of consumer trust, which can be detrimental in a competitive market.
To illustrate the impact of data protection regulations, let's take a look at some key aspects of GDPR:
Aspect | Description |
---|---|
Data Subject Rights | Individuals have the right to access their data, rectify inaccuracies, and request deletion. |
Consent | Companies must obtain clear and explicit consent from users before collecting their data. |
Data Breach Notification | Organizations must notify authorities and affected individuals within 72 hours of a data breach. |
Privacy by Design | Data protection measures must be integrated into the development of AI systems from the outset. |
In addition to GDPR, various regions and countries are developing their own regulations, which can lead to a complex web of compliance requirements for global AI companies. For instance, California's California Consumer Privacy Act (CCPA) provides similar protections as GDPR but has its own unique stipulations. This divergence in regulations highlights the necessity for AI developers to stay informed and adaptable.
As AI continues to permeate various sectors, including healthcare, finance, and education, the ethical implications of data usage become increasingly significant. Organizations must prioritize ethical data usage, ensuring that any data collected is not only compliant with regulations but also respects the privacy and autonomy of individuals. This means implementing robust data governance frameworks and fostering a culture of transparency within organizations.
Ultimately, the future of AI and its ethical landscape will be shaped by how well companies navigate these regulations. By prioritizing data protection, businesses can not only comply with legal requirements but also build a foundation of trust with their users. After all, in the world of AI, trust is the currency that can drive innovation and adoption.
- What is GDPR? GDPR stands for General Data Protection Regulation, a comprehensive data protection law in the EU.
- How does GDPR affect AI? GDPR requires AI companies to handle personal data responsibly, ensuring user rights are upheld.
- What are the penalties for non-compliance? Companies can face fines up to 4% of their annual global revenue or €20 million, whichever is higher.
- Are there other data protection laws? Yes, laws like CCPA in California and various other national regulations exist globally.

Ethical Data Usage
In today's data-driven world, the concept of is more critical than ever. As artificial intelligence (AI) systems become increasingly integrated into our daily lives, the way we handle data can significantly impact trust and accountability. It's not just about collecting data; it's about how we use it, who we share it with, and whether we respect the rights of individuals from whom this data is sourced. Imagine a world where every click, every purchase, and every interaction is meticulously monitored and analyzed. Sounds convenient, right? But what happens when that convenience comes at the cost of personal privacy?
To maintain trust in AI systems, organizations must prioritize ethical data practices. This involves ensuring informed consent from users before collecting their data, being transparent about how that data will be used, and implementing robust safeguards to protect sensitive information. Informed consent means that individuals understand what data is being collected, how it will be used, and the potential risks involved. This is not just a checkbox exercise; it requires clear communication and a genuine effort to empower users.
Moreover, ethical data usage extends beyond just consent. It also encompasses the principle of data minimization, which advocates for the collection of only the data necessary for a specific purpose. By limiting data collection, organizations can reduce the risk of breaches and misuse. This principle is particularly crucial in AI, where vast amounts of data can lead to unintended consequences if not handled properly. For instance, if an AI system collects personal data without a clear purpose, it not only raises ethical concerns but also opens the door to potential legal ramifications.
Another vital aspect of ethical data usage is the implementation of responsible data handling practices. Organizations should establish clear policies on data storage, sharing, and disposal to ensure that data is not kept longer than necessary or shared without proper authorization. This involves regular audits and assessments to identify potential vulnerabilities in data management processes. The goal here is to create a culture of accountability where everyone involved in data handling understands their responsibilities and the ethical implications of their actions.
In conclusion, ethical data usage is not merely a regulatory requirement; it's a fundamental pillar that supports the integrity of AI systems. By prioritizing informed consent, data minimization, and responsible handling practices, organizations can foster a trustworthy environment that respects individual privacy. As we move forward into an era dominated by AI, it's essential that we keep these ethical considerations at the forefront of our data practices. After all, in the grand scheme of things, it's not just about the data; it's about the people behind it.
- What is ethical data usage? Ethical data usage refers to the responsible collection, handling, and processing of data that respects individual privacy and rights.
- Why is informed consent important? Informed consent ensures that individuals are aware of how their data will be used and allows them to make informed decisions about sharing their information.
- How can organizations ensure ethical data practices? Organizations can implement policies for data minimization, transparency in data usage, and regular audits to maintain ethical standards.
- What are the consequences of unethical data usage? Unethical data usage can lead to loss of trust, legal repercussions, and potential harm to individuals whose data is misused.
