Ethical Considerations in AI Adoption: What to Know
As we dive into the realm of artificial intelligence (AI), it's essential to grasp the ethical considerations that accompany its adoption. AI is not just a technological advancement; it's a tool that can shape our future, influencing how we live, work, and interact. The implications of AI stretch far beyond mere efficiency and automation—they touch on fundamental issues of fairness, accountability, privacy, and the broader societal impact of these technologies. So, what does it really mean to adopt AI ethically? Let’s explore the key ethical issues that should be at the forefront of any conversation around AI technology.
AI ethics encompasses the moral implications of AI technology. It serves as a guiding framework to ensure that AI systems are developed and deployed responsibly. The foundational principles of ethical AI include transparency, fairness, and accountability. These principles help in addressing the potential pitfalls that can arise from AI adoption. For instance, without transparency, users may not understand how decisions are made by AI systems, leading to distrust and skepticism. Furthermore, ethical AI development seeks to prevent biases that can skew outcomes, ensuring that all users are treated fairly and equitably.
Fairness is a critical aspect of AI ethics. When we talk about fairness in AI, we are referring to the need for equitable algorithms that do not discriminate against any group. It's vital to recognize that biases can be introduced at various stages of AI system development, from data collection to algorithm design. If we’re not careful, we risk perpetuating existing inequalities rather than alleviating them. The question we must ask ourselves is: can we truly trust AI systems to make decisions that affect our lives if they are built on biased foundations?
There are several types of biases that can affect AI systems, including:
- Data Bias: This occurs when the data used to train AI models is unrepresentative or flawed. For example, if an AI system is trained primarily on data from one demographic, it may not perform well for others.
- Algorithmic Bias: This type of bias happens when the algorithms themselves favor certain outcomes over others, often due to the way they are programmed or the data they are trained on.
- Human Bias: Human biases can inadvertently influence AI systems, particularly during the design and implementation phases.
Understanding these biases is crucial for developing fair AI systems that serve all individuals equally.
Data bias arises when training datasets are unrepresentative or flawed. For instance, if an AI system is trained on data that predominantly reflects one demographic, it may fail to accurately represent or serve other groups. This section will explore how data collection methods can contribute to biased AI outcomes. It's like trying to bake a cake with only one ingredient—no matter how well you mix it, the final product won't be complete.
Algorithmic bias occurs when the algorithms themselves favor certain outcomes. This can happen for various reasons, including the way algorithms are designed or the assumptions made during their development. The consequences of algorithmic bias can be severe, leading to unfair treatment of individuals in critical areas such as hiring, lending, and law enforcement.
Strategies to mitigate bias in AI systems are crucial for ethical AI adoption. Techniques such as diverse data sampling, regular audits, and inclusive design practices can help ensure fairness in AI outcomes. It’s essential to adopt a proactive approach rather than a reactive one—addressing potential biases before they manifest in harmful ways.
Accountability is essential in AI deployment. As AI technologies become more integrated into our daily lives, the responsibilities of developers and organizations in ensuring ethical AI use grow significantly. Without accountability, it becomes challenging to hold anyone responsible for the consequences of AI decisions, leading to a potential erosion of trust.
Creating accountability frameworks helps delineate responsibilities and ensures that AI systems are used ethically. Various models for accountability exist, ranging from internal governance structures within organizations to external regulatory bodies. By establishing clear lines of responsibility, organizations can foster a culture of ethical AI use.
The legal landscape surrounding AI accountability is evolving. Current laws and potential regulations are beginning to address the unique challenges posed by AI technologies. Understanding these legal implications is crucial for developers and users alike, as failure to comply could result in significant penalties and reputational damage.
Privacy is a significant concern in AI adoption. As AI technologies become more sophisticated, they often require vast amounts of personal data to function effectively. This raises important questions about how this data is collected, used, and protected. Are we sacrificing our privacy in exchange for convenience? The answer to this question is a delicate balance between innovation and personal rights.
Understanding data collection practices is crucial for addressing privacy concerns. Many AI systems rely on personal data to provide tailored experiences, but this can lead to significant privacy risks if not managed correctly. Evaluating how data is gathered and used in AI systems is a critical step in ensuring ethical AI practices.
User consent and transparency are vital for ethical AI. It's essential to inform users about how their data will be used and to obtain their consent before data collection occurs. Transparency builds trust, and without it, users may feel exploited or manipulated.
AI technologies can profoundly affect society. The implications of AI adoption stretch beyond individual users, influencing economic, social, and cultural dynamics. As we embrace AI, we must consider how it reshapes our world and the potential consequences of these changes.
The rise of AI raises concerns about job displacement. Automation has the potential to replace many traditional jobs, leading to economic shifts that can affect livelihoods. While AI can create new opportunities, we must be vigilant about the impact on those whose jobs may be at risk.
AI technologies can influence social dynamics in various ways. From changing how we communicate to altering our relationships, the societal implications of AI are profound. As we navigate this new landscape, it's essential to consider how AI affects not just individuals but entire communities and cultures.
Q: What are the primary ethical concerns surrounding AI?
A: The main ethical concerns include fairness, accountability, and privacy issues, as well as the societal impact of AI technologies.
Q: How can bias in AI systems be addressed?
A: Bias can be mitigated through diverse data sampling, regular audits, and inclusive design practices to ensure fairness.
Q: Why is accountability important in AI?
A: Accountability ensures that developers and organizations take responsibility for the ethical use of AI, fostering trust and integrity in AI applications.
Q: What role does user consent play in AI?
A: User consent is essential for ethical AI, as it ensures that individuals are informed about how their data will be used and allows them to make informed decisions.
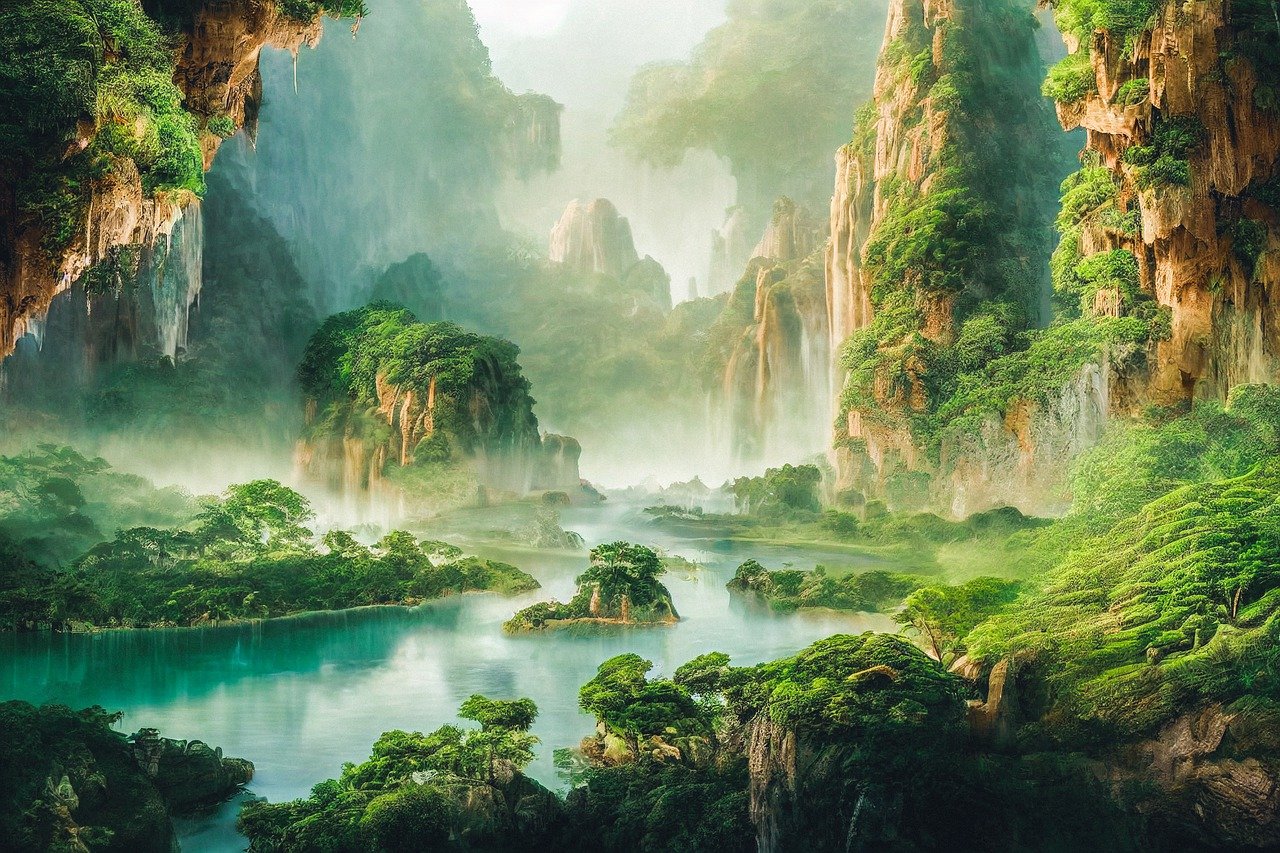
Understanding AI Ethics
When we dive into the world of artificial intelligence, it's like stepping into a vast ocean of possibilities and challenges. AI ethics encompasses the moral implications of AI technology, guiding us in navigating this complex landscape. It raises essential questions about how we should develop and deploy AI systems responsibly. Just like a compass helps sailors find their way, ethical principles serve as our guide in ensuring that AI serves humanity positively and equitably.
At the heart of AI ethics are several foundational principles that help shape our understanding of what it means to be ethical in this rapidly evolving field. These principles include:
- Fairness: Ensuring that AI systems do not perpetuate or exacerbate existing inequalities.
- Accountability: Establishing clear responsibilities for those who create and deploy AI technologies.
- Transparency: Making AI systems understandable and explainable to users and stakeholders.
- Privacy: Protecting individuals' data and ensuring their personal information is used responsibly.
Each of these principles plays a crucial role in guiding the ethical development of AI. For instance, fairness is not just about avoiding discrimination; it’s about actively working to create systems that promote equity. Imagine a world where AI systems are designed to uplift underrepresented communities rather than marginalize them. That’s the kind of future we should strive for!
Moreover, accountability is vital. When AI systems make decisions that impact people's lives—like hiring, lending, or even healthcare—there should be a clear understanding of who is responsible for those decisions. This is where frameworks come into play, helping to clarify roles and responsibilities in AI deployment.
Transparency is another cornerstone of AI ethics. Users should know how AI systems function and the data they rely on. Think about it: would you trust a black box that makes decisions about your life without understanding how it works? Transparency builds trust, and without it, skepticism about AI will only grow.
Lastly, privacy concerns are paramount in the age of big data. As AI technologies analyze vast amounts of personal information, ensuring that individuals' privacy is protected is non-negotiable. Organizations must be diligent in their data collection practices, ensuring that they respect user consent and maintain transparency about how data is used.
In conclusion, understanding AI ethics is not just about recognizing the challenges but also about embracing the responsibility that comes with innovation. As we continue to integrate AI into various aspects of our lives, it’s crucial to keep these ethical principles at the forefront. This way, we can harness the power of AI while safeguarding our values and ensuring a just society for all.
