Ensuring Ethical Consistency in AI Applications
As we dive into the world of artificial intelligence (AI), it becomes increasingly evident that ethical considerations are not just an afterthought; they are the backbone of responsible AI development. Imagine a world where AI systems operate with the same moral compass that guides human interactions. This is not merely a dream; it's a necessity. With rapid advancements in technology, the potential for AI to impact our lives is immense, but so are the ethical dilemmas that come along with it. From healthcare to finance, AI applications have the power to revolutionize industries, yet they also carry the risk of perpetuating biases and eroding trust if not managed correctly. Therefore, ensuring ethical consistency in AI applications is paramount for fostering a safe and equitable future.
Establishing robust ethical frameworks is essential for guiding AI development and ensuring that applications align with societal values and norms. Think of these frameworks as the guiding principles that help us navigate the murky waters of AI ethics. They foster trust and accountability in AI systems, ensuring that developers are not just creating algorithms but are also considering the broader implications of their work. Without such frameworks, we risk creating a technological landscape that operates in a moral vacuum, leading to decisions that might benefit a few at the expense of many. It's not just about what AI can do, but what it *should* do.
Despite the growing emphasis on ethics, various challenges hinder the effective implementation of ethical principles in AI. These challenges include biases, lack of transparency, and the rapid pace of technological advancement. For instance, how can we expect AI to make fair decisions if the data it learns from is skewed? The reality is that biases in AI can lead to unfair outcomes, perpetuating existing inequalities. The challenge is not just identifying these biases but also understanding their roots and implications. Additionally, the speed at which technology evolves can outpace our ability to regulate and ensure ethical compliance, leaving a gap that can be exploited.
Bias in AI is a pressing concern that can lead to outcomes that are not just unfair but also damaging. Imagine a hiring algorithm that favors one demographic over another simply because it was trained on biased data. This is where the importance of identifying and mitigating bias becomes crucial. We need to develop fair and equitable AI applications that serve diverse populations. This isn't just about fairness; it's about creating systems that reflect and respect the diversity of the world we live in.
Various techniques can be employed to mitigate bias in AI systems. These include:
- Algorithmic Adjustments: Tweaking algorithms to ensure they do not favor one group over another.
- Diverse Training Data: Ensuring that the data used for training AI systems represents a wide array of demographics.
- Regular Audits: Conducting audits to check for biases and making necessary adjustments.
By adopting these techniques, we can work towards ensuring that AI applications are more representative and just for all users.
Examining case studies where bias affected AI outcomes can provide valuable insights into the importance of addressing these issues. For instance, consider the case of a facial recognition system that performed poorly on individuals with darker skin tones. Such examples highlight the urgent need for bias mitigation strategies in AI development. By analyzing these instances, we can learn from past mistakes and implement effective solutions in future AI projects.
Transparency in AI processes is vital for accountability. It enables stakeholders to understand how decisions are made and fosters trust across various sectors. When people know how an AI system operates, they are more likely to trust its decisions. Imagine if every time you received a recommendation from an AI, you could see the data and logic behind it. This level of transparency could revolutionize how we interact with technology, making it more user-friendly and ethically sound.
Implementing best practices in AI development can help organizations align their technologies with ethical standards. This promotes responsible innovation and safeguards user rights and societal interests. To achieve this, organizations should prioritize ethical considerations from the outset of the development process, rather than as an afterthought.
Engaging stakeholders, including users, ethicists, and policymakers, is essential for understanding diverse perspectives. This engagement ensures that AI applications meet ethical expectations and community needs. By involving a wide range of voices in the development process, we can create AI systems that not only serve a purpose but also resonate with the values of the communities they impact.
Ongoing monitoring and evaluation of AI systems are necessary to identify ethical concerns and adapt practices accordingly. This proactive approach ensures that AI applications remain aligned with established ethical frameworks over time. Just like any good relationship, maintaining ethical consistency requires ongoing communication and adjustment.
Q1: Why is ethical AI important?
Ethical AI is crucial because it ensures that AI systems operate fairly and transparently, minimizing biases and fostering trust among users.
Q2: What are some common challenges in implementing ethical AI?
Common challenges include biases in data, lack of transparency in decision-making processes, and the rapid pace of technological advancements that outstrip regulatory measures.
Q3: How can bias in AI systems be mitigated?
Bias can be mitigated through techniques such as algorithmic adjustments, using diverse training data, and conducting regular audits of AI systems.
Q4: What role do stakeholders play in ethical AI development?
Stakeholders provide diverse perspectives that help ensure AI applications meet ethical standards and community needs, promoting more responsible innovation.
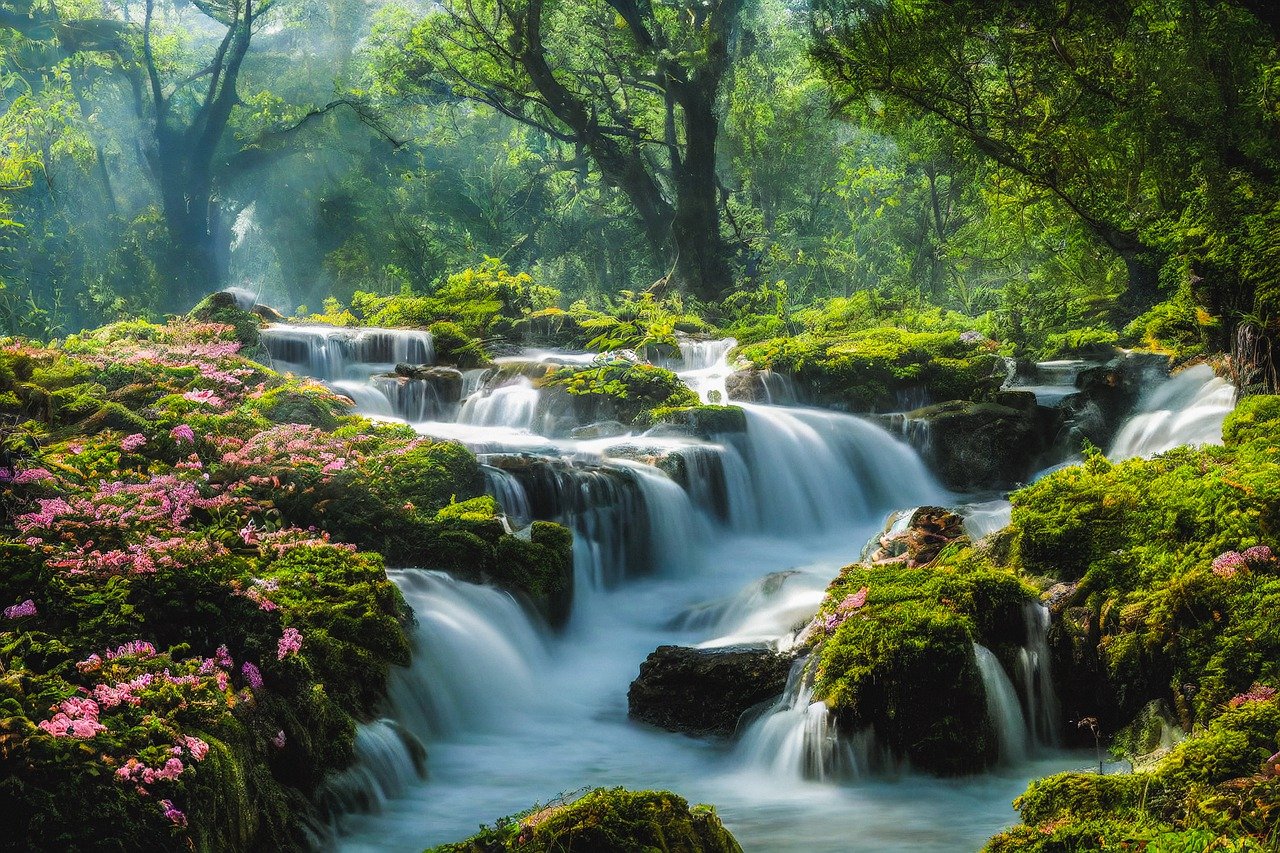
The Importance of Ethical Frameworks
In today’s rapidly evolving technological landscape, the significance of ethical frameworks in artificial intelligence (AI) cannot be overstated. As AI technologies permeate various aspects of our lives—from healthcare to finance—they bring along complex ethical dilemmas that demand careful consideration. Establishing robust ethical frameworks is not merely a recommendation; it is a necessity for guiding AI development. These frameworks serve as a compass, ensuring that applications align with societal values and norms, thereby fostering trust and accountability in AI systems.