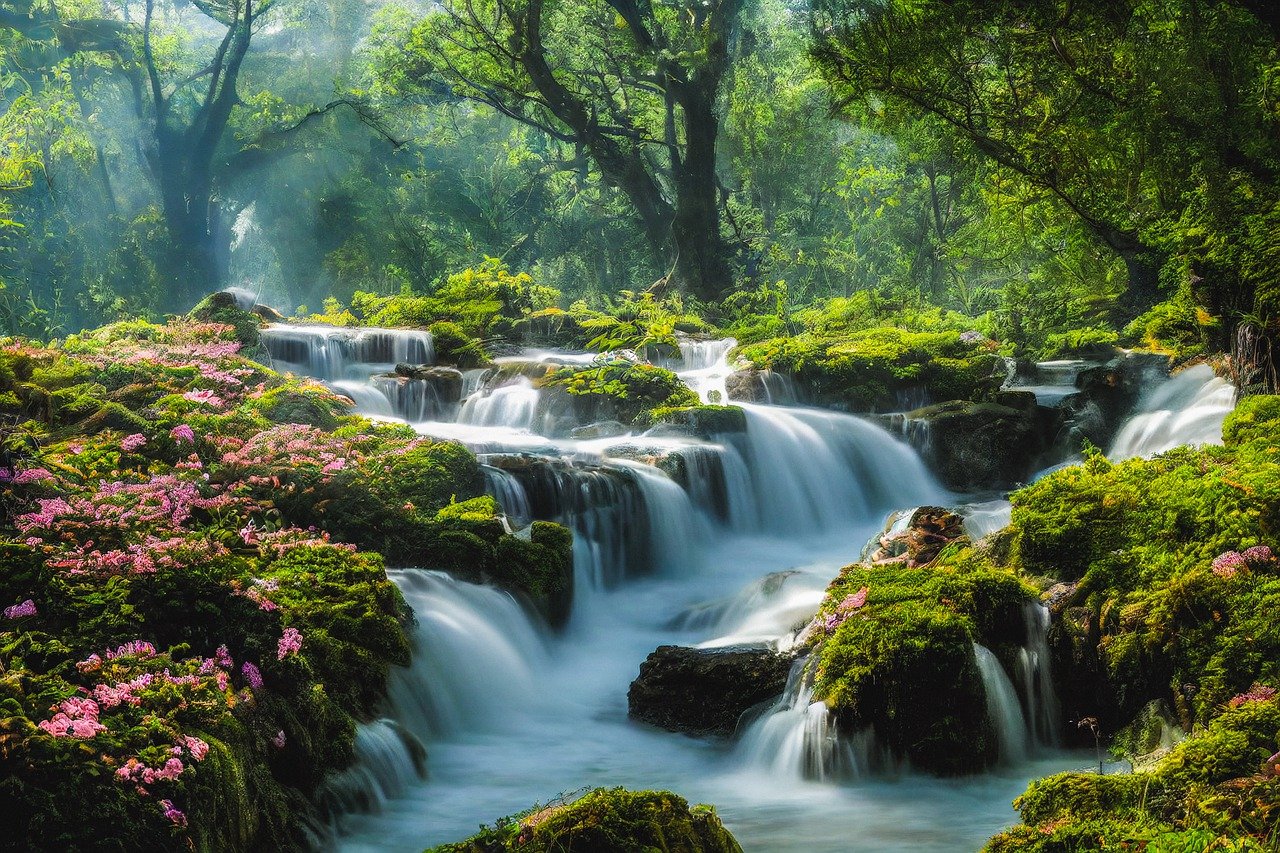
The Future of Ethical AI
The future of ethical AI is not just a distant dream; it is a necessity that we must actively pursue today. Imagine a world where artificial intelligence not only enhances our daily lives but also aligns seamlessly with our moral compass and societal values. This vision can only be achieved through collaborative efforts among technologists, ethicists, and policymakers. The convergence of these diverse perspectives is crucial for developing AI systems that respect human dignity and promote equality.
As we look ahead, several key factors will shape the ethical landscape of AI. First and foremost, we need to prioritize interdisciplinary collaboration. By bringing together experts from various fields, we can ensure that the development of AI technologies is informed by a broad range of insights and ethical considerations. For instance, ethicists can provide guidance on moral dilemmas, while technologists can share the technical challenges of implementing ethical guidelines in AI systems.
Furthermore, public engagement is essential. The voices of everyday people must be included in discussions about AI's future. After all, it is the public that will be most affected by these technologies. By fostering open dialogues, we can better understand societal concerns and expectations regarding AI. This engagement can take many forms, such as community forums, surveys, and collaborative workshops, allowing stakeholders to express their thoughts and ideas on ethical AI development.