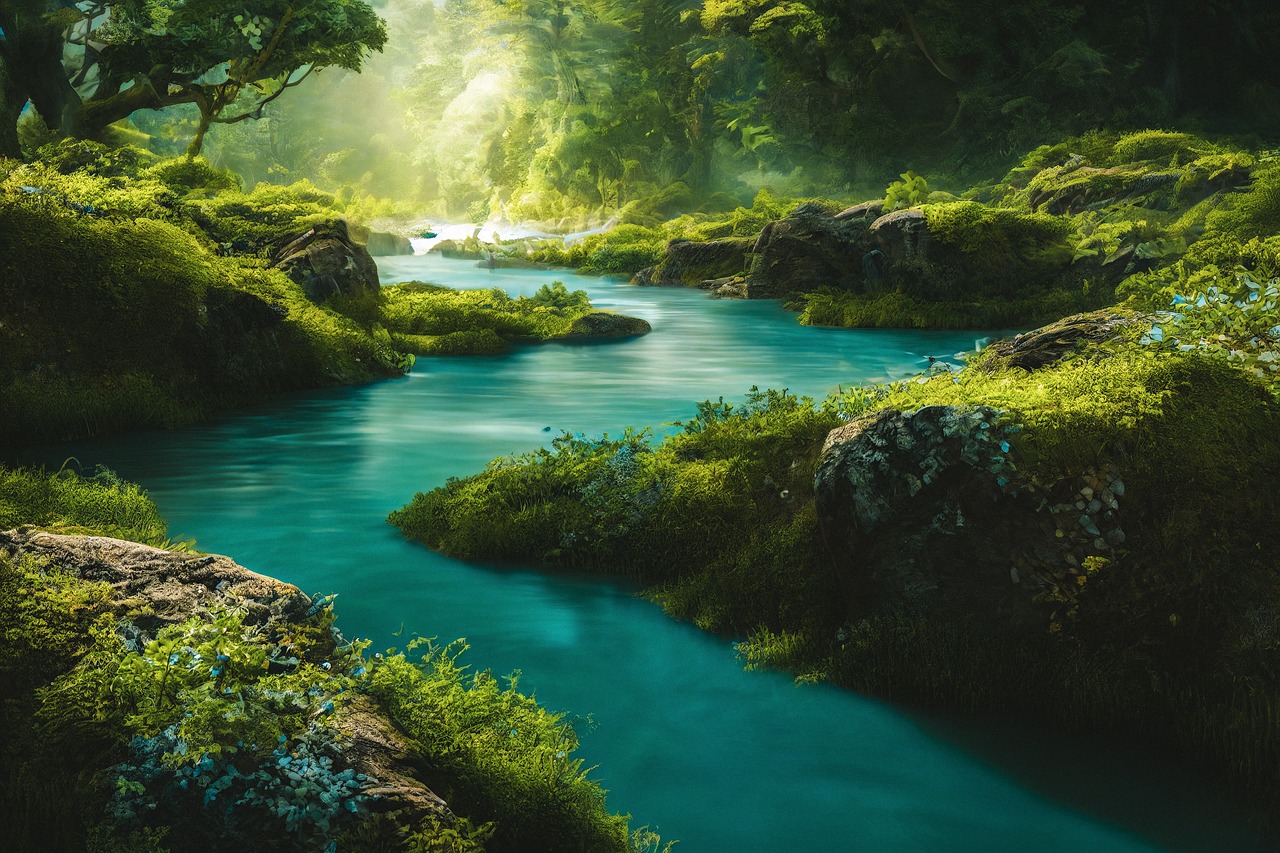
Fairness in AI
Fairness in artificial intelligence is not just a buzzword; it’s a fundamental principle that can make or break the impact of AI technologies on society. Imagine a world where AI systems are deployed in critical areas such as hiring, law enforcement, and lending, yet they carry the weight of biases that can lead to unfair treatment of individuals. This is why understanding fairness in AI is paramount. The stakes are high, and the implications of biased AI can ripple through communities, affecting lives in profound ways.
At its core, fairness in AI revolves around the idea that algorithms should treat all individuals equitably, without discrimination based on race, gender, age, or other attributes. However, achieving this ideal is easier said than done. AI systems are often trained on historical data, which may contain biases that reflect societal inequalities. If we’re not careful, these biases can be perpetuated or even amplified by the algorithms we create. So, how do we ensure that fairness is integrated into AI systems from the ground up?
One way to tackle fairness is by examining the various types of biases that can creep into AI systems. These biases can be categorized into three main types: data bias, algorithmic bias, and human bias. Each of these biases has its own set of implications for fairness:
- Data Bias: This occurs when the data used to train AI models is unrepresentative or flawed. For instance, if a facial recognition system is primarily trained on images of lighter-skinned individuals, it may perform poorly on individuals with darker skin tones, leading to discriminatory outcomes.
- Algorithmic Bias: Even if the data is fair, the algorithms themselves can introduce biases. For example, if an algorithm is designed to optimize for a specific outcome without considering fairness constraints, it may inadvertently favor certain groups over others.
- Human Bias: Finally, human bias can seep into AI systems through the choices made by developers. If the team creating an AI model lacks diversity, their unconscious biases may influence the design and functionality of the system.
Addressing these biases is crucial for creating fair AI systems. Strategies such as diversifying training datasets, implementing fairness-aware algorithms, and conducting regular audits can help mitigate the risks associated with bias. It’s not just about technology; it’s about fostering a culture of responsibility and awareness among AI developers and stakeholders. After all, the goal is to create AI that enhances human capabilities rather than undermines them.
In conclusion, fairness in AI is a complex yet essential topic that requires ongoing attention and action. As we continue to integrate AI into various aspects of our lives, we must prioritize the development of equitable algorithms that serve all members of society. By doing so, we can harness the power of AI while ensuring that it contributes positively to our communities.
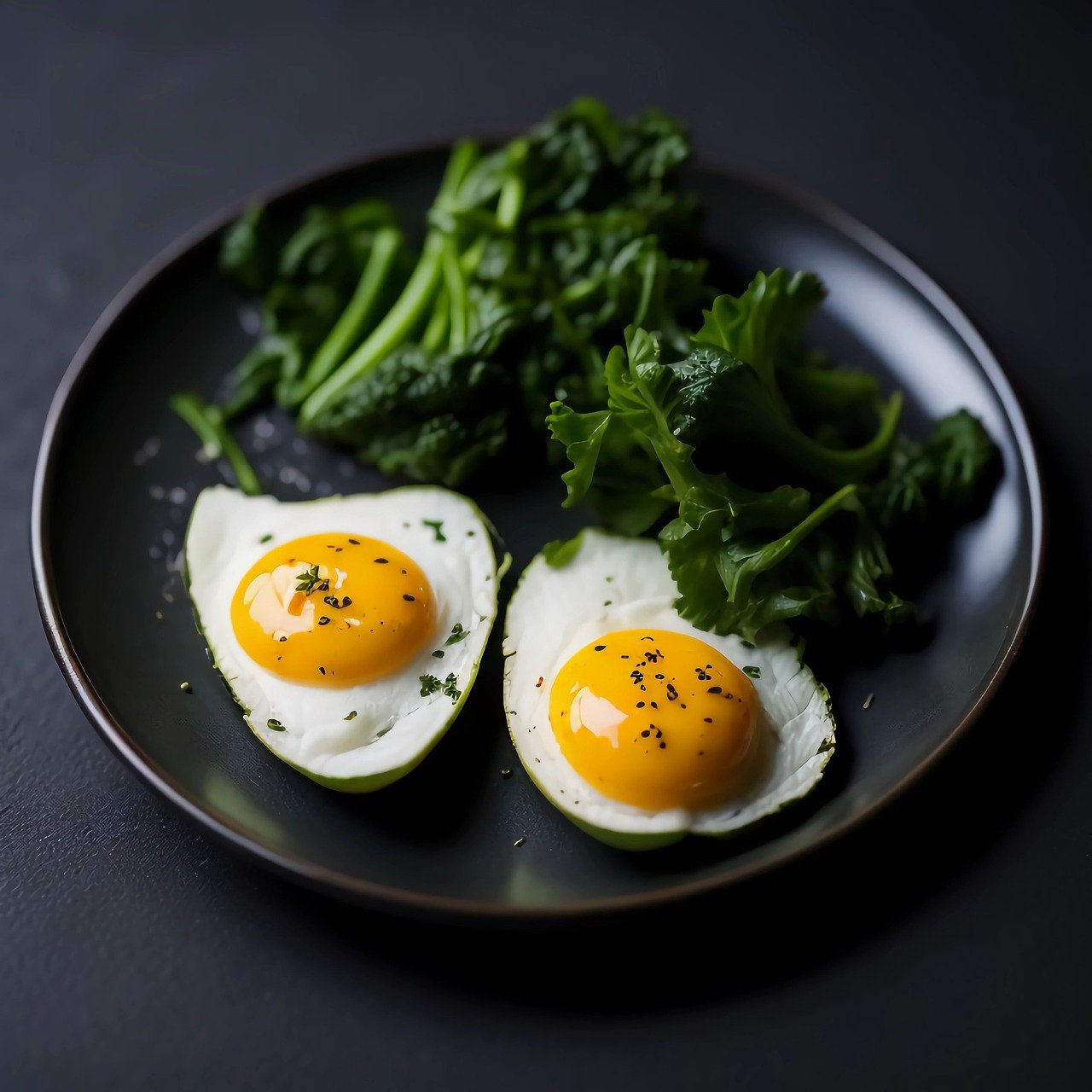
Types of Bias in AI
When we talk about bias in AI, it’s like peeling an onion—there are multiple layers, and each one reveals something different about how AI systems can behave unfairly. Bias can creep in at various stages of the AI development process, affecting everything from the data we use to train our models to the algorithms that process this data. Understanding the types of bias is crucial for creating fair and equitable AI systems.
One of the most prominent forms of bias is data bias. This occurs when the data used to train AI systems is unrepresentative or flawed. Imagine trying to teach a child about the world using only a limited set of experiences; the child would develop a skewed understanding of reality. Similarly, if an AI system is trained on data that doesn't accurately reflect the diversity of the population it serves, it can lead to decisions that are unfair or discriminatory. For instance, if a facial recognition system is trained primarily on images of light-skinned individuals, it may struggle to accurately identify people with darker skin tones.
Next, we have algorithmic bias, which arises from the way algorithms are designed and implemented. Even if the data is unbiased, the algorithm itself can favor certain outcomes over others. This can happen due to the choices made by developers or inherent flaws in the algorithm's logic. For example, if an algorithm is programmed to prioritize efficiency, it might inadvertently overlook important factors like user privacy or fairness. This is akin to a chef who focuses solely on speed in the kitchen, potentially sacrificing the quality of the dish.
Lastly, there's human bias, which is perhaps the most insidious type. Humans are naturally biased beings, and these biases can seep into AI systems during the design and development phases. Whether it’s through the selection of training data or the interpretation of results, human bias can skew the outcomes significantly. It’s like trying to build a house on a shaky foundation; no matter how well you construct the walls, the house will never be stable.
To sum it up, the types of bias in AI can be categorized into three main groups:
- Data Bias: Flawed or unrepresentative training datasets.
- Algorithmic Bias: Biases arising from the design and logic of algorithms.
- Human Bias: Influences from human decisions and interpretations during development.
Recognizing these biases is the first step toward addressing them. By understanding how they manifest, we can take proactive measures to mitigate their effects and ensure that AI technologies serve all segments of society fairly and equitably. In the next sections, we’ll delve into strategies for addressing these biases and the importance of establishing accountability in AI systems.