Imagine a world where AI operates without ethical guidance. It’s like sailing a ship without a map; the potential for disaster is immense. Ethical frameworks provide that map, helping developers navigate the murky waters of AI ethics. They outline the principles that govern AI behavior, ensuring that technologies do not just function effectively but also respect human rights and dignity. This alignment with societal values is essential for building trust among users, as people are more likely to embrace technologies that they perceive as fair and just.
Moreover, ethical frameworks help organizations mitigate risks associated with AI deployment. By adhering to established guidelines, companies can avoid potential legal repercussions and reputational damage that may arise from unethical practices. For example, a well-defined ethical framework can guide organizations in making decisions about data privacy, ensuring that users’ information is handled responsibly. This not only protects users but also enhances the credibility of the organization in the eyes of the public.
To illustrate the importance of these frameworks, let’s consider a few key components that should be included:
- Transparency: Clear communication about how AI systems operate is crucial for user trust.
- Accountability: Establishing who is responsible for AI decisions helps in addressing ethical breaches.
- Inclusivity: Engaging diverse voices in the development process ensures that AI serves all segments of society.
In conclusion, ethical frameworks are not just guidelines; they are the backbone of responsible AI development. They help bridge the gap between technological advancement and societal values, ensuring that AI technologies enhance human life rather than diminish it. As we continue to innovate, we must remain vigilant in our commitment to ethical principles, ensuring that our creations reflect the best of what humanity has to offer.
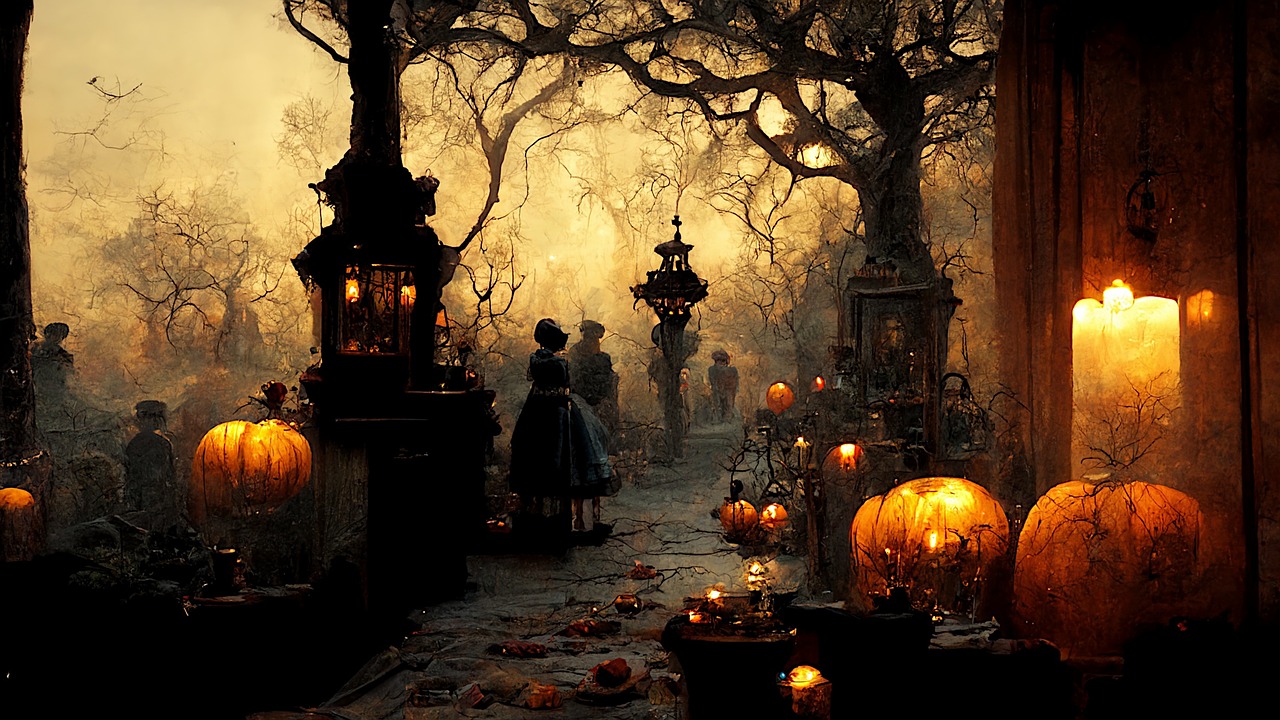
Challenges in Ethical AI Implementation
As we dive into the realm of artificial intelligence, it’s crucial to recognize the challenges that come with ensuring ethical standards are upheld. Despite the growing emphasis on ethics in AI, various hurdles can obstruct the effective implementation of these principles. One of the most daunting challenges is the prevalence of bias in AI systems. Bias can seep into algorithms from many sources, including the data used for training and the frameworks that guide their development. When AI systems are trained on historical data that reflect societal inequalities, they can inadvertently perpetuate those biases, leading to unfair outcomes that affect marginalized groups disproportionately.