Another critical aspect is the establishment of robust regulatory frameworks. Policymakers must create regulations that not only address current ethical challenges but also anticipate future developments in AI technology. These frameworks should promote transparency, accountability, and fairness in AI systems. For example, regulations could require companies to conduct regular audits of their AI algorithms to ensure they adhere to ethical standards. This proactive approach can help mitigate risks before they escalate into significant issues.
Moreover, education plays a vital role in shaping the future of ethical AI. We must equip future generations with the knowledge and skills necessary to navigate the complexities of AI technology. Educational institutions should integrate ethics into their STEM curricula, fostering a culture of responsibility among budding technologists. By instilling a strong ethical foundation, we can empower individuals to make informed decisions in their professional lives, ensuring that AI development remains aligned with human values.
Finally, the concept of ethical AI design should be at the forefront of technological innovation. This means incorporating ethical considerations into the design process from the very beginning, rather than as an afterthought. By adopting inclusive design practices, developers can create AI systems that cater to diverse user needs and reduce the risk of bias. For instance, utilizing a wide range of datasets during the training phase can help ensure that AI algorithms are fair and representative of all demographics.
In summary, the future of ethical AI is a collective responsibility that requires collaboration, public engagement, regulatory frameworks, education, and ethical design practices. As we move forward, let us strive to create AI technologies that enhance our lives while upholding our shared values. The journey may be challenging, but the potential rewards—a more equitable, just, and humane society—are well worth the effort.
- What is ethical AI? Ethical AI refers to the development and implementation of artificial intelligence systems that adhere to moral principles and societal values, ensuring fairness, accountability, and transparency.
- Why is collaboration important in ethical AI? Collaboration among technologists, ethicists, and policymakers is crucial to address the multifaceted challenges of AI and to align technological advancements with human values.
- How can we mitigate bias in AI systems? Mitigating bias involves using diverse datasets, conducting regular audits, and implementing inclusive design practices to ensure fairness in AI outcomes.
- What role does education play in the future of ethical AI? Education helps prepare future generations to understand and navigate the ethical implications of AI, fostering a culture of responsibility in technology development.
Frequently Asked Questions
- What are the main ethical principles guiding AI development?
The primary ethical principles guiding AI development include fairness, accountability, and transparency. These principles are crucial for ensuring that AI technologies are developed and used responsibly, minimizing harm and promoting trust among users.
- How does bias affect AI algorithms?
Bias in AI algorithms can lead to discrimination and inequality in outcomes. If the data used to train these algorithms is biased, the AI can perpetuate or even exacerbate existing societal biases, resulting in unfair treatment of certain groups.
- What are common sources of bias in AI?
Common sources of bias in AI include skewed datasets, which may not represent the diversity of the population, and algorithmic design choices that inadvertently favor certain outcomes over others. Recognizing these pitfalls is essential for creating fair AI systems.
- Why is data quality important in AI?
Data quality is critical because it directly impacts the performance and fairness of AI systems. Using diverse and representative datasets helps ensure that AI outcomes are equitable and reliable, reducing the risk of bias.
- What is algorithmic transparency?
Algorithmic transparency refers to the clarity and openness surrounding how AI algorithms make decisions. It is essential for accountability, allowing users and stakeholders to understand the reasoning behind AI outcomes and fostering trust in the technology.
- How can bias in AI be mitigated?
Mitigating bias in AI requires proactive strategies such as conducting algorithm audits and adopting inclusive design practices. These approaches help ensure fairness and reduce the potential for discrimination in AI applications.
- What privacy concerns are associated with AI?
AI technologies often raise significant privacy concerns, especially regarding data collection and usage. These concerns emphasize the need for ethical practices in handling personal data to maintain user trust.
- How do data protection regulations affect AI?
Data protection regulations, such as the GDPR, play a vital role in safeguarding privacy in AI applications. They establish guidelines for data handling, ensuring that user rights are respected and promoting responsible AI development.
- What is ethical data usage in AI?
Ethical data usage involves practices that prioritize informed consent and responsible data handling. These practices are essential for maintaining trust in AI systems and ensuring that data is used in a manner that respects individual privacy.
- What does the future of ethical AI look like?
The future of ethical AI relies on collaboration among technologists, ethicists, and policymakers. By working together, these groups can create an AI landscape that aligns with human values and societal goals, paving the way for a better tomorrow.