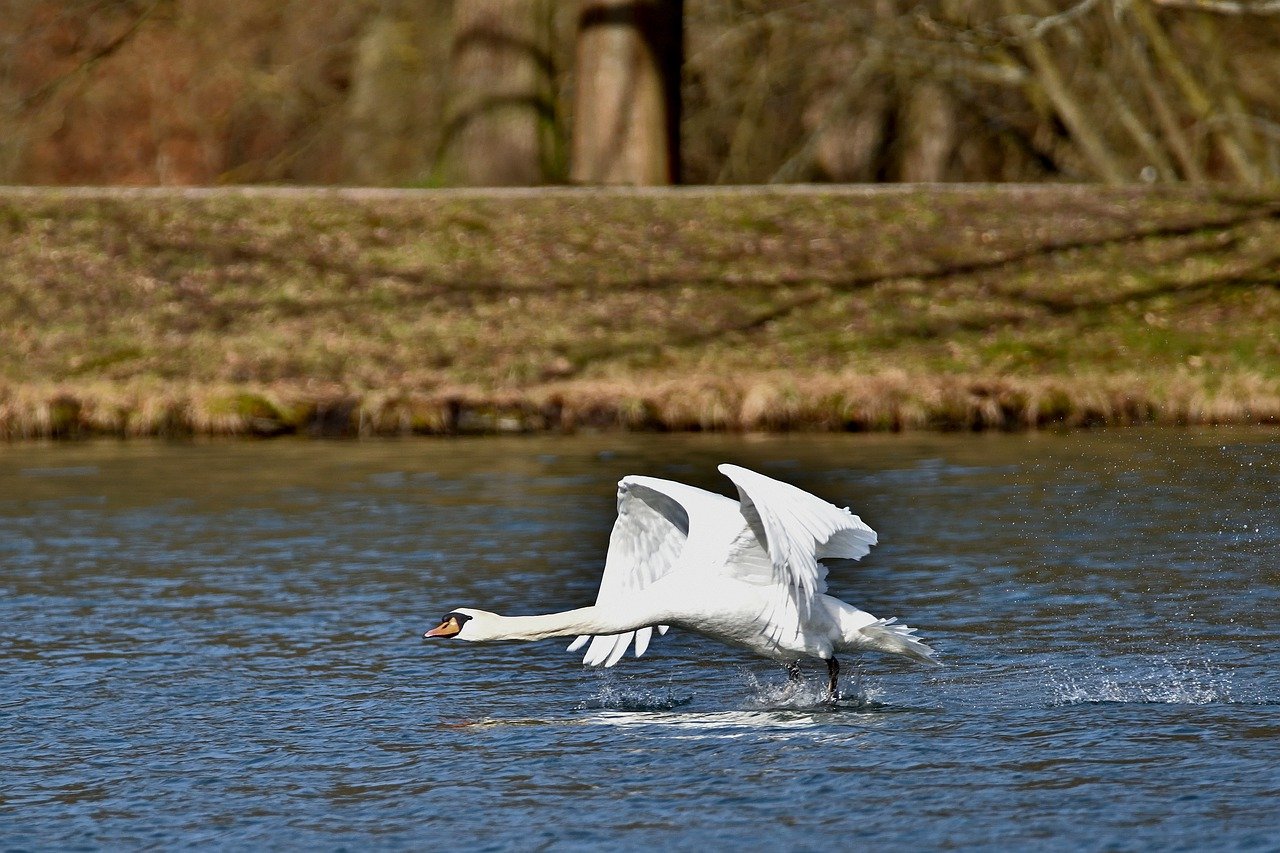
Data Bias
Data bias is a significant concern in the realm of artificial intelligence, as it arises when the datasets used to train AI models are unrepresentative or flawed. Imagine trying to bake a cake using a recipe that calls for ingredients that are either missing or of poor quality; the end result will likely be a disaster. Similarly, when AI systems are trained on biased or incomplete data, the outcomes can be skewed, leading to unfair or inaccurate results. This issue can manifest in various ways, affecting everything from hiring practices to criminal justice decisions.
One of the primary sources of data bias is the selection bias that occurs during the data collection process. If certain groups are overrepresented or underrepresented in the dataset, the AI model may develop a skewed understanding of reality. For instance, if a facial recognition system is trained predominantly on images of individuals from a specific demographic, it may struggle to accurately identify individuals from other backgrounds. This can lead to serious consequences, such as misidentification or lack of access to services for those who don't fit the model's narrow understanding.
Moreover, data bias can also arise from historical biases embedded in the data itself. For example, if an AI system is trained on historical hiring data that reflects past discrimination, it may perpetuate those biases in its decision-making processes. This creates a vicious cycle where AI systems reinforce existing inequalities rather than help to eliminate them. To illustrate the impact of data bias, consider the following table:
Type of Bias | Example | Potential Impact |
---|---|---|
Selection Bias | Facial recognition trained on predominantly white faces | Higher error rates for non-white individuals |
Historical Bias | AI hiring tool trained on biased historical data | Reinforcement of discriminatory hiring practices |
Measurement Bias | Inaccurate data collection methods | Skewed results affecting decision-making |
Addressing data bias is crucial for ensuring that AI systems operate fairly and effectively. This involves not only scrutinizing the data collection methods but also actively working to include diverse perspectives in the datasets used for training. By doing so, we can create more robust AI models that reflect the complexity of the real world.
In conclusion, understanding and mitigating data bias is essential for the ethical adoption of AI technologies. As we continue to integrate AI into various aspects of our lives, we must remain vigilant about the quality and representation of the data we use. After all, the integrity of AI systems hinges on the integrity of the data that powers them.
- What is data bias in AI? Data bias refers to the inaccuracies and unfairness that arise when AI systems are trained on unrepresentative or flawed datasets.
- How does data bias affect AI outcomes? Data bias can lead to skewed results, reinforcing existing inequalities and resulting in unfair treatment of certain groups.
- What can be done to mitigate data bias? Strategies include diversifying training datasets, improving data collection methods, and continuously monitoring AI outcomes for fairness.
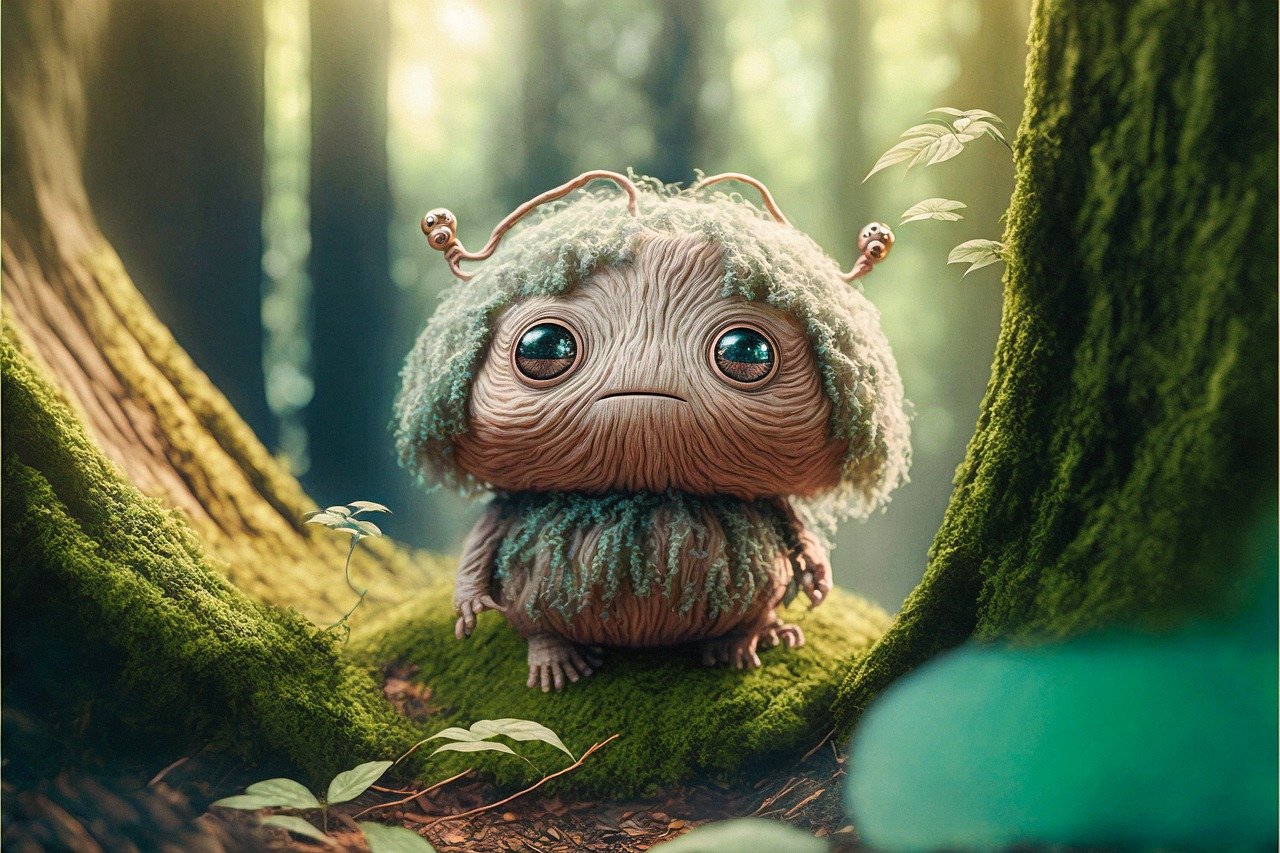
Algorithmic Bias
Algorithmic bias is a critical concern in the realm of artificial intelligence, referring to the systematic and unfair discrimination that can arise from the algorithms used in AI systems. This type of bias can manifest in various ways, often leading to outcomes that favor one group over another, thereby perpetuating existing inequalities. Imagine a scenario where an AI system is designed to screen job applicants. If the algorithm is trained on historical hiring data that reflects biased hiring practices, it may inadvertently favor candidates who resemble those previously hired, thereby disadvantaging equally qualified candidates from diverse backgrounds.
One of the fundamental reasons algorithmic bias occurs is due to the design and implementation processes that developers follow. Often, these algorithms are built on datasets that may not be fully representative of the population they are meant to serve. For instance, if an AI system is trained predominantly on data from a specific demographic, it may struggle to accurately assess or predict outcomes for individuals outside that demographic. This can lead to significant repercussions, particularly in sensitive areas such as healthcare, criminal justice, and hiring practices, where biased decisions can have life-altering effects.
Moreover, the lack of transparency in how algorithms operate can exacerbate the issue of algorithmic bias. Many AI systems function as "black boxes," making it challenging for users and stakeholders to understand how decisions are made. This opacity can lead to a lack of accountability, as it becomes difficult to pinpoint where biases originate and how they can be corrected. To illustrate the impact of algorithmic bias, consider the following table that outlines some common areas where this bias can occur:
Area of Impact | Example of Algorithmic Bias | Potential Consequences |
---|---|---|
Hiring | AI favors candidates from a specific demographic | Missed talent, lack of diversity |
Healthcare | Algorithms prioritize treatments based on biased data | Worsened health outcomes for underrepresented groups |
Criminal Justice | Predictive policing tools disproportionately target certain neighborhoods | Increased surveillance and mistrust in communities |
Addressing algorithmic bias requires a multifaceted approach. Developers and organizations must prioritize fairness in their AI systems by employing diverse datasets and continually testing algorithms for bias. Implementing regular audits and fostering a culture of transparency can also help mitigate the risks associated with algorithmic bias. Ultimately, as we advance further into the age of AI, it becomes increasingly vital to ensure that the algorithms we create are not only efficient but also ethical and just.
- What is algorithmic bias? Algorithmic bias refers to the unfair discrimination that can arise from the algorithms used in AI systems, often leading to outcomes that favor one group over another.
- How does algorithmic bias occur? It occurs due to non-representative training datasets, design choices, and the lack of transparency in algorithmic processes.
- What are the consequences of algorithmic bias? It can lead to significant repercussions in areas like hiring, healthcare, and criminal justice, perpetuating existing inequalities and causing harm to marginalized groups.
- How can we address algorithmic bias? By using diverse datasets, conducting regular audits, and promoting transparency in AI systems, we can work towards reducing algorithmic bias.