Another significant challenge is the lack of transparency in AI processes. Many AI algorithms operate as "black boxes," where the decision-making process is not visible or understandable to users or stakeholders. This opacity can lead to distrust, as people may feel uncomfortable with systems that make decisions affecting their lives without clear explanations. Imagine trying to navigate a maze blindfolded; that’s how it feels for users trying to understand AI-driven decisions. Without transparency, accountability is also compromised, making it difficult to hold organizations responsible for the outcomes produced by their AI systems.
The rapid pace of technological advancement adds another layer of complexity. As AI technologies evolve, ethical frameworks struggle to keep up. This lag can lead to a disconnect between what is technologically feasible and what is ethically acceptable. For instance, consider how quickly social media algorithms have developed. They can influence public opinion and behavior in ways that were unimaginable just a few years ago. As a result, organizations may rush to deploy new technologies without thoroughly considering their ethical implications, which can lead to unintended consequences.
To summarize, the challenges in ethical AI implementation can be categorized as follows:
- Bias in AI Systems: Unfair outcomes due to biased training data and algorithms.
- Lack of Transparency: Difficulty in understanding AI decision-making processes.
- Rapid Technological Advancement: Ethical frameworks lagging behind technology development.
Addressing these challenges requires a concerted effort from all stakeholders involved in AI development. By recognizing and tackling these issues head-on, we can work towards creating AI systems that are not only innovative but also ethically sound and socially responsible.
- What is bias in AI? Bias in AI refers to the presence of prejudiced outcomes in AI systems, often stemming from biased training data or design choices.
- Why is transparency important in AI? Transparency is vital for accountability and trust, allowing users to understand how decisions are made by AI systems.
- How can organizations address ethical challenges in AI? Organizations can implement robust ethical frameworks, engage stakeholders, and continuously monitor AI systems to ensure they align with ethical standards.
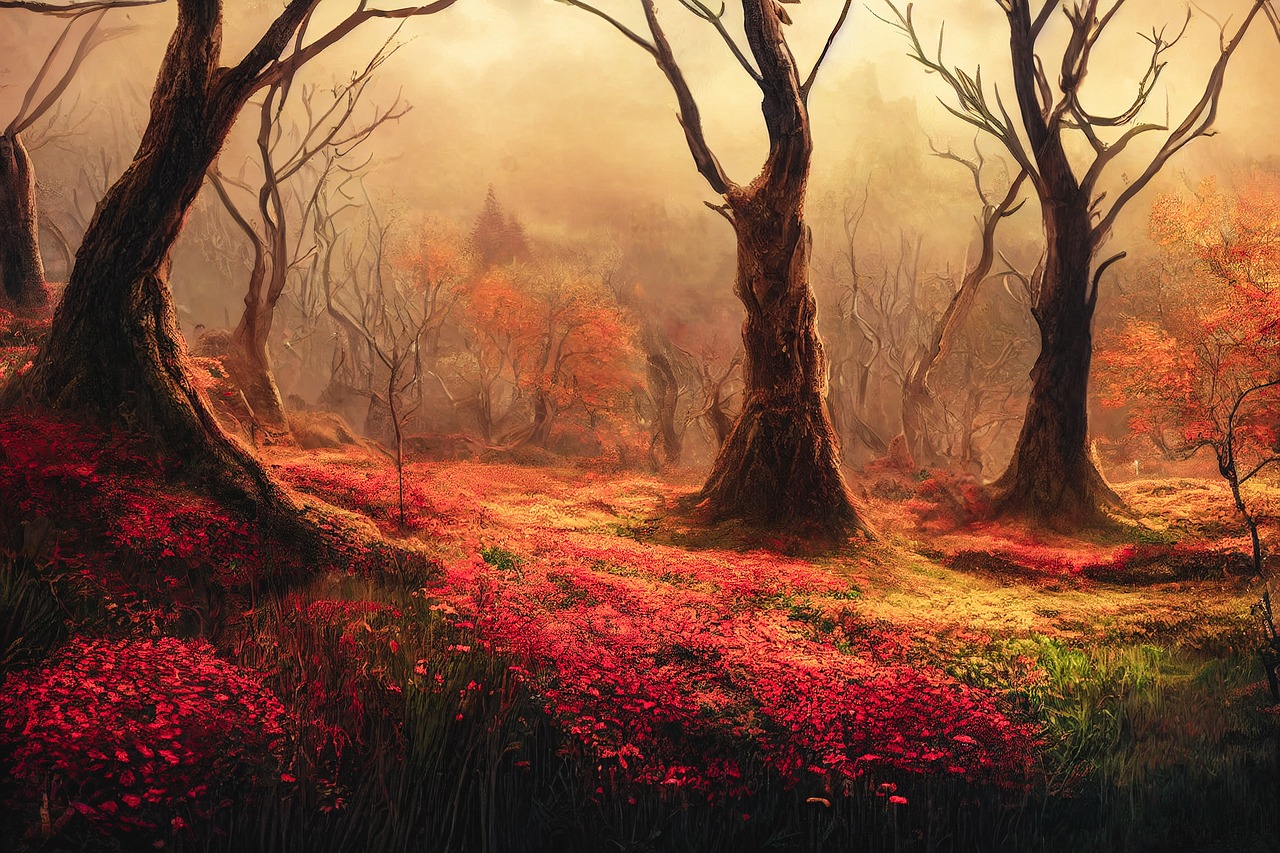
Addressing Bias in AI Systems
Bias in AI systems is a critical issue that has garnered significant attention in recent years. When we talk about bias, we’re often referring to the unfair treatment of individuals based on race, gender, age, or other characteristics. Imagine a world where an AI system decides whether you qualify for a loan based on biased historical data. This could lead to unfair outcomes that perpetuate existing inequalities, leaving marginalized groups at a disadvantage. It’s essential to identify and mitigate these biases to develop fair and equitable AI applications that serve diverse populations.
One of the primary reasons bias exists in AI is due to the data used to train these systems. If the training data reflects historical inequalities, the AI will likely learn and replicate those biases. For instance, if an AI system is trained on data that predominantly features one demographic, it might not perform well for others. This is why diversity in training data is crucial. By including a variety of perspectives and backgrounds in the datasets, we can help ensure that AI systems are more representative and just, ultimately leading to outcomes that benefit everyone.
Moreover, addressing bias in AI systems requires a multifaceted approach. Here are some techniques that can be employed:
- Algorithmic Adjustments: Modifying the algorithms to account for identified biases can help in reducing their impact. This can include techniques like re-weighting outcomes based on demographic factors.