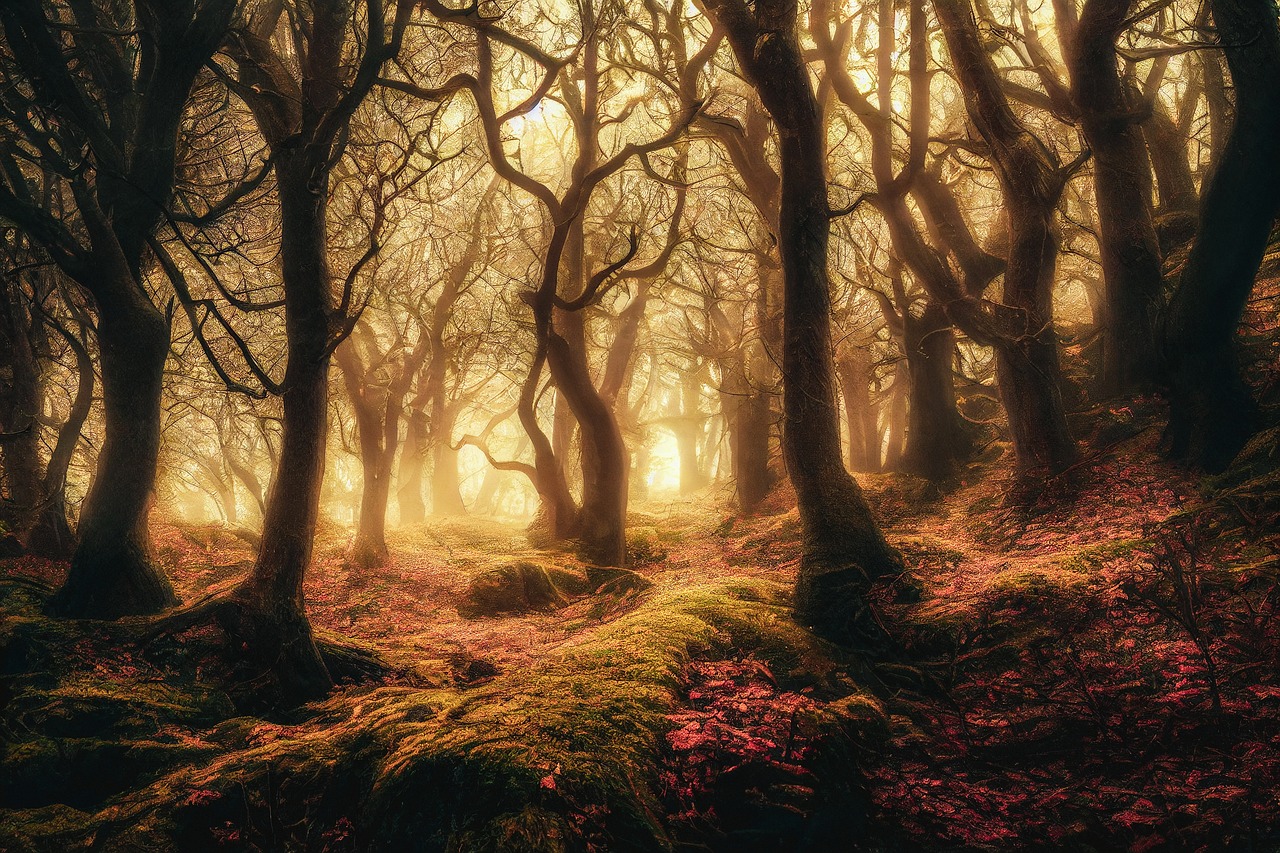
Addressing Bias in AI
Addressing bias in AI is not just an ethical obligation; it's a necessity for building trust and ensuring the technology serves all segments of society equitably. As we dive deeper into the world of artificial intelligence, it becomes increasingly clear that bias can seep into AI systems in numerous ways, from the data used to train models to the algorithms themselves. To tackle these biases effectively, several strategies can be employed.
One of the first steps in addressing bias is to diversify training data. By ensuring that the datasets used to train AI systems are representative of the diverse populations they will serve, we can significantly reduce the risk of biased outcomes. For instance, if an AI system is designed to recognize faces but is primarily trained on images of individuals from a single demographic, it may struggle to accurately identify faces from other backgrounds.
Furthermore, it's crucial to implement regular audits of AI systems. These audits can help identify and rectify biases that may have been overlooked during the initial development phase. By continuously monitoring AI performance and outcomes, organizations can make necessary adjustments and ensure that their systems remain fair and unbiased over time.
Another effective strategy involves the use of algorithmic fairness techniques. These techniques can help adjust the algorithms to account for biases. For example, techniques such as re-weighting or re-sampling can help balance the influence of different groups within the training data. Additionally, incorporating fairness constraints directly into the algorithm can help ensure that the system does not favor one group over another.
It's also essential to promote a culture of inclusivity and awareness among AI developers and stakeholders. Training teams to recognize their own biases and understand the implications of their work can lead to more conscientious AI development. When developers are aware of the potential for bias, they are more likely to take proactive steps to mitigate it in their work.
In conclusion, addressing bias in AI requires a multifaceted approach that combines diverse data, regular audits, algorithmic fairness techniques, and a culture of inclusivity. By embracing these strategies, we can pave the way for a more equitable future in AI, where technology truly serves everyone.
- What is bias in AI? Bias in AI refers to systematic errors in the outputs of AI systems that result from prejudiced assumptions in the machine learning process.
- How can we identify bias in AI systems? Bias can be identified through testing and auditing the AI systems with diverse datasets and analyzing the outcomes for discrepancies among different demographic groups.
- What are the consequences of biased AI? Biased AI can lead to unfair treatment of individuals, perpetuate stereotypes, and result in significant societal harm, especially in critical areas like hiring, law enforcement, and lending.
- Can AI be made completely unbiased? While it may not be possible to eliminate all biases entirely, implementing best practices can significantly reduce their impact and promote fairness.
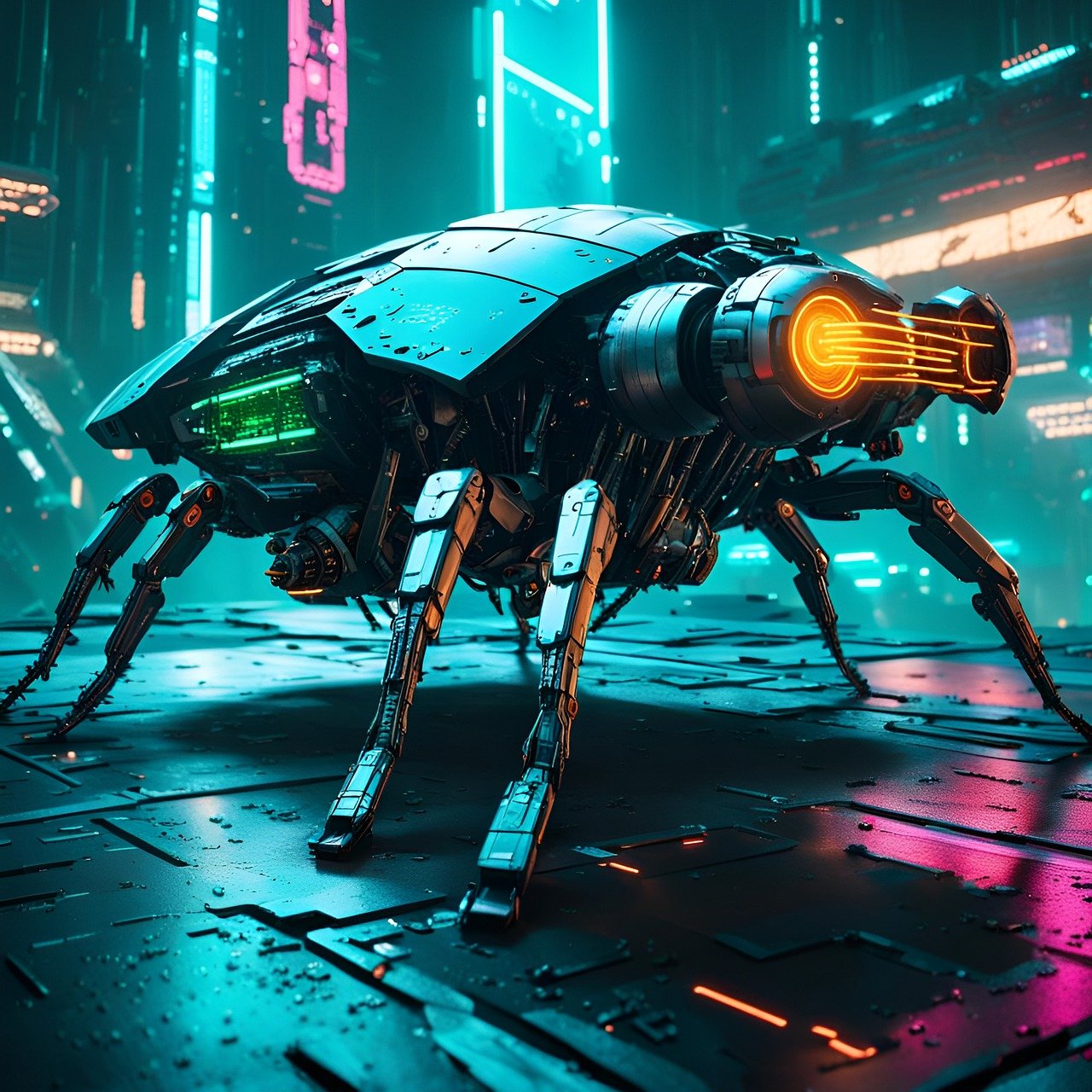
Accountability in AI Systems
Accountability is a cornerstone of ethical AI deployment. In a world increasingly driven by artificial intelligence, understanding who is responsible for the decisions made by these systems is crucial. As AI technologies become more complex, the lines of responsibility can blur, leading to questions about who should be held accountable when things go awry. Whether it’s a self-driving car making a split-second decision or a hiring algorithm that inadvertently discriminates, the need for clear accountability structures becomes paramount.
One of the primary challenges in establishing accountability in AI systems is the **lack of transparency**. Many AI models, especially those based on deep learning, operate as "black boxes," making it difficult to trace how decisions are made. This opacity can hinder our ability to assign responsibility when outcomes are harmful or unjust. To combat this, organizations must prioritize transparency in their AI systems. This involves not only making algorithms understandable but also documenting the decision-making processes and data sources used in AI development.
Moreover, the **responsibilities of developers and organizations** in ensuring ethical AI use cannot be overstated. Developers must adhere to ethical guidelines and best practices throughout the AI lifecycle, from design to deployment. Organizations, on the other hand, should implement policies that promote accountability at all levels. This might include regular audits of AI systems, establishing ethics boards, and providing training on ethical AI practices for employees. The following table illustrates some key responsibilities:
Responsibility | Description |
---|---|
Transparency | Ensuring AI decision-making processes are clear and understandable. |
Documentation | Keeping thorough records of data sources and algorithmic choices. |
Training | Providing staff with education on ethical AI practices and accountability. |
Regular Audits | Conducting periodic reviews of AI systems to identify and rectify issues. |
Establishing accountability frameworks is another essential step. These frameworks can help delineate responsibilities and ensure that AI systems are used ethically. They can take various forms, such as regulatory guidelines, internal policies, or industry standards, and should be tailored to the specific context in which the AI is deployed. By creating a structured approach to accountability, organizations can foster a culture of responsibility and ethical behavior.
Finally, the **legal implications of AI accountability** are evolving rapidly. As lawmakers grapple with the challenges posed by AI, new regulations are emerging that aim to hold developers and organizations accountable for their AI systems. This evolving legal landscape means that businesses must stay informed and adapt to new laws that may affect how they develop and deploy AI technologies. Understanding these regulations is vital not only for compliance but also for maintaining public trust in AI systems.
- What is accountability in AI systems? Accountability in AI systems refers to the responsibility of developers and organizations to ensure that AI technologies are used ethically and transparently.
- Why is transparency important in AI? Transparency is crucial because it allows stakeholders to understand how AI systems make decisions, which is essential for trust and accountability.
- What are some best practices for ensuring accountability in AI? Best practices include establishing clear accountability frameworks, conducting regular audits, and providing training on ethical AI practices.
- How are laws evolving around AI accountability? Laws are evolving to address the unique challenges posed by AI, with new regulations being introduced to hold developers and organizations accountable for their AI systems.