- Diverse Training Data: Actively seeking out and incorporating diverse datasets can help in minimizing bias. This could involve collecting data from underrepresented groups to ensure a more balanced perspective.
- Regular Audits: Conducting routine audits of AI systems can help identify biases that may not be immediately apparent. This proactive approach allows organizations to address issues before they become systemic.
Case studies provide valuable insights into the real-world implications of bias in AI. For example, a well-documented case involved a hiring algorithm that favored male candidates over female candidates due to biased training data. This incident highlighted the importance of scrutinizing AI systems and reinforced the need for implementing effective solutions to address these biases. By learning from such examples, organizations can better understand the potential pitfalls of AI and work towards developing fairer systems.
Ultimately, addressing bias in AI systems is not just about correcting the technology; it’s about fostering a culture of responsibility and ethics in AI development. By prioritizing fairness, transparency, and accountability, we can create AI applications that truly serve the needs of all users, paving the way for a more equitable digital future.
- What is bias in AI? Bias in AI refers to the tendency of AI systems to produce unfair outcomes based on prejudiced data or algorithms.
- Why is it important to address bias in AI? Addressing bias is crucial to ensure fairness and equality in AI applications, as biased systems can perpetuate existing inequalities.
- How can organizations mitigate bias in AI? Organizations can mitigate bias through diverse training data, algorithmic adjustments, and regular audits of their AI systems.
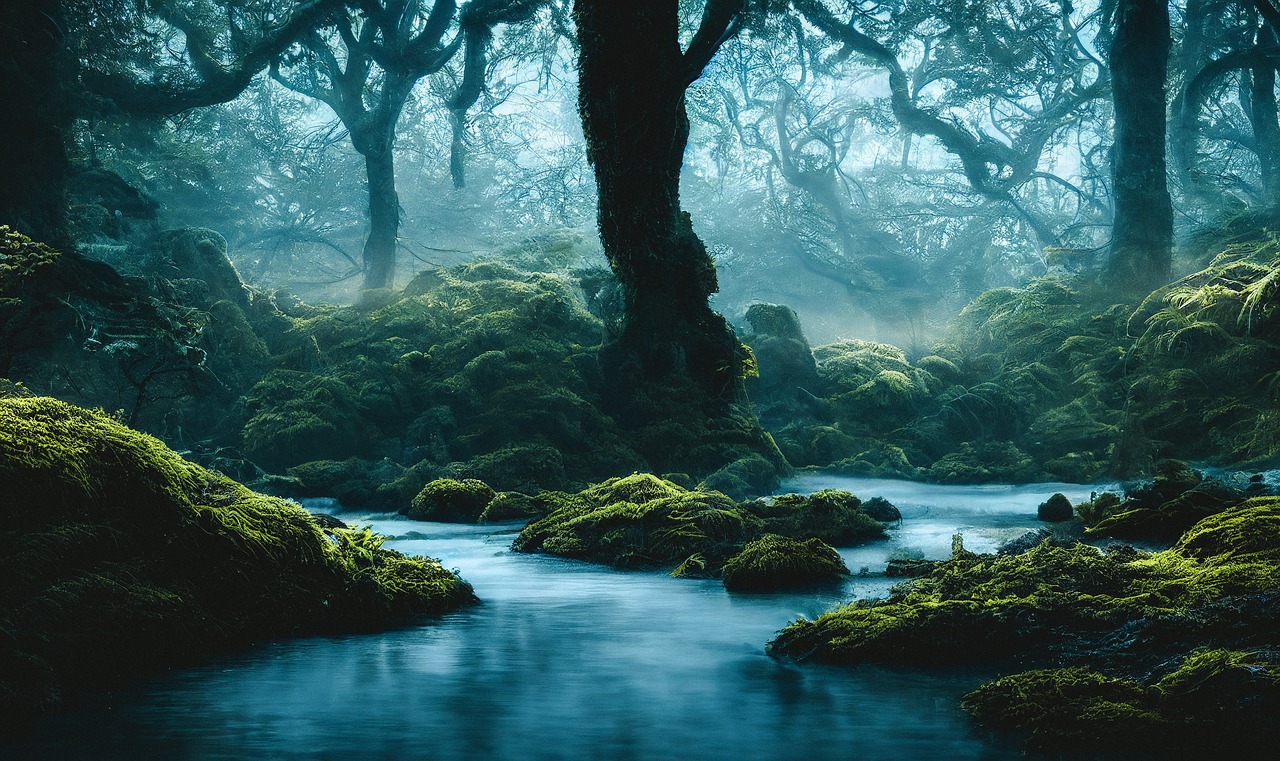
Techniques for Bias Mitigation
When it comes to ensuring fairness in AI systems, bias mitigation is not just an option; it's a necessity. Bias can creep into AI models through various channels, including biased training data, flawed algorithms, or even the subjective decisions of developers. To combat this, several techniques can be employed, each designed to enhance the fairness and accuracy of AI applications. One of the most effective ways to combat bias is through the use of algorithmic adjustments. This involves tweaking the algorithms to account for known biases, allowing the system to make more equitable decisions.
Another critical technique is the incorporation of diverse training data. By ensuring that the data used to train AI models is representative of the diverse populations they serve, we can significantly reduce the risk of biased outcomes. For instance, if an AI system is designed to analyze facial recognition, using a dataset that includes a wide range of ethnicities, genders, and ages will help the model learn to recognize features across different demographics accurately. This approach not only improves the model's performance but also builds trust among users who may have previously felt marginalized by technology.
In addition to these methods, regular audits of AI systems are essential. Conducting audits allows organizations to identify and address biases that may have slipped through the cracks during development. These audits can be performed by internal teams or outsourced to third-party experts who specialize in ethical AI practices. By implementing a systematic approach to auditing, organizations can ensure that their AI applications evolve alongside societal norms and expectations.
Furthermore, feedback loops play a pivotal role in bias mitigation. Establishing a mechanism for users to report issues or provide feedback on AI outputs can help developers understand real-world implications of their systems. This continuous feedback can inform necessary adjustments, making the AI more responsive to the needs of its users. For example, if users notice that an AI-driven hiring tool consistently overlooks qualified candidates from certain backgrounds, this feedback can trigger a review of the underlying algorithms and data.
To summarize, the journey towards bias mitigation in AI is multifaceted and requires a combination of techniques. From algorithmic adjustments and diverse training data to regular audits and user feedback loops, each method contributes to a more equitable AI ecosystem. By prioritizing these strategies, organizations can not only enhance the fairness of their AI applications but also foster a culture of accountability and trust.
- What is bias in AI? Bias in AI refers to systematic and unfair discrimination against certain individuals or groups, often arising from biased training data or flawed algorithms.
- How can I identify bias in my AI system? You can identify bias by conducting audits, analyzing outcomes across different demographics, and gathering user feedback.
- What are the consequences of biased AI? Biased AI can lead to unfair treatment of individuals, perpetuate existing inequalities, and damage trust in AI technologies.
- How often should I audit my AI systems? Regular audits should be conducted at least annually, or more frequently if significant changes are made to the system or its data.
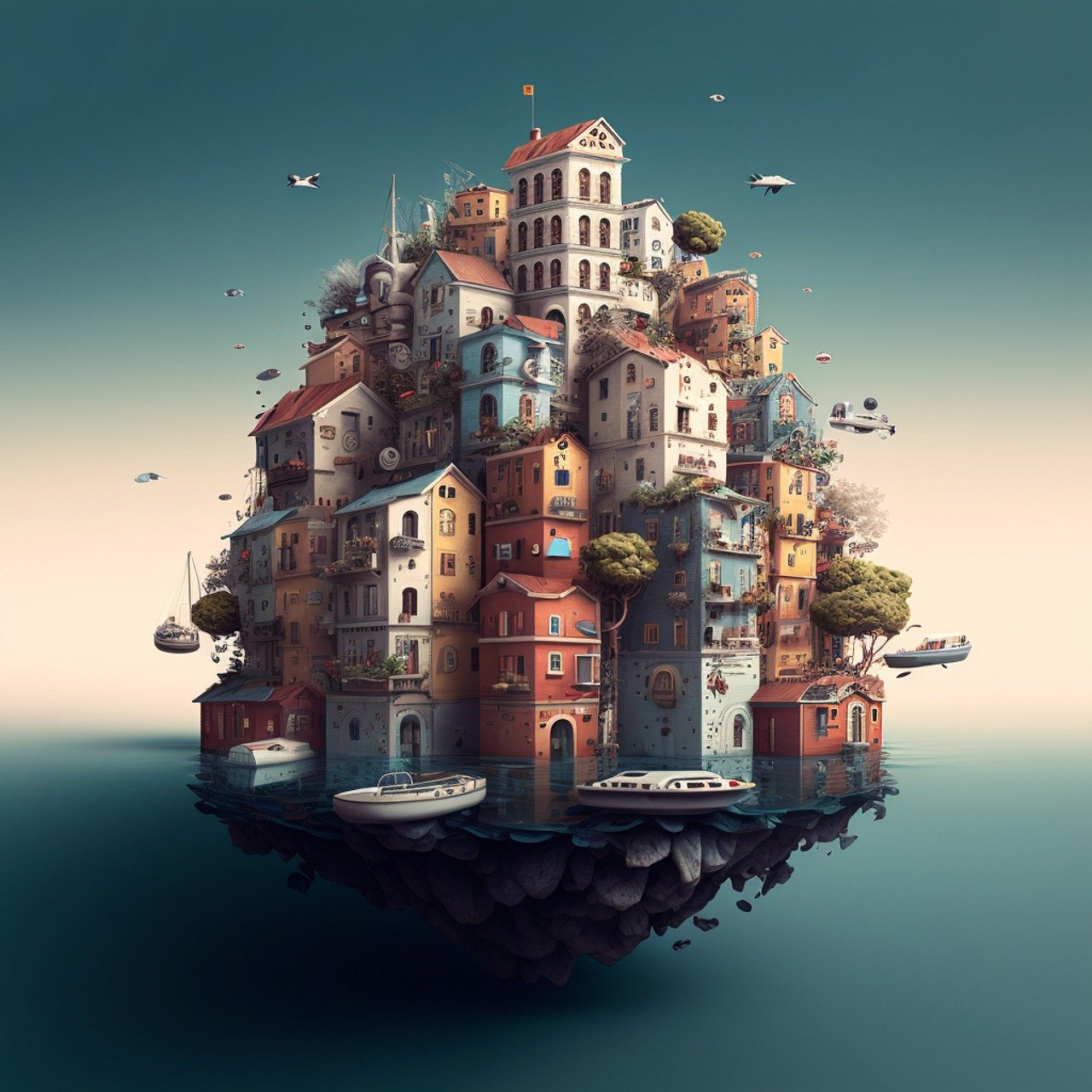
Case Studies on Bias
Understanding the impact of bias in AI systems is crucial for developing ethical applications. Several case studies highlight the real-world consequences of biased algorithms, showcasing the urgent need for intervention and reform. One notable example is the facial recognition technology used by law enforcement agencies. Studies have shown that these systems often misidentify individuals from minority groups at disproportionately higher rates compared to their white counterparts. This not only raises ethical concerns but also leads to potential wrongful arrests and loss of trust in law enforcement.
Another significant case is the use of AI in hiring processes. Companies have increasingly turned to algorithms to screen resumes and identify the best candidates. However, if these algorithms are trained on historical hiring data that reflects past biases, they can perpetuate discrimination against women and minority applicants. For instance, a well-known tech company faced backlash when it was revealed that its AI recruitment tool favored male candidates over female candidates due to biased training data. This incident illustrates how bias can seep into AI systems, affecting career opportunities and perpetuating gender inequality.
Moreover, the healthcare sector is not immune to these issues. AI systems designed to predict patient outcomes or recommend treatments have been shown to exhibit racial biases. A study revealed that an algorithm used in healthcare disproportionately favored white patients over black patients when allocating resources, leading to disparities in treatment quality. This not only highlights the ethical implications of biased AI but also raises questions about the fairness of healthcare delivery.
These case studies serve as a wake-up call for developers and organizations to prioritize fairness and equity in their AI systems. By examining these instances where bias has led to negative outcomes, stakeholders can identify the gaps in their current practices and implement necessary changes. Addressing these biases is not just about correcting past mistakes; it's about ensuring a more just and equitable future for all.
- What is bias in AI? Bias in AI refers to the systematic favoritism or discrimination that can occur in algorithms, often as a result of biased training data or flawed model design.
- How can bias in AI systems be identified? Bias can be identified through rigorous testing and evaluation of AI outputs across different demographic groups to ensure fairness and representation.
- What are some common techniques for mitigating bias? Techniques include using diverse training datasets, implementing fairness constraints in algorithms, and conducting regular audits of AI systems.
- Why is transparency important in AI? Transparency allows stakeholders to understand how decisions are made, which fosters trust and accountability in AI applications.