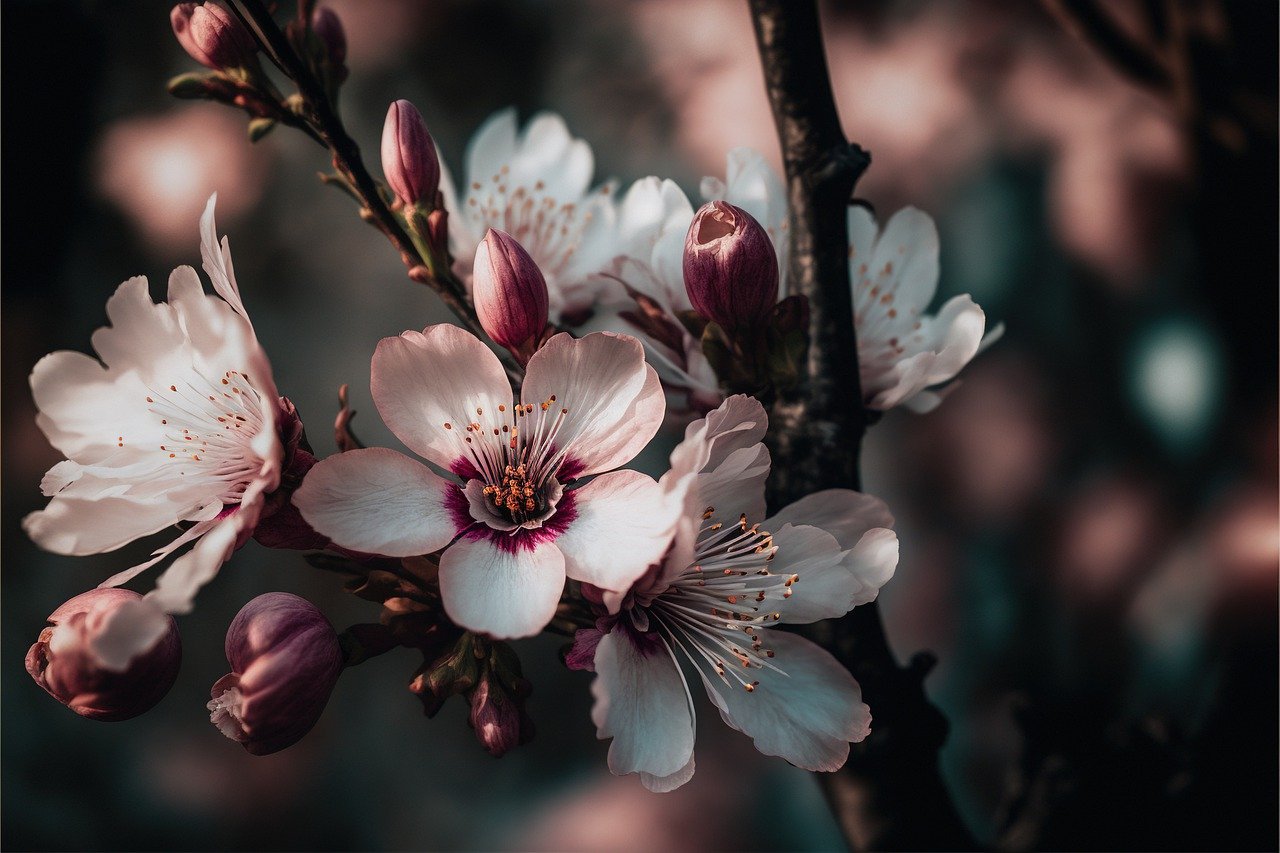
Establishing Accountability Frameworks
In the rapidly evolving landscape of artificial intelligence (AI), establishing accountability frameworks is not just a recommendation; it's a necessity. These frameworks serve as a guiding light, ensuring that all stakeholders involved in AI development and deployment understand their responsibilities. Think of it as a roadmap that outlines the ethical journey of AI technologies, helping to navigate the complex terrain of moral implications and societal impacts.
At the heart of these frameworks lies the principle of responsibility. Developers, organizations, and users must recognize that the decisions made during the design, development, and deployment of AI systems can have far-reaching consequences. As we dive deeper into this topic, it's crucial to understand that accountability is not just about assigning blame when things go wrong; it's about fostering a culture of ethical awareness and proactive governance.
One of the key components of an effective accountability framework is the establishment of clear roles and responsibilities. This involves not only identifying who is responsible for various aspects of the AI lifecycle but also ensuring that these roles are well-communicated across all levels of the organization. For instance, consider the following roles:
Role | Responsibilities |
---|---|
AI Developers | Design and implement algorithms with fairness and transparency in mind. |
Data Scientists | Ensure data integrity and representativeness in training datasets. |
Compliance Officers | Monitor adherence to ethical guidelines and legal regulations. |
End Users | Provide feedback and report any unethical outcomes or biases. |
These roles highlight the collaborative nature of accountability in AI. Each participant plays a vital part in ensuring that the technology is used ethically and responsibly. Furthermore, organizations should consider implementing regular training sessions to keep everyone informed about the latest ethical standards and best practices in AI. This not only enhances individual awareness but also cultivates a culture of accountability throughout the organization.
Another essential aspect of accountability frameworks is the establishment of feedback mechanisms. These mechanisms allow users and stakeholders to voice their concerns regarding AI systems. By creating channels for reporting issues—whether it's bias in AI outcomes or data privacy concerns—organizations can swiftly address problems before they escalate. Imagine a scenario where an AI-driven hiring tool inadvertently favors one demographic over another. If users can easily report this issue, the organization can take immediate action to rectify the bias, thus reinforcing their commitment to ethical AI.
Lastly, accountability frameworks must also include a component for transparency. This means that organizations should be open about their AI systems, including how they work and the data they use. Transparency builds trust among users and stakeholders, making them feel more comfortable interacting with AI technologies. By being upfront about their practices, organizations can foster a sense of collective responsibility, where everyone is invested in the ethical use of AI.
In conclusion, establishing accountability frameworks is not just about compliance; it's about embedding ethics into the very fabric of AI development and deployment. By clearly defining roles, implementing feedback mechanisms, and promoting transparency, organizations can create an environment where ethical AI thrives. In a world where technology is advancing at lightning speed, it’s our responsibility to ensure that our ethical standards keep pace.
- What are accountability frameworks in AI?
Accountability frameworks in AI are structured guidelines that define the roles, responsibilities, and ethical obligations of all stakeholders involved in the development and deployment of AI systems. - Why is accountability important in AI?
Accountability is crucial in AI to ensure that ethical standards are upheld, biases are minimized, and the technology is used responsibly, ultimately fostering trust among users and stakeholders. - How can organizations ensure accountability?
Organizations can ensure accountability by establishing clear roles, implementing feedback mechanisms, and promoting transparency in their AI systems. - What role do users play in AI accountability?
Users play a vital role by providing feedback, reporting issues, and actively participating in discussions about the ethical implications of AI technologies.
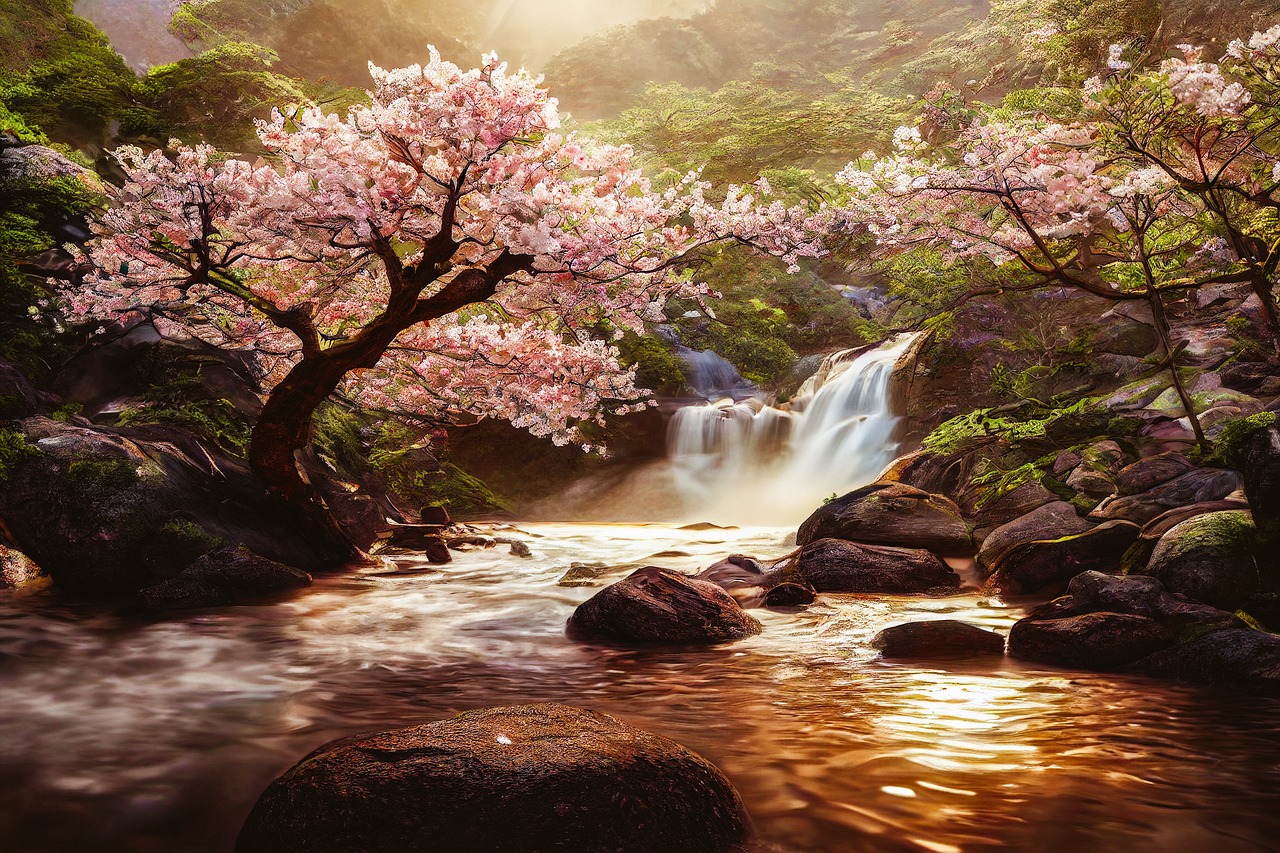
Legal Implications of AI Accountability
The rapid advancement of artificial intelligence (AI) technologies has opened up a Pandora's box of legal implications that society is only beginning to navigate. As AI systems become more integrated into various sectors, from healthcare to finance, the question of accountability becomes paramount. Who is responsible when an AI system makes a mistake? Is it the developers, the organizations that deploy these systems, or the AI itself? These questions are not just academic; they have real-world consequences that can affect individuals and businesses alike.
One of the primary legal concerns surrounding AI accountability is the liability for harm. If an AI system causes damage—whether physical, financial, or reputational—determining who is liable can be a complex issue. For instance, consider a scenario where an autonomous vehicle gets into an accident. Is the manufacturer liable for the software's failure, or is the owner responsible for the vehicle's operation? The legal frameworks currently in place are often ill-equipped to handle such nuances, leading to potential gaps in accountability.
Moreover, as AI technologies evolve, so too does the regulatory landscape. Governments worldwide are starting to recognize the need for laws that specifically address AI accountability. For example, the European Union has proposed regulations that aim to establish clear guidelines for AI use, emphasizing transparency and accountability. These regulations may require organizations to maintain detailed records of AI decision-making processes, ensuring that there is a trail that can be audited if necessary.
Another critical aspect of AI accountability is the ethical responsibility of developers and organizations. While legal accountability is essential, it is equally important for companies to adopt ethical frameworks that guide their AI practices. This includes implementing best practices for fairness, transparency, and user consent. Organizations that prioritize ethical AI are likely to build trust with their users, which can ultimately lead to greater success in the marketplace.
In light of these complexities, it becomes evident that a multifaceted approach is necessary to address the legal implications of AI accountability. This includes:
- Developing clear liability frameworks that delineate responsibility in AI-related incidents.
- Establishing regulatory guidelines that promote transparency and ethical use of AI technologies.
- Encouraging organizations to adopt internal accountability measures that prioritize ethical considerations in AI development.
As we move forward, it is crucial for stakeholders—be they developers, businesses, or policymakers—to engage in ongoing dialogue about the legal implications of AI accountability. By doing so, we can create a more robust framework that not only addresses current challenges but also anticipates future developments in the ever-evolving world of artificial intelligence.
- What is AI accountability? AI accountability refers to the responsibility of developers and organizations to ensure that AI systems operate ethically and transparently, and to be held liable for any adverse outcomes resulting from their use.
- Who is liable if an AI system causes harm? Liability can fall on various parties, including developers, organizations that deploy the AI, or even the users, depending on the circumstances and existing legal frameworks.
- Are there any regulations governing AI accountability? Yes, various governments and organizations are beginning to propose regulations aimed at establishing clear guidelines for AI use, particularly in the European Union.
- How can organizations ensure ethical AI use? Organizations can implement best practices for fairness, transparency, and user consent, and create internal accountability measures to guide their AI development.