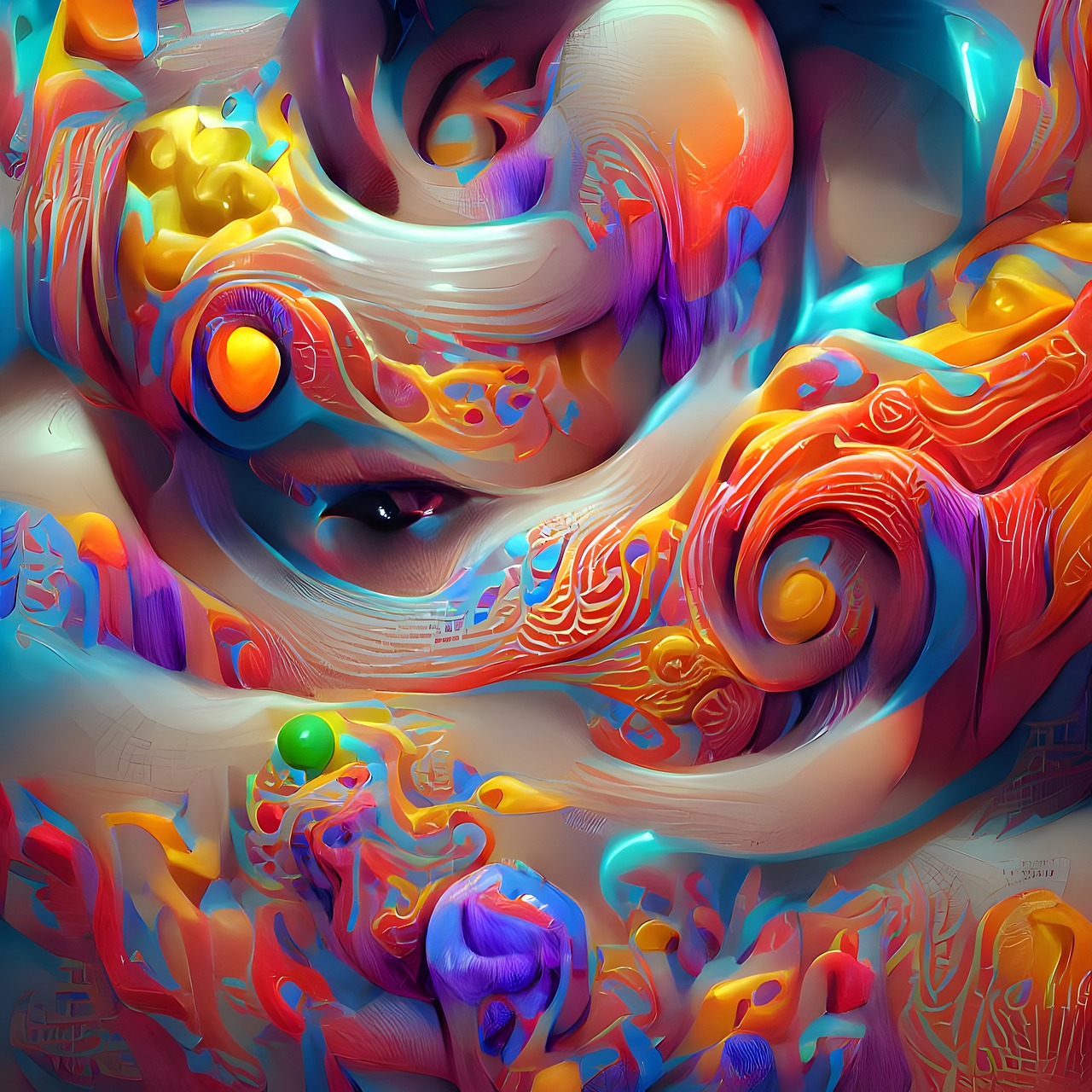
Ensuring Transparency and Accountability
Transparency in AI processes is not just a buzzword; it is a crucial element that defines the trustworthiness of artificial intelligence systems. When we talk about transparency, we are referring to the ability of stakeholders—including users, developers, and regulators—to understand how AI systems make decisions. Imagine driving a car without a clear view of the road ahead; that’s what using a non-transparent AI feels like. Users are left in the dark, unsure of how their data is being used or how decisions are being made. This lack of clarity can lead to skepticism and fear, which can ultimately stifle innovation and adoption of AI technologies.
To foster trust, AI developers must implement practices that promote transparency. This can involve several strategies, such as providing clear documentation of algorithms, making data sources available for scrutiny, and employing explainable AI techniques that clarify how decisions are reached. For instance, if an AI system denies a loan application, it should be able to explain the reasoning behind that decision in understandable terms. This not only helps the user comprehend the outcome but also ensures that the developers are held accountable for their technology.
Moreover, accountability goes hand in hand with transparency. When AI systems operate without oversight, there is a risk of misuse or unintended consequences. Organizations must establish clear lines of accountability, which means identifying who is responsible for the AI's performance and its impacts on users and society. This could involve creating an ethics board that reviews AI projects or appointing a chief ethics officer to oversee compliance with ethical standards.
In the realm of AI, accountability can also be enhanced through regular audits and assessments. These evaluations can help organizations identify potential ethical issues and rectify them before they escalate. For instance, an AI system used in hiring practices should be regularly reviewed to ensure it is not inadvertently discriminating against certain groups. By being proactive rather than reactive, companies can build a culture of responsibility around their AI technologies.
In summary, ensuring transparency and accountability in AI applications is essential for fostering trust and promoting ethical practices. By adopting clear communication strategies, establishing accountability measures, and conducting regular evaluations, organizations can not only enhance user confidence but also align their AI technologies with societal values. The journey towards ethical AI is ongoing, but with commitment and diligence, we can create systems that serve everyone fairly and justly.
- What is the role of transparency in AI?
Transparency helps users understand how AI systems make decisions, fostering trust and accountability. - How can organizations ensure accountability in AI?
Organizations can establish clear lines of responsibility, conduct regular audits, and create ethics boards to oversee AI practices. - Why is bias a concern in AI systems?
Bias can lead to unfair outcomes, perpetuating inequalities and affecting the credibility of AI applications. - What are explainable AI techniques?
Explainable AI techniques allow users to understand the reasoning behind AI decisions, making the technology more accessible and trustworthy.

Best Practices for Ethical AI Development
In the rapidly evolving world of artificial intelligence, it's imperative for organizations to adopt best practices that ensure ethical considerations are at the forefront of AI development. This isn't just about compliance; it's about fostering a culture of responsibility and trust. By aligning AI technologies with ethical standards, organizations can promote responsible innovation that safeguards user rights and societal interests.
One of the most effective ways to ensure ethical AI development is through stakeholder engagement. This involves actively involving users, ethicists, and policymakers in the development process. By gathering diverse perspectives, organizations can better understand the ethical expectations and community needs that their AI applications must meet. Imagine building a house without consulting an architect; the result might not be livable. Similarly, failing to engage with stakeholders can lead to AI systems that don't resonate with the very people they are designed to serve.
Another critical practice is the implementation of continuous monitoring and evaluation of AI systems. AI is not a set-it-and-forget-it technology; it requires ongoing scrutiny to ensure that it remains aligned with established ethical frameworks. This could involve regular audits, user feedback loops, and performance assessments. Just like a car needs regular maintenance to keep running smoothly, AI systems must be continuously evaluated to identify any ethical concerns that may arise over time.
Furthermore, organizations should consider adopting a transparent approach to AI development. Transparency is vital for accountability, allowing stakeholders to understand how decisions are made. This not only fosters trust but also encourages a culture of openness where ethical concerns can be raised and addressed promptly. For instance, if an AI system is used for hiring, providing clarity on how it evaluates candidates can help mitigate fears of bias and discrimination.
Additionally, organizations can benefit from creating a comprehensive ethical guideline document that outlines their commitment to ethical AI practices. This document should be easily accessible and regularly updated to reflect new insights and technological advancements. By having a clear reference point, both developers and stakeholders can stay aligned on the ethical standards that guide their work.
To summarize, the best practices for ethical AI development include:
- Engaging stakeholders to gather diverse perspectives.
- Implementing continuous monitoring and evaluation of AI systems.
- Adopting a transparent approach to foster trust and accountability.
- Creating comprehensive ethical guidelines that are regularly updated.
By embedding these practices into the core of AI development, organizations can not only mitigate risks associated with unethical practices but also pave the way for innovations that are both socially responsible and beneficial. In the end, ethical AI isn't just a checkbox to tick; it's a commitment to creating technology that uplifts and empowers all members of society.
Q1: What are the key components of ethical AI development?
A1: Key components include stakeholder engagement, continuous monitoring, transparency, and comprehensive ethical guidelines.
Q2: Why is stakeholder engagement important in AI development?
A2: Engaging stakeholders helps gather diverse perspectives, ensuring that AI applications meet ethical expectations and community needs.
Q3: How can organizations ensure transparency in AI?
A3: Organizations can ensure transparency by clearly communicating how AI systems make decisions and by providing accessible information to stakeholders.
Q4: What role does continuous monitoring play in ethical AI?
A4: Continuous monitoring helps identify and address ethical concerns, ensuring that AI systems remain aligned with established ethical frameworks.
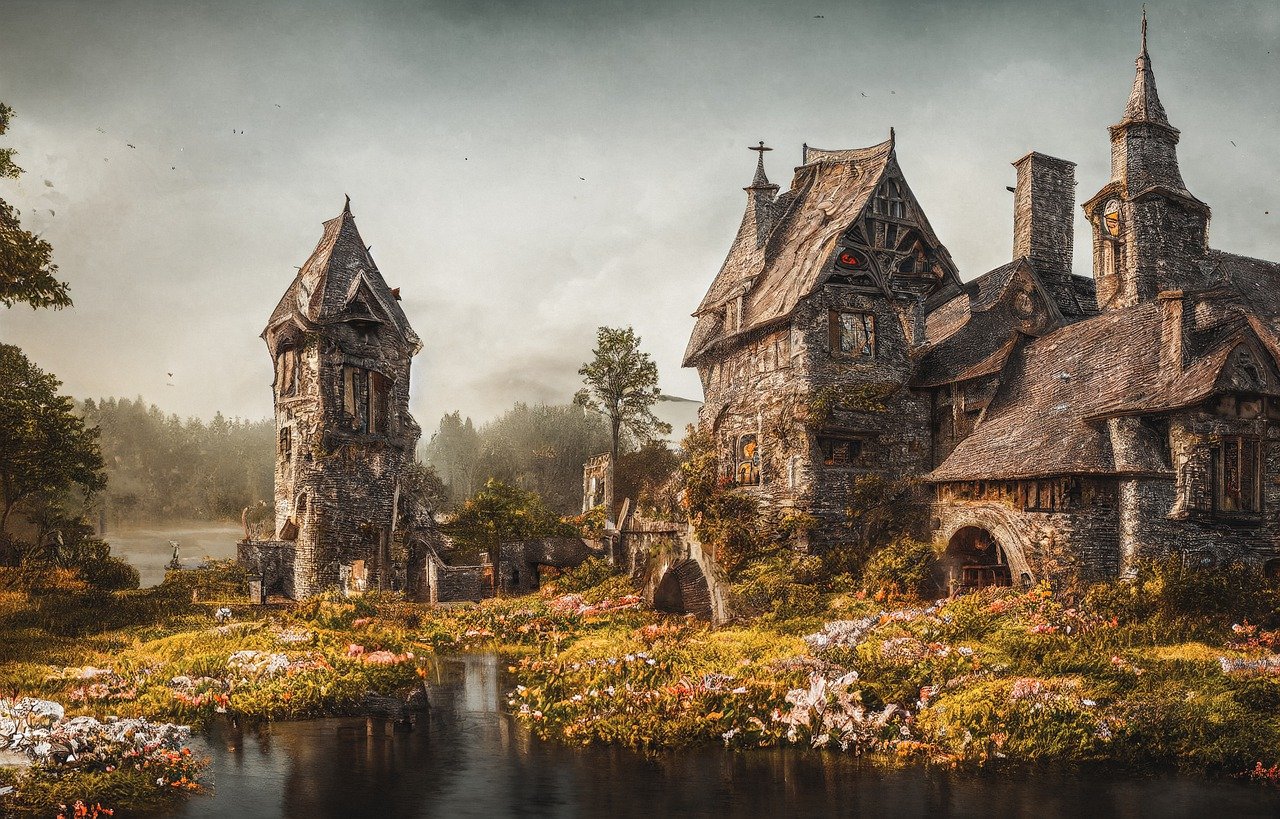
Stakeholder Engagement
Engaging stakeholders in the development of AI technologies is not just a checkbox exercise; it's a fundamental aspect that can significantly shape the ethical landscape of AI applications. When we talk about stakeholders, we’re referring to a diverse group that includes users, ethicists, policymakers, and even the communities that these technologies will impact. By involving these parties early in the design and implementation phases, organizations can gain invaluable insights that reflect a broader spectrum of values and concerns. Think of it like throwing a party: if you want everyone to have a good time, you need to consider the preferences of all your guests, not just your own.
One of the most compelling reasons for stakeholder engagement is the **diversity of perspectives** it brings to the table. For instance, users from different backgrounds may have unique needs and expectations from AI systems, and failing to recognize these differences can lead to products that are either irrelevant or harmful. Engaging with ethicists can help organizations navigate the murky waters of moral dilemmas that arise in AI deployment, while policymakers can provide guidance on legal and regulatory frameworks that must be adhered to. This collective input not only fosters a sense of **ownership** among stakeholders but also enhances the overall quality and acceptance of AI applications.