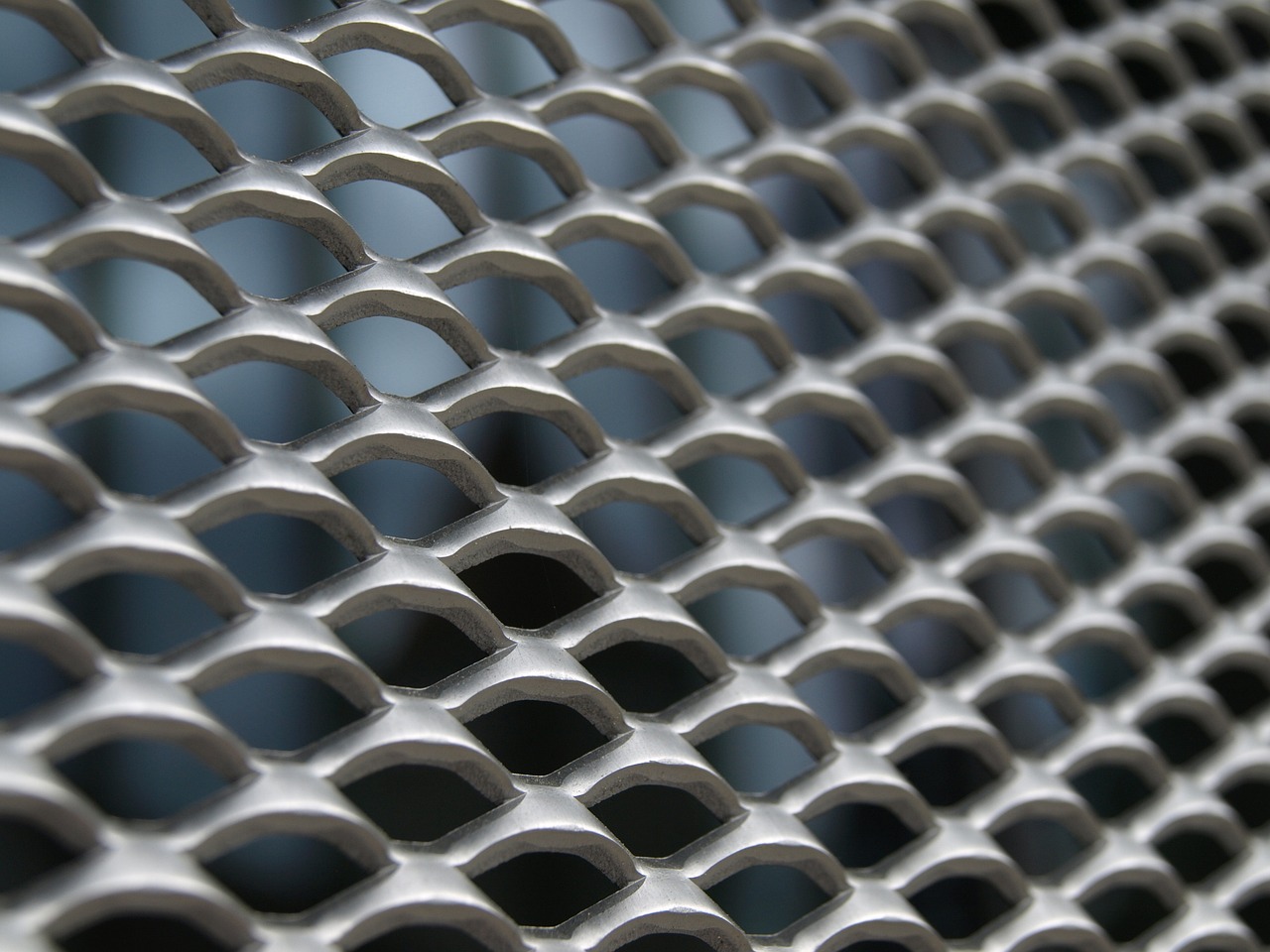
Privacy Concerns with AI
In today's digital age, where technology permeates every aspect of our lives, the adoption of artificial intelligence (AI) raises significant privacy concerns. As AI systems increasingly rely on vast amounts of data to learn and make decisions, the question of how this data is collected, stored, and used becomes crucial. Are we sacrificing our personal information on the altar of convenience? The answer is not straightforward, and it requires a closer examination of the processes involved in AI data handling.
At the heart of privacy issues in AI lies the concept of data collection practices. AI systems often gather data from various sources, including social media, online transactions, and even smart devices. This data collection can be extensive, leading to a detailed profile of individuals without their explicit consent. For instance, many users may not realize that their interactions with AI-driven applications are being monitored and analyzed to improve services, often without clear communication about how their data will be used.
Moreover, the lack of transparency in these data collection practices can create a sense of distrust among users. When individuals are unaware of what data is being collected, how it is being used, or who has access to it, they may feel that their privacy is compromised. This is where the importance of user consent and transparency comes into play. Companies deploying AI technologies must prioritize informing users about their data usage policies and obtaining explicit consent. Imagine walking into a store and finding out that every item you touch is being recorded without your knowledge; it's unsettling, right? This analogy highlights the need for clear communication in the digital realm as well.
To address these privacy concerns, many organizations are beginning to implement more robust data protection measures. These include anonymizing data to remove personally identifiable information and establishing clear guidelines for data retention. However, while these measures are steps in the right direction, they are not foolproof. The evolving landscape of AI technology means that privacy regulations must also keep pace. Governments and regulatory bodies are increasingly scrutinizing AI practices, aiming to create frameworks that protect user privacy while fostering innovation.
In summary, the intersection of AI and privacy is fraught with challenges that require careful consideration. As we embrace the benefits of AI technologies, we must also advocate for stronger privacy protections to ensure that our personal information remains secure. The conversation around privacy is not just about compliance; it's about building trust and ensuring that individuals have control over their own data in an AI-driven world.
- What are the main privacy concerns associated with AI?
Privacy concerns primarily revolve around data collection practices, user consent, and the potential misuse of personal information. - How can organizations ensure user privacy when using AI?
Organizations can implement robust data protection measures, maintain transparency about data usage, and obtain explicit user consent. - Are there regulations governing AI and privacy?
Yes, various regulations are being developed globally to address privacy concerns in AI, including GDPR in Europe and CCPA in California.
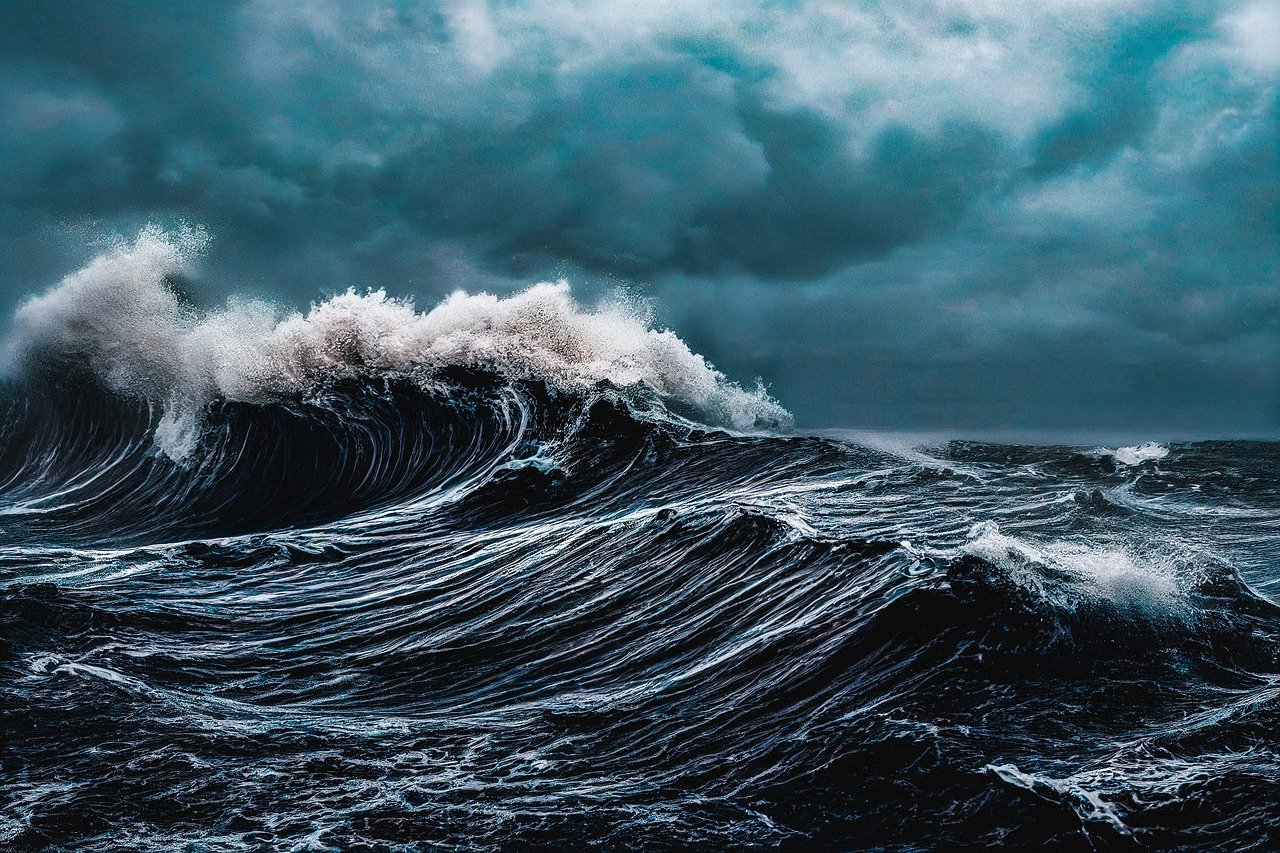
Data Collection Practices
In the realm of artificial intelligence, are pivotal. They form the backbone of any AI system, influencing everything from its performance to its ethical implications. But what does it mean to collect data responsibly? Well, it’s not just about gathering as much information as possible; it’s about ensuring that the data is accurate, relevant, and ethically sourced. Imagine trying to bake a cake with spoiled ingredients—no matter how good the recipe is, the result will be far from delightful. Similarly, poor data collection can lead to flawed AI systems that produce unreliable outcomes.
One of the primary concerns with data collection is consent. Users often unwittingly provide their information, sometimes without a clear understanding of how it will be used. Transparency is crucial here. Organizations should not only inform users about what data is being collected but also why it’s necessary. For instance, a social media platform might collect user data to improve user experience, but if users aren't aware of this, it raises ethical questions. Therefore, the practice of obtaining informed consent should be a fundamental part of any data collection strategy.
Moreover, data minimization is another vital principle. This concept revolves around collecting only the data that is absolutely necessary for the intended purpose. By adhering to this principle, organizations can significantly reduce the risk of data breaches and misuse. Think of it as only taking what you need from a buffet—if you pile your plate too high, not only do you risk wasting food, but you also increase the chance of spilling something. Similarly, excessive data collection can lead to complications and ethical dilemmas.
Another aspect to consider is the diversity of data sources. Relying on a narrow range of data can lead to skewed results. For instance, if an AI system is trained predominantly on data from a specific demographic, it may not perform well for users outside that group. This is akin to trying to teach a class of students using examples that only resonate with a few—most will be left confused. Thus, incorporating diverse data sources can enhance the fairness and effectiveness of AI systems.
Lastly, organizations must continuously evaluate their data collection practices. This involves not just looking at the data itself, but also the methods used to gather it. Regular audits can help identify potential ethical issues and biases that may have crept in over time. Just like maintaining a garden, where you must regularly check for weeds and pests, AI systems require ongoing attention to ensure they remain healthy and ethical.
Data Collection Principle | Description |
---|---|
Informed Consent | Users must be clearly informed about what data is collected and how it will be used. |
Data Minimization | Only collect data that is necessary for the intended purpose. |
Diversity of Data Sources | Use a wide range of data to avoid bias and ensure fairness. |
Regular Audits | Continuously evaluate data collection methods to identify and rectify ethical issues. |
- What is the importance of data collection in AI? Data collection is crucial as it determines the effectiveness and reliability of AI systems.
- How can organizations ensure ethical data collection? By obtaining informed consent, practicing data minimization, and ensuring diversity in data sources.
- What are the risks of poor data collection practices? Poor data collection can lead to biased outcomes, privacy violations, and a lack of trust from users.
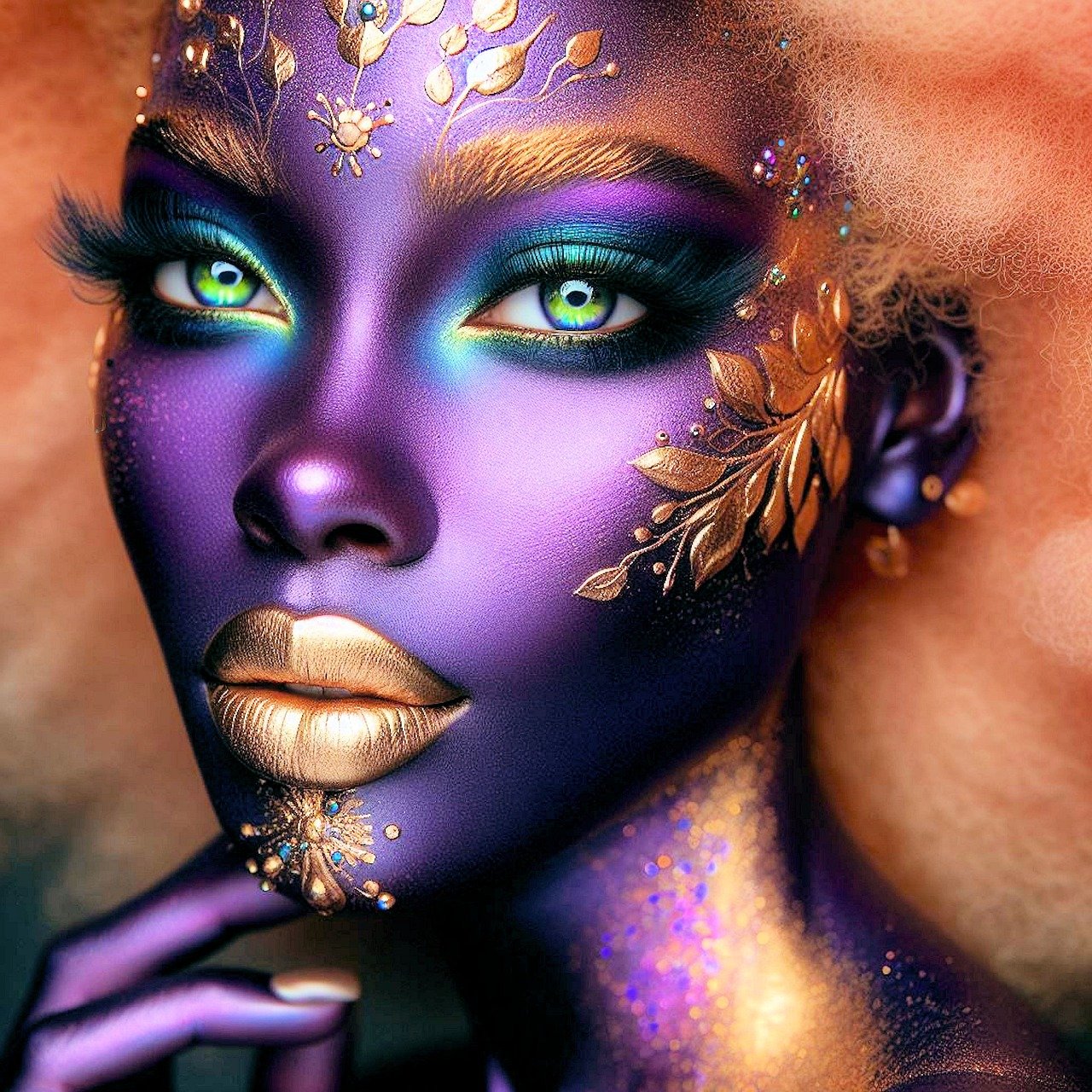
User Consent and Transparency
User consent and transparency are not just buzzwords in the realm of artificial intelligence; they are the bedrock of ethical AI practices. Imagine walking into a store where the owner knows everything about you—your preferences, your shopping habits, and even your personal history—without ever having asked for your permission. Sounds invasive, right? This analogy captures the essence of why user consent is crucial when it comes to AI technologies. Users should be fully aware of what data is being collected, how it is being used, and who it is being shared with. Transparency builds trust, and without it, users may feel exploited or manipulated.
When it comes to AI systems, the data collected can range from simple preferences to sensitive personal information. Therefore, it’s imperative for organizations to implement clear and accessible privacy policies. These policies should not be buried in legal jargon but rather articulated in a way that is easy for the average user to understand. For instance, companies can use straightforward language and visual aids to explain their data collection practices. A well-structured privacy policy might include:
- What data is collected? This can include user behavior, location data, and personal identifiers.
- How is the data used? Clarifying whether the data is used for improving services, targeted advertising, or research.
- Who has access to the data? Informing users if their data will be shared with third parties.
- How can users opt-out? Providing clear instructions on how users can withdraw consent or delete their data.
Additionally, organizations should implement mechanisms that allow users to easily give or withdraw consent. This could involve simple toggle switches in user settings or straightforward opt-in/opt-out options during the onboarding process. For example, a mobile app could prompt users to allow location tracking with a clear explanation of why this data is necessary for the app's functionality. By doing so, users feel empowered and informed, rather than coerced into surrendering their data.
Moreover, transparency is not just about informing users; it’s also about actively engaging them in conversations about their data. Companies can create feedback loops, where users can voice their concerns and suggestions regarding data use. This not only enhances user trust but also fosters a sense of community and shared responsibility. In this age of information, where data breaches and misuse are rampant, being transparent about data practices is more important than ever.
In conclusion, user consent and transparency are vital components of ethical AI adoption. Organizations must prioritize clear communication and user engagement to build trust and ensure that AI technologies serve the best interests of society. After all, in the world of AI, users are not just data points; they are individuals with rights and expectations.
- What is user consent in AI? User consent in AI refers to the process of obtaining permission from users before collecting, using, or sharing their personal data.
- Why is transparency important in AI? Transparency is crucial because it builds trust between users and organizations, ensuring that users are informed about how their data is being used.
- How can I ensure my data is safe when using AI? Always read privacy policies, look for transparency in data practices, and utilize opt-out options whenever available.
- What rights do I have regarding my data? Users typically have the right to know what data is collected, how it is used, and the ability to withdraw consent or request data deletion.
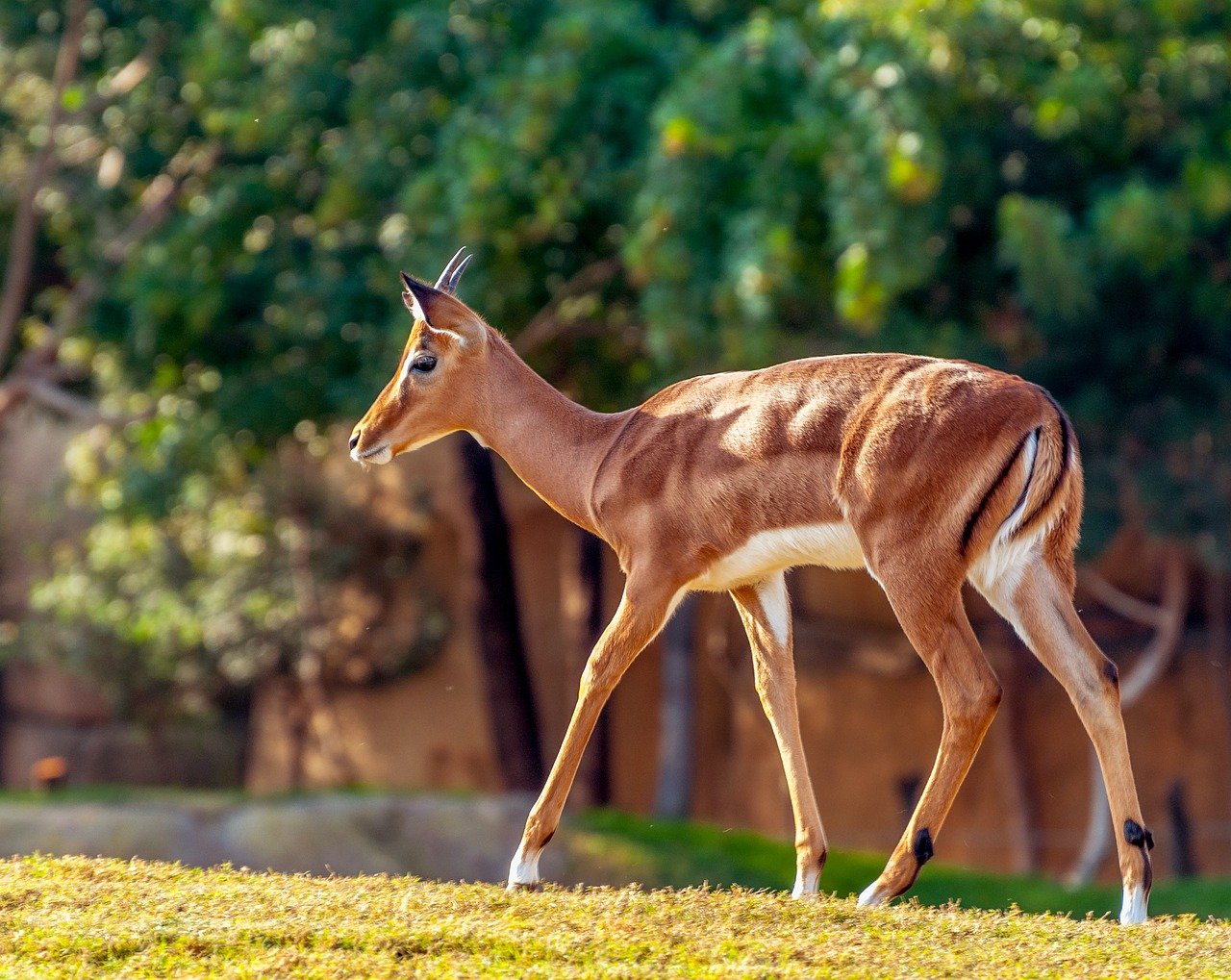
The Societal Impact of AI
Artificial Intelligence (AI) is not just a tech buzzword; it's a transformative force reshaping our world in ways we never imagined. As we stand on the brink of a new era, the societal impact of AI is becoming increasingly evident. From altering job markets to changing the way we communicate, AI technologies are penetrating every aspect of our lives. But what does this mean for us as individuals and as a society? Let's dive into the profound implications of AI adoption.
One of the most talked-about effects of AI is its potential to displace jobs. Automation and machine learning are revolutionizing industries, leading to increased efficiency and productivity. However, this progress comes at a cost. Many traditional jobs are at risk of being replaced by machines, leaving a significant portion of the workforce in uncertainty. For instance, roles in manufacturing, customer service, and even some professional services are increasingly being automated. The question arises: how do we prepare for a future where machines might take over tasks we once considered uniquely human?
Moreover, the economic landscape is shifting dramatically. With AI-driven tools enhancing productivity, businesses can operate at a scale and speed previously thought impossible. This shift may lead to greater economic inequality, where a small number of tech-savvy individuals or corporations reap the benefits, while others are left behind. It's crucial for policymakers to consider strategies that promote inclusive growth and ensure that the benefits of AI are shared across society.
On a social level, AI technologies are influencing our relationships and communication patterns. Social media algorithms, powered by AI, curate the content we see, shaping our perceptions and interactions. While this can foster connections, it can also lead to echo chambers where diverse viewpoints are drowned out. Furthermore, as AI systems become more integrated into our daily lives, we must ask ourselves: are we losing our ability to engage in meaningful, face-to-face conversations? The implications for mental health and community cohesion are profound.
To illustrate the societal impact of AI, consider the following table that outlines key areas affected by AI adoption:
Area | Impact |
---|---|
Employment | Job displacement and creation of new roles |
Economy | Increased productivity vs. economic inequality |
Social Interaction | Changes in communication and relationship dynamics |
Cultural Norms | Shifts in societal values and behaviors |
As we embrace these changes, it's essential to foster a dialogue about the ethical implications of AI. How can we ensure that AI serves humanity rather than undermines it? This conversation must include diverse voices, particularly those from marginalized communities who may be disproportionately affected by these technologies. By prioritizing inclusivity and ethical considerations, we can harness the potential of AI to create a better future for everyone.