Furthermore, stakeholder engagement can serve as a **feedback loop**, allowing organizations to refine their technologies based on real-world experiences and concerns. For example, if a community feels that an AI system is perpetuating stereotypes or biases, their feedback can lead to immediate changes in the algorithm or its deployment strategy. This iterative approach not only improves the technology but also builds trust and accountability, as stakeholders see their voices being heard and their concerns addressed.
To effectively engage stakeholders, organizations can adopt several strategies:
- **Workshops and Focus Groups**: These can be organized to facilitate open discussions where stakeholders can share their thoughts and experiences related to AI applications.
- **Surveys and Questionnaires**: Collecting quantitative data can help gauge public sentiment and identify key areas of concern that need addressing.
- **Public Forums**: Hosting community meetings allows for broader participation and can highlight issues that may not be apparent in smaller, controlled settings.
In summary, stakeholder engagement is not merely an ethical obligation; it is a strategic advantage. By weaving together the insights and expectations of various stakeholders, organizations can create AI technologies that are not only effective but also ethically sound and socially responsible. As we move forward in this rapidly evolving field, it’s clear that the voices of those impacted by AI must be at the forefront of development and deployment strategies.
Q1: Why is stakeholder engagement important in AI development?
A1: Stakeholder engagement is crucial because it brings diverse perspectives to the table, ensuring that AI technologies meet the needs and expectations of various groups, thereby fostering trust and accountability.
Q2: Who are considered stakeholders in AI?
A2: Stakeholders include users, ethicists, policymakers, community members, and anyone else who may be affected by the deployment of AI technologies.
Q3: How can organizations effectively engage stakeholders?
A3: Organizations can engage stakeholders through workshops, surveys, public forums, and focus groups to gather feedback and insights that inform AI development.
Q4: What are the benefits of involving stakeholders in AI design?
A4: Involving stakeholders can lead to better technology that is more aligned with societal values, increased trust, and a sense of ownership among those affected by AI applications.

Continuous Monitoring and Evaluation
In the fast-paced world of artificial intelligence, are not just optional; they are essential. Imagine a ship navigating through turbulent waters without a compass or a map. It would be reckless, right? Well, that’s exactly what it’s like for AI systems that lack ongoing scrutiny. Continuous monitoring ensures that AI applications remain aligned with ethical standards, societal norms, and user expectations over time. It’s about maintaining a dynamic relationship between technology and the people it serves.
One of the primary reasons for this ongoing evaluation is the evolving nature of AI technologies. As algorithms learn and adapt, they can unintentionally drift away from their intended ethical frameworks. This drift can lead to unforeseen consequences, such as biased outcomes or violations of user privacy. Therefore, organizations must implement robust monitoring mechanisms that not only track performance but also assess ethical compliance. This involves regularly reviewing the data being used, the decisions being made, and the impact those decisions have on users and society as a whole.
To facilitate this process, organizations can adopt a structured approach to monitoring and evaluation. Here are some key components that should be included:
- Regular Audits: Conducting periodic audits of AI systems helps identify any ethical lapses or biases that may have emerged since the last evaluation.
- User Feedback: Actively seeking feedback from users can provide insights into how AI applications are perceived and whether they meet ethical standards.
- Performance Metrics: Establishing clear metrics for success that include ethical considerations ensures that AI systems are evaluated holistically.
Moreover, it’s crucial to create a culture of transparency within organizations. When stakeholders understand how AI systems operate and how decisions are made, they are more likely to trust these technologies. This transparency can be achieved through open communication about the algorithms used, the data sources, and the rationale behind decision-making processes. By fostering an environment where questions and concerns can be raised without fear, organizations can better align their AI applications with ethical expectations.
In addition, organizations should establish a dedicated team responsible for the continuous monitoring and evaluation of AI systems. This team should comprise individuals with diverse backgrounds, including ethicists, data scientists, and user experience experts. By bringing together a variety of perspectives, the team can more effectively identify potential ethical issues and propose solutions that are both innovative and responsible.
Finally, as technologies evolve, so too should the frameworks and practices surrounding them. This means that continuous monitoring and evaluation are not static processes but rather ongoing commitments to ethical integrity. Organizations should be prepared to adapt their practices in response to new developments in AI and emerging societal expectations. By doing so, they can ensure that their AI applications not only perform well but also contribute positively to society.
- What is the purpose of continuous monitoring in AI? Continuous monitoring helps ensure that AI systems remain aligned with ethical standards and societal norms, preventing biases and maintaining user trust.
- How can organizations implement effective monitoring practices? Organizations can implement regular audits, gather user feedback, and establish clear performance metrics that include ethical considerations.
- Why is transparency important in AI systems? Transparency fosters trust and accountability, allowing stakeholders to understand how decisions are made and ensuring ethical compliance.
- Who should be involved in the monitoring process? A dedicated team comprising ethicists, data scientists, and user experience experts should oversee the continuous monitoring and evaluation of AI systems.
Frequently Asked Questions
- What are ethical frameworks in AI?
Ethical frameworks in AI are structured guidelines that help developers and organizations align their AI applications with societal values and norms. They are essential for ensuring that AI technologies are developed responsibly, fostering trust and accountability among users and stakeholders.
- Why is addressing bias in AI systems important?
Addressing bias in AI systems is crucial because it helps prevent unfair outcomes that can perpetuate existing inequalities. By identifying and mitigating biases, we can create fairer and more equitable AI applications that serve the needs of diverse populations.
- What techniques can be used to mitigate bias in AI?
There are several techniques to mitigate bias in AI, including algorithmic adjustments, the use of diverse training data, and implementing fairness-aware algorithms. These methods help ensure that AI outcomes are more representative and just for all users.
- How does transparency contribute to ethical AI?
Transparency in AI processes is vital for accountability. It allows stakeholders to understand how decisions are made, which fosters trust in AI applications. When users know how an AI system operates, they are more likely to feel comfortable and secure in its use.
- What are some best practices for ethical AI development?
Best practices for ethical AI development include engaging stakeholders, such as users and policymakers, continuously monitoring and evaluating AI systems, and adhering to established ethical frameworks. These practices promote responsible innovation while safeguarding user rights and societal interests.
- How can stakeholder engagement improve AI applications?
Engaging stakeholders provides diverse perspectives that can help shape AI applications to meet ethical expectations and community needs. Involving users, ethicists, and policymakers ensures that AI technologies are more aligned with societal values.
- Why is continuous monitoring necessary for AI systems?
Continuous monitoring of AI systems is necessary to identify ethical concerns and adapt practices accordingly. This ongoing evaluation ensures that AI applications remain aligned with established ethical frameworks over time, addressing any emerging issues promptly.