- What are the main societal impacts of AI? AI impacts employment, economic inequality, social interactions, and cultural norms.
- How can we mitigate the negative effects of AI? By promoting inclusive policies and engaging diverse voices in discussions about AI ethics.
- Will AI create more jobs than it displaces? While AI may create new roles, the transition may not be smooth, and some jobs will inevitably be lost.
- How does AI affect communication? AI technologies can change how we interact, often leading to less face-to-face communication and more algorithm-driven interactions.

Job Displacement and Economic Changes
The rise of artificial intelligence (AI) has sparked a whirlwind of discussions about its potential to revolutionize industries and redefine the workforce. However, amidst the excitement, there's a looming concern: **job displacement**. As machines become smarter and more capable of performing tasks traditionally carried out by humans, many fear that their jobs could be at risk. But is it all doom and gloom? Not necessarily! While AI may lead to the automation of certain roles, it also opens the door to new opportunities and economic transformations.
First, let's consider the **impact on employment**. Studies show that AI can automate repetitive and mundane tasks, which might lead to the displacement of jobs in sectors like manufacturing and customer service. For instance, chatbots can handle customer inquiries, reducing the need for human operators. However, this doesn't mean that all jobs will vanish. Instead, the workforce may shift towards roles that require **creativity, emotional intelligence, and complex problem-solving**—skills that machines are still far from mastering.
Moreover, the integration of AI can lead to the **creation of new job categories**. According to a report by the World Economic Forum, while 75 million jobs may be displaced by 2022, **133 million new roles** could emerge that are more adapted to the new division of labor. This transition may require workers to reskill or upskill, emphasizing the importance of continuous education and training programs. Companies and governments need to collaborate to ensure that displaced workers have access to the necessary resources to adapt to the evolving job landscape.
Now, let's delve into the **economic changes** that AI adoption brings. The infusion of AI technologies into businesses can lead to increased productivity and efficiency. For example, AI can analyze vast amounts of data at lightning speed, providing insights that help companies make informed decisions. This boost in productivity can contribute to **economic growth** and potentially lead to lower prices for consumers. However, it can also exacerbate income inequality if the benefits of AI are not distributed equitably across society.
To illustrate the potential economic impact, consider the following table:
Sector | Potential Job Displacement | New Opportunities Created |
---|---|---|
Manufacturing | 500,000 | 150,000 (robot maintenance, programming) |
Customer Service | 300,000 | 100,000 (AI oversight, customer experience roles) |
Healthcare | 200,000 | 250,000 (AI-assisted roles, data analysis) |
As we can see, while some sectors may experience significant job losses, others will flourish with new roles that require a different skill set. This **transformation** is akin to the Industrial Revolution, where technological advancements led to the obsolescence of certain jobs but also paved the way for new industries and opportunities.
In conclusion, while the rise of AI presents challenges in terms of job displacement, it also offers a chance for economic evolution. The key lies in how we respond to these changes. By prioritizing education, reskilling, and equitable distribution of AI's benefits, we can navigate this transition effectively and harness the power of AI to create a brighter future for all.
- Will AI take away all jobs? Not all jobs will be eliminated; many will evolve, and new jobs will be created.
- What skills will be in demand in an AI-driven economy? Skills like creativity, emotional intelligence, and complex problem-solving will be increasingly valuable.
- How can workers prepare for changes brought by AI? Continuous education and training will be essential for adapting to the new job landscape.
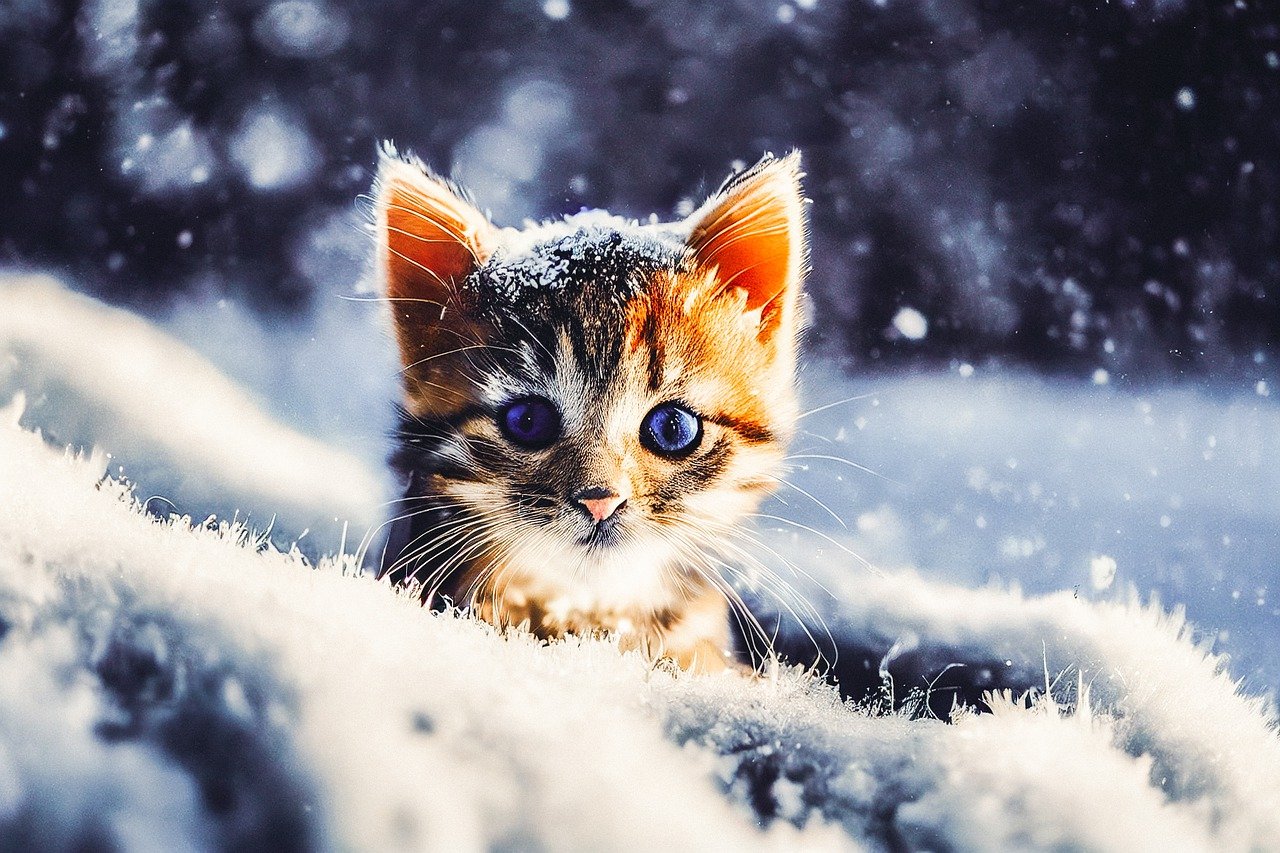
Social Implications of AI
The rise of artificial intelligence (AI) is reshaping the way we interact with the world, leading to significant social implications that cannot be overlooked. As AI technologies become more integrated into our daily lives, we find ourselves at a crossroads, where our relationships, communication styles, and even societal structures are being redefined. Imagine a world where machines not only assist us but also influence our decisions and interactions. This is not a far-off future; it is happening now.
One of the most profound impacts of AI is on communication. With the advent of AI-driven chatbots and virtual assistants, the way we engage with each other and with technology is evolving. While these tools can enhance productivity and provide instant responses, they can also create a sense of detachment. Have you ever noticed how a conversation with a chatbot feels different from talking to a person? The nuances of human interaction can sometimes be lost in translation, leading to a society that may become more isolated despite being more connected digitally.
Moreover, AI has the potential to influence relationships. Consider how dating apps use algorithms to match individuals based on preferences and behaviors. While this can lead to successful partnerships, it also raises questions about authenticity. Are we truly connecting with people, or are we simply responding to an algorithm? The reliance on AI in personal relationships can lead to a superficial understanding of compatibility, where the depth of human emotion is overshadowed by data-driven decisions.
Furthermore, the societal structure itself is being impacted by AI technologies. For instance, the way we consume information has drastically changed. Traditional media outlets are being challenged by AI-generated content, which can spread information faster than ever before. This shift raises concerns about the quality of information and the potential for misinformation. As AI systems curate news feeds based on our preferences, we risk creating echo chambers that reinforce our existing beliefs rather than challenge them. The implications of this are significant, as it can lead to polarization and a fragmented society.
To illustrate these points, let’s consider a few key areas where AI is making its mark:
Area | Implication |
---|---|
Communication | Increased reliance on digital interactions may reduce face-to-face communication skills. |
Relationships | AI-driven matchmaking can lead to superficial connections. |
Information Consumption | AI can create echo chambers, limiting exposure to diverse viewpoints. |
As we navigate this new landscape, it is crucial to foster a critical awareness of how AI influences our lives. Encouraging open dialogues about the role of AI in society can help mitigate its negative effects. We must ask ourselves: How do we want to shape our future with AI? The answers to these questions will guide us in creating a society that leverages AI for the betterment of all, rather than allowing it to dictate our interactions and relationships.
- What are the main social implications of AI? AI impacts communication, relationships, and information consumption, leading to potential isolation and superficial connections.
- How can AI influence our relationships? AI-driven tools can enhance matchmaking but may lead to less authentic connections.
- What is the risk of AI in information consumption? AI can create echo chambers, limiting exposure to diverse perspectives and fostering polarization.
Frequently Asked Questions
- What are the main ethical considerations in AI adoption?
When it comes to AI adoption, the primary ethical considerations include fairness, accountability, privacy, and the overall societal impact of AI technologies. These elements are crucial in ensuring that AI systems are developed and deployed in a way that respects human rights and promotes social good.
- How can bias be introduced in AI systems?
Bias can creep into AI systems through various avenues, primarily through data bias, algorithmic bias, and human bias. Data bias occurs when the training datasets are flawed or unrepresentative, while algorithmic bias stems from the algorithms favoring certain outcomes. Human bias can influence both data collection and algorithm design, leading to skewed results.
- What strategies can be implemented to address bias in AI?
To tackle bias in AI, organizations can adopt several strategies, such as using diverse datasets, implementing fairness-aware algorithms, and regularly auditing AI systems for bias. Engaging multidisciplinary teams during the development process can also help in identifying and mitigating potential biases.
- Why is accountability important in AI systems?
Accountability is vital in AI systems because it ensures that developers and organizations take responsibility for the outcomes of their technologies. Establishing clear accountability frameworks helps delineate roles and responsibilities, making it easier to address any ethical concerns that arise during AI deployment.
- What are the legal implications of AI accountability?
The legal landscape surrounding AI accountability is rapidly evolving. Current laws may not fully address the unique challenges posed by AI technologies, leading to potential gaps in regulation. As AI continues to grow, new regulations are likely to emerge, holding developers and organizations accountable for ethical AI use.
- How do AI technologies impact personal privacy?
AI technologies can significantly impact personal privacy by collecting and analyzing vast amounts of personal data. This raises concerns about how data is used, stored, and shared, making it essential for organizations to prioritize data protection and ensure that users' privacy is respected.
- What role does user consent play in AI ethics?
User consent is a cornerstone of ethical AI practices. It is crucial for organizations to inform users about how their data will be used and to obtain their explicit consent. Transparency in data collection practices fosters trust and empowers users to make informed decisions.
- What are the societal implications of AI adoption?
The societal implications of AI are profound, affecting everything from job displacement to social dynamics. As AI technologies automate tasks, there are concerns about job loss and economic changes. Additionally, AI can influence relationships and communication, reshaping how society interacts.
- How does AI contribute to job displacement?
AI contributes to job displacement primarily through automation, where machines take over tasks traditionally performed by humans. This can lead to significant shifts in the job market, requiring workers to adapt to new roles and develop new skills to remain relevant.
- Can AI technologies influence social dynamics?
Absolutely! AI technologies can alter social dynamics by changing how we communicate, interact, and build relationships. For instance, AI-driven platforms can enhance connectivity but may also lead to decreased face-to-face interactions, impacting the fabric of